GraphDialog: Integrating Graph Knowledge into End-to-End Task-Oriented Dialogue Systems
Shiquan Yang, Rui Zhang, Sarah Erfani
Dialog and Interactive Systems Long Paper
You can open the pre-recorded video in a separate window.
Abstract:
End-to-end task-oriented dialogue systems aim to generate system responses directly from plain text inputs. There are two challenges for such systems: one is how to effectively incorporate external knowledge bases (KBs) into the learning framework; the other is how to accurately capture the semantics of dialogue history. In this paper, we address these two challenges by exploiting the graph structural information in the knowledge base and in the dependency parsing tree of the dialogue. To effectively leverage the structural information in dialogue history, we propose a new recurrent cell architecture which allows representation learning on graphs. To exploit the relations between entities in KBs, the model combines multi-hop reasoning ability based on the graph structure. Experimental results show that the proposed model achieves consistent improvement over state-of-the-art models on two different task-oriented dialogue datasets.
NOTE: Video may display a random order of authors.
Correct author list is at the top of this page.
Connected Papers in EMNLP2020
Similar Papers
AttnIO: Knowledge Graph Exploration with In-and-Out Attention Flow for Knowledge-Grounded Dialogue
Jaehun Jung, Bokyung Son, Sungwon Lyu,
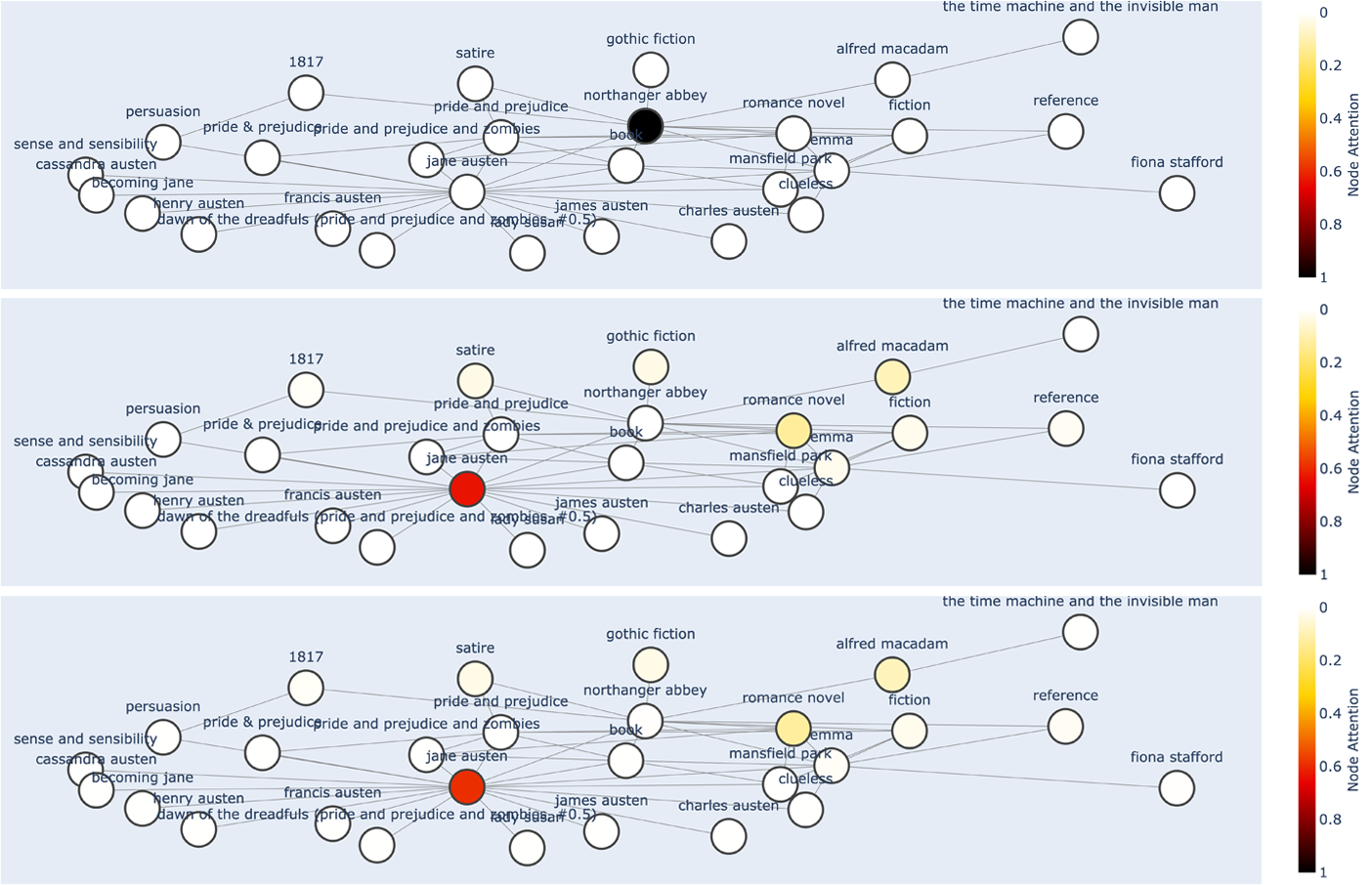
Multi-turn Response Selection using Dialogue Dependency Relations
Qi Jia, Yizhu Liu, Siyu Ren, Kenny Zhu, Haifeng Tang,

doc2dial: A Goal-Oriented Document-Grounded Dialogue Dataset
Song Feng, Hui Wan, Chulaka Gunasekara, Siva Patel, Sachindra Joshi, Luis Lastras,
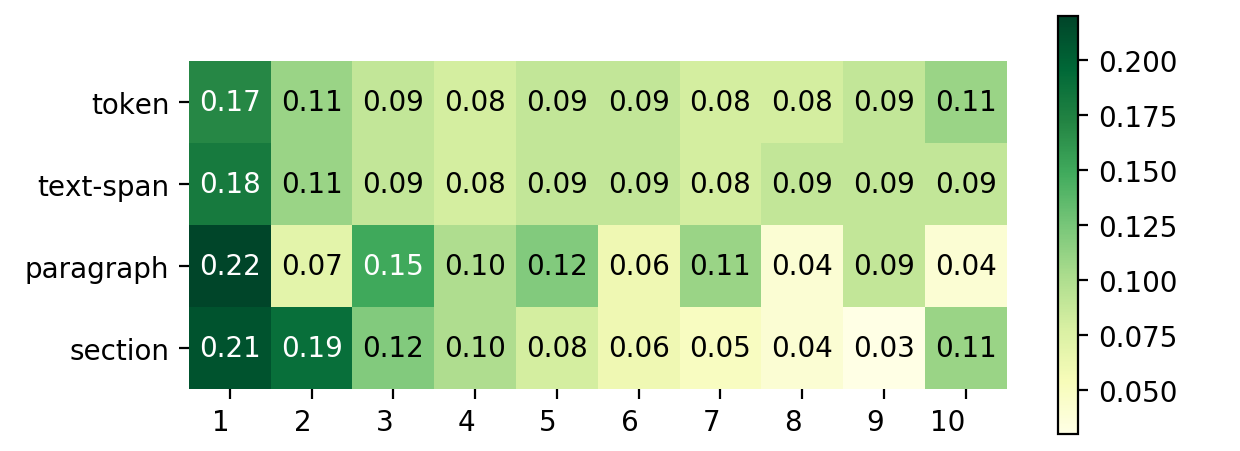
MinTL: Minimalist Transfer Learning for Task-Oriented Dialogue Systems
Zhaojiang Lin, Andrea Madotto, Genta Indra Winata, Pascale Fung,
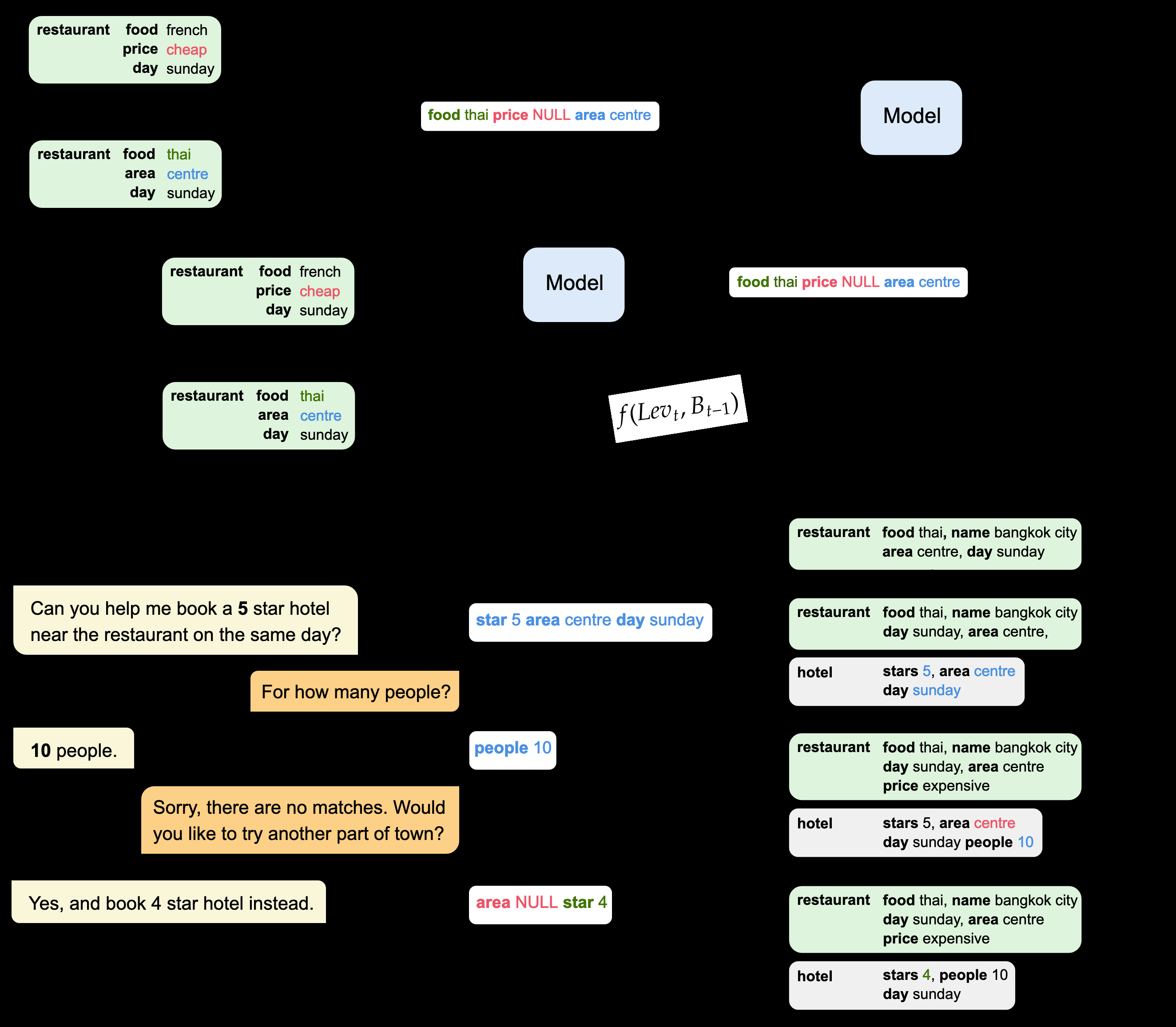