Improving Low Compute Language Modeling with In-Domain Embedding Initialisation
Charles Welch, Rada Mihalcea, Jonathan K. Kummerfeld
Interpretability and Analysis of Models for NLP Short Paper
You can open the pre-recorded video in a separate window.
Abstract:
Many NLP applications, such as biomedical data and technical support, have 10-100 million tokens of in-domain data and limited computational resources for learning from it. How should we train a language model in this scenario? Most language modeling research considers either a small dataset with a closed vocabulary (like the standard 1 million token Penn Treebank), or the whole web with byte-pair encoding. We show that for our target setting in English, initialising and freezing input embeddings using in-domain data can improve language model performance by providing a useful representation of rare words, and this pattern holds across several different domains. In the process, we show that the standard convention of tying input and output embeddings does not improve perplexity when initializing with embeddings trained on in-domain data.
NOTE: Video may display a random order of authors.
Correct author list is at the top of this page.
Connected Papers in EMNLP2020
Similar Papers
An Empirical Investigation Towards Efficient Multi-Domain Language Model Pre-training
Kristjan Arumae, Qing Sun, Parminder Bhatia,
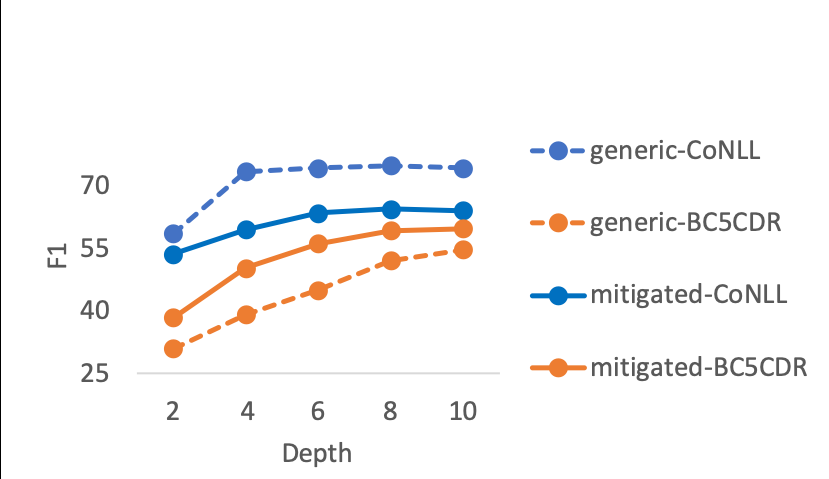
On the importance of pre-training data volume for compact language models
Vincent Micheli, Martin d'Hoffschmidt, François Fleuret,

Multi-Stage Pre-training for Low-Resource Domain Adaptation
Rong Zhang, Revanth Gangi Reddy, Md Arafat Sultan, Vittorio Castelli, Anthony Ferritto, Radu Florian, Efsun Sarioglu Kayi, Salim Roukos, Avi Sil, Todd Ward,

Data and Representation for Turkish Natural Language Inference
Emrah Budur, Rıza Özçelik, Tunga Gungor, Christopher Potts,
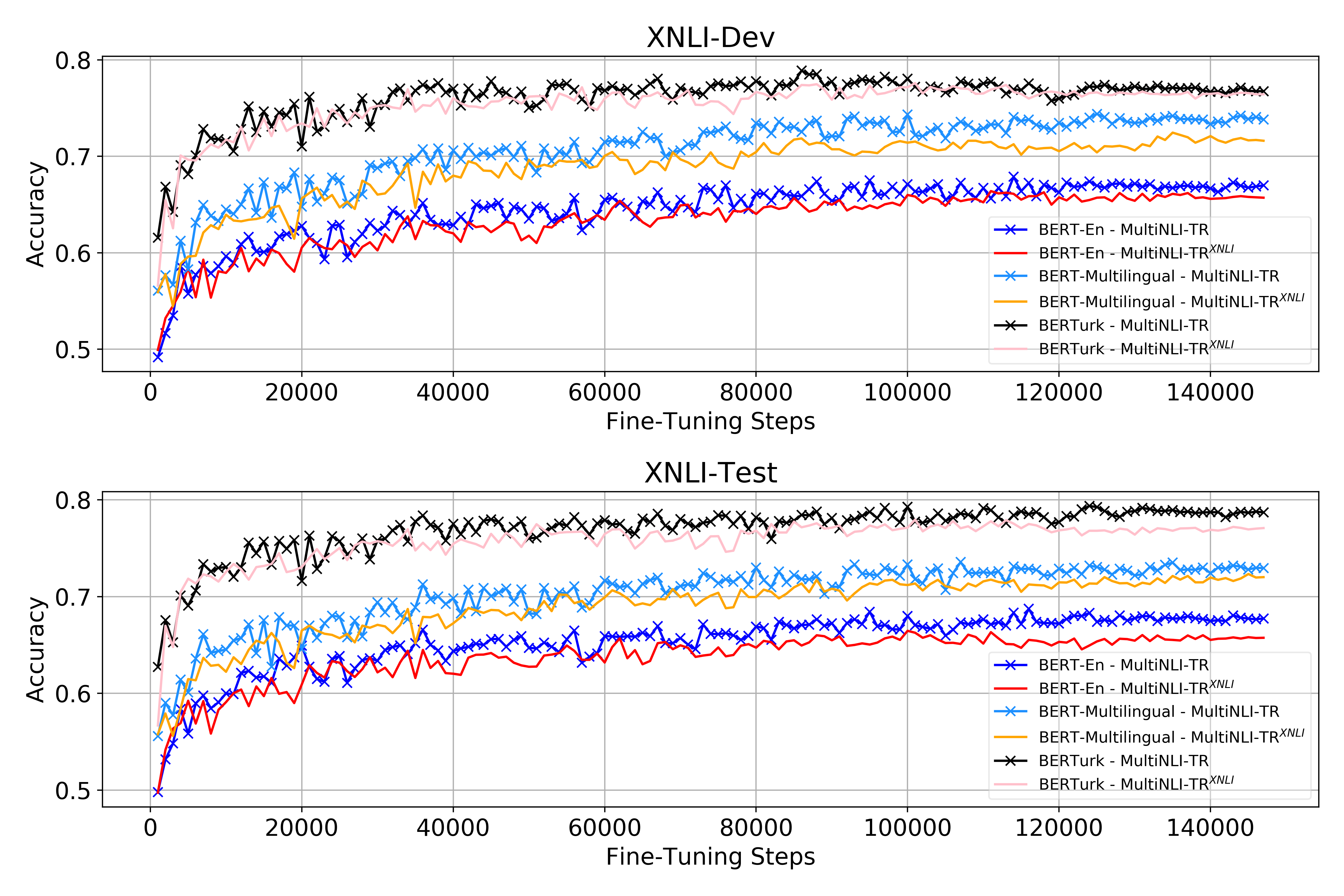