Uncertainty-Aware Label Refinement for Sequence Labeling
Tao Gui, Jiacheng Ye, Qi Zhang, Zhengyan Li, Zichu Fei, Yeyun Gong, Xuanjing Huang
Syntax: Tagging, Chunking, and Parsing Long Paper
You can open the pre-recorded video in a separate window.
Abstract:
Conditional random fields (CRF) for label decoding has become ubiquitous in sequence labeling tasks. However, the local label dependencies and inefficient Viterbi decoding have always been a problem to be solved. In this work, we introduce a novel two-stage label decoding framework to model long-term label dependencies, while being much more computationally efficient. A base model first predicts draft labels, and then a novel two-stream self-attention model makes refinements on these draft predictions based on long-range label dependencies, which can achieve parallel decoding for a faster prediction. In addition, in order to mitigate the side effects of incorrect draft labels, Bayesian neural networks are used to indicate the labels with a high probability of being wrong, which can greatly assist in preventing error propagation. The experimental results on three sequence labeling benchmarks demonstrated that the proposed method not only outperformed the CRF-based methods but also greatly accelerated the inference process.
NOTE: Video may display a random order of authors.
Correct author list is at the top of this page.
Connected Papers in EMNLP2020
Similar Papers
An Exploration of Arbitrary-Order Sequence Labeling via Energy-Based Inference Networks
Lifu Tu, Tianyu Liu, Kevin Gimpel,
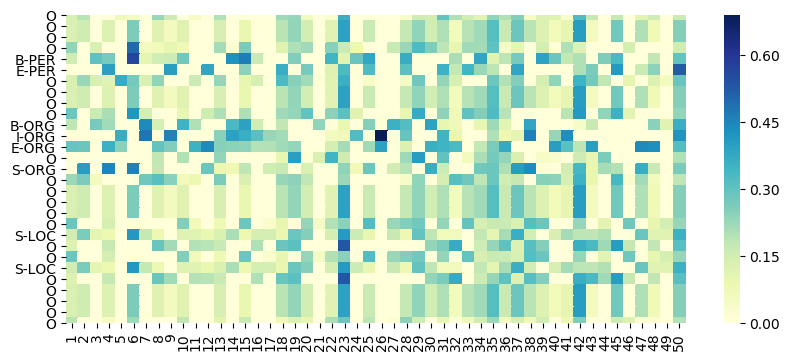
Semantic Label Smoothing for Sequence to Sequence Problems
Michal Lukasik, Himanshu Jain, Aditya Menon, Seungyeon Kim, Srinadh Bhojanapalli, Felix Yu, Sanjiv Kumar,

Augmented Natural Language for Generative Sequence Labeling
Ben Athiwaratkun, Cicero Nogueira dos Santos, Jason Krone, Bing Xiang,
