Self-Induced Curriculum Learning in Self-Supervised Neural Machine Translation
Dana Ruiter, Josef van Genabith, Cristina España-Bonet
Machine Translation and Multilinguality Long Paper
You can open the pre-recorded video in a separate window.
Abstract:
Self-supervised neural machine translation (SSNMT) jointly learns to identify and select suitable training data from comparable (rather than parallel) corpora and to translate, in a way that the two tasks support each other in a virtuous circle. In this study, we provide an in-depth analysis of the sampling choices the SSNMT model makes during training. We show how, without it having been told to do so, the model self-selects samples of increasing (i) complexity and (ii) task-relevance in combination with (iii) performing a denoising curriculum. We observe that the dynamics of the mutual-supervision signals of both system internal representation types are vital for the extraction and translation performance. We show that in terms of the Gunning-Fog Readability index, SSNMT starts extracting and learning from Wikipedia data suitable for high school students and quickly moves towards content suitable for first year undergraduate students.
NOTE: Video may display a random order of authors.
Correct author list is at the top of this page.
Connected Papers in EMNLP2020
Similar Papers
Multilingual Denoising Pre-training for Neural Machine Translation
Jiatao Gu, Yinhan Liu, Naman Goyal, Xian Li, Sergey Edunov, Marjan Ghazvininejad, Mike Lewis, Luke Zettlemoyer,

Towards Enhancing Faithfulness for Neural Machine Translation
Rongxiang Weng, Heng Yu, Xiangpeng Wei, Weihua Luo,
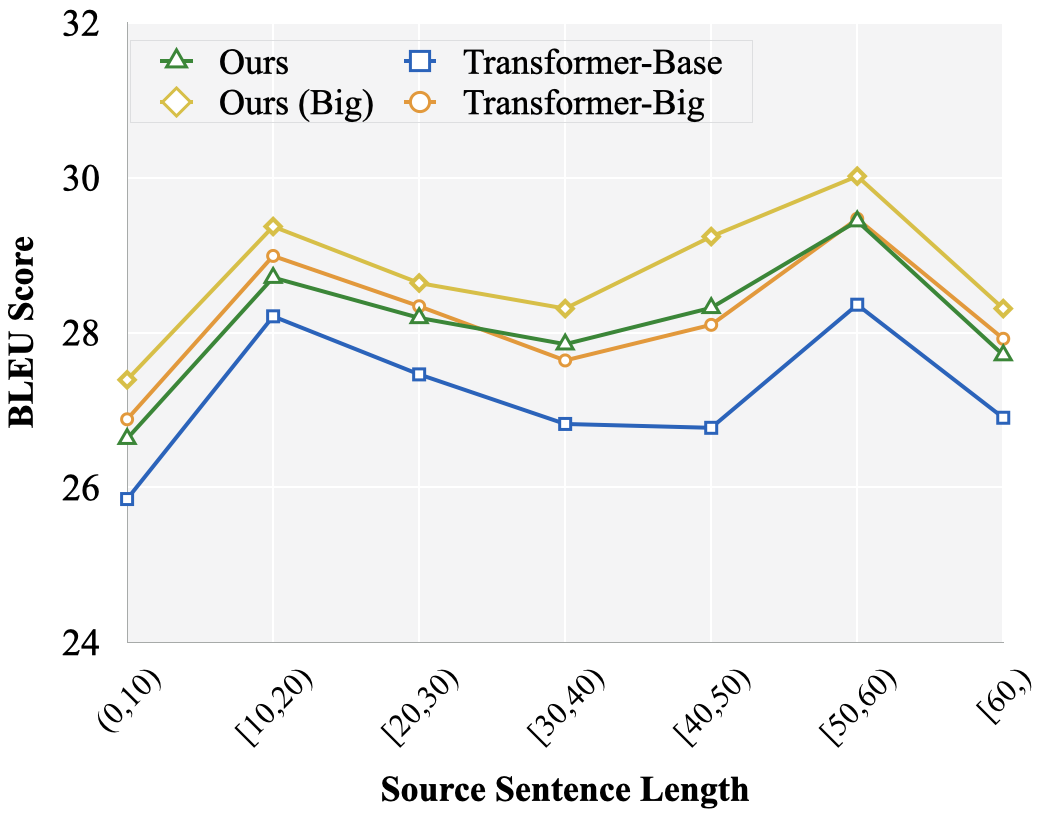
Shallow-to-Deep Training for Neural Machine Translation
Bei Li, Ziyang Wang, Hui Liu, Yufan Jiang, Quan Du, Tong Xiao, Huizhen Wang, Jingbo Zhu,

Learning Which Features Matter: RoBERTa Acquires a Preference for Linguistic Generalizations (Eventually)
Alex Warstadt, Yian Zhang, Xiaocheng Li, Haokun Liu, Samuel R. Bowman,
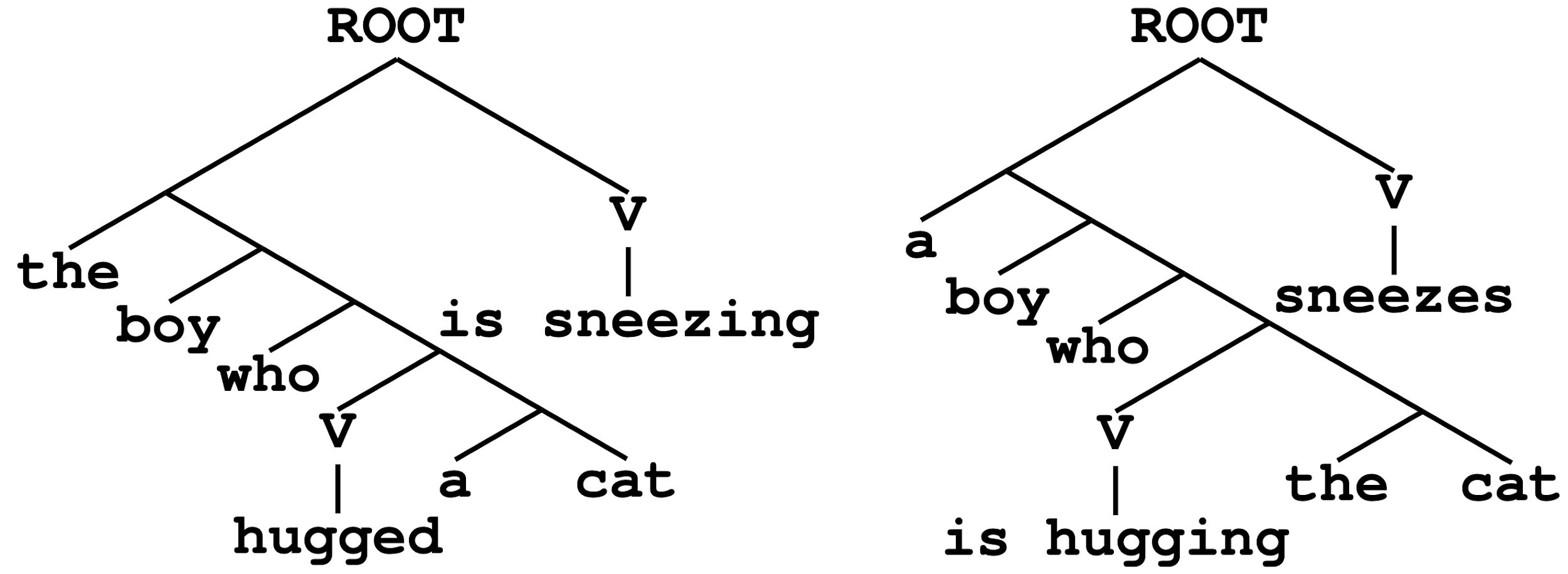