A Neural Generative Model for Joint Learning Topics and Topic-Specific Word Embeddings
Lixing Zhu, Deyu Zhou, Yulan He
Machine Learning for NLP Tacl Paper
You can open the pre-recorded video in a separate window.
Abstract:
We propose a novel generative model to explore both local and global context for joint learning topics and topic-specific word embeddings. In particular, we assume that global latent topics are shared across documents; a word is generated by a hidden semantic vector encoding its contextual semantic meaning; and its context words are generated conditional on both the hidden semantic vector and global latent topics. Topics are trained jointly with the word embeddings. The trained model maps words to topic-dependent embeddings, which naturally addresses the issue of word polysemy. Experimental results show that the proposed model outperforms the word-level embedding methods in both word similarity evaluation and word sense disambiguation. Furthermore, the model also extracts more coherent topics compared to existing neural topic models or other models for joint learning of topics and word embeddings. Finally, the model can be easily integrated with existing deep contextualized word embedding learning methods to further improve the performance of downstream tasks such as sentiment classification.
NOTE: Video may display a random order of authors.
Correct author list is at the top of this page.
Connected Papers in EMNLP2020
Similar Papers
Short Text Topic Modeling with Topic Distribution Quantization and Negative Sampling Decoder
Xiaobao Wu, Chunping Li, Yan Zhu, Yishu Miao,

Tired of Topic Models? Clusters of Pretrained Word Embeddings Make for Fast and Good Topics too!
Suzanna Sia, Ayush Dalmia, Sabrina J. Mielke,
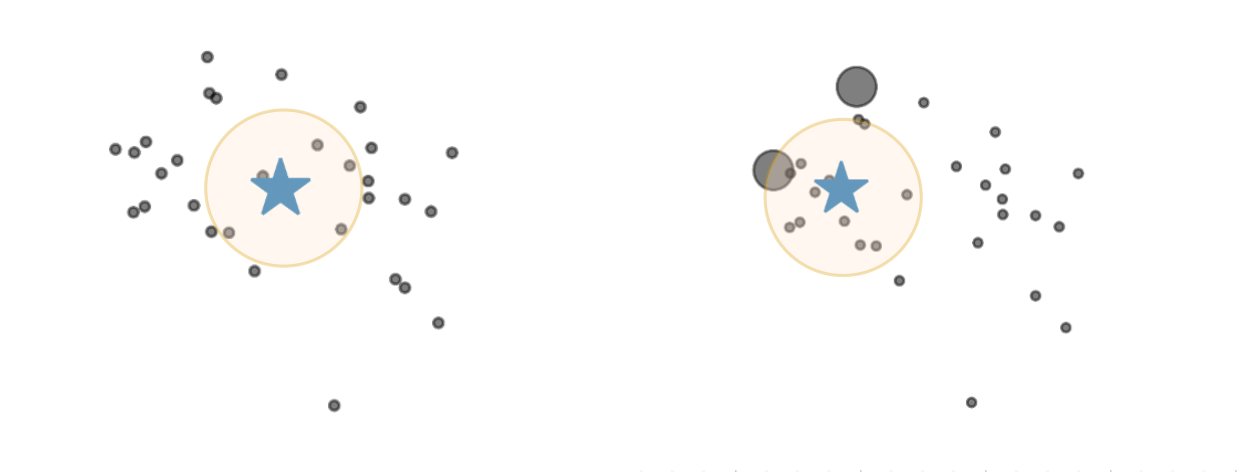
Methods for Numeracy-Preserving Word Embeddings
Dhanasekar Sundararaman, Shijing Si, Vivek Subramanian, Guoyin Wang, Devamanyu Hazarika, Lawrence Carin,
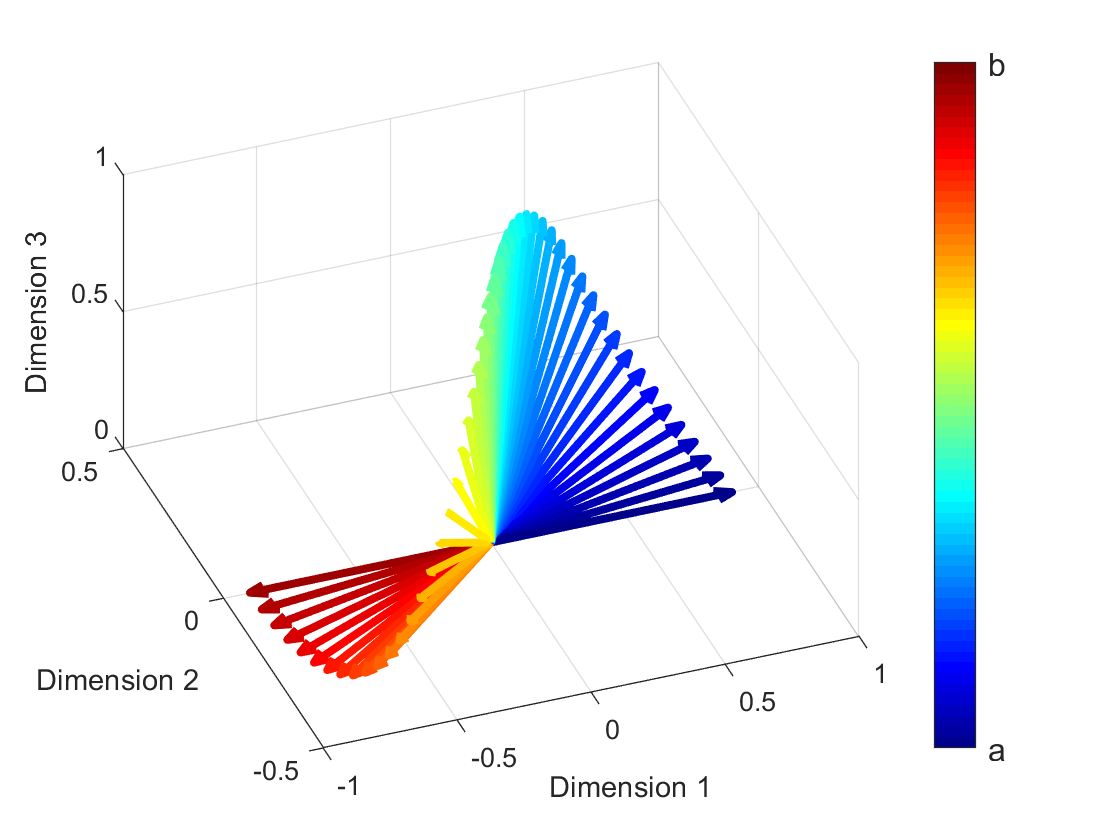
A Bilingual Generative Transformer for Semantic Sentence Embedding
John Wieting, Graham Neubig, Taylor Berg-Kirkpatrick,
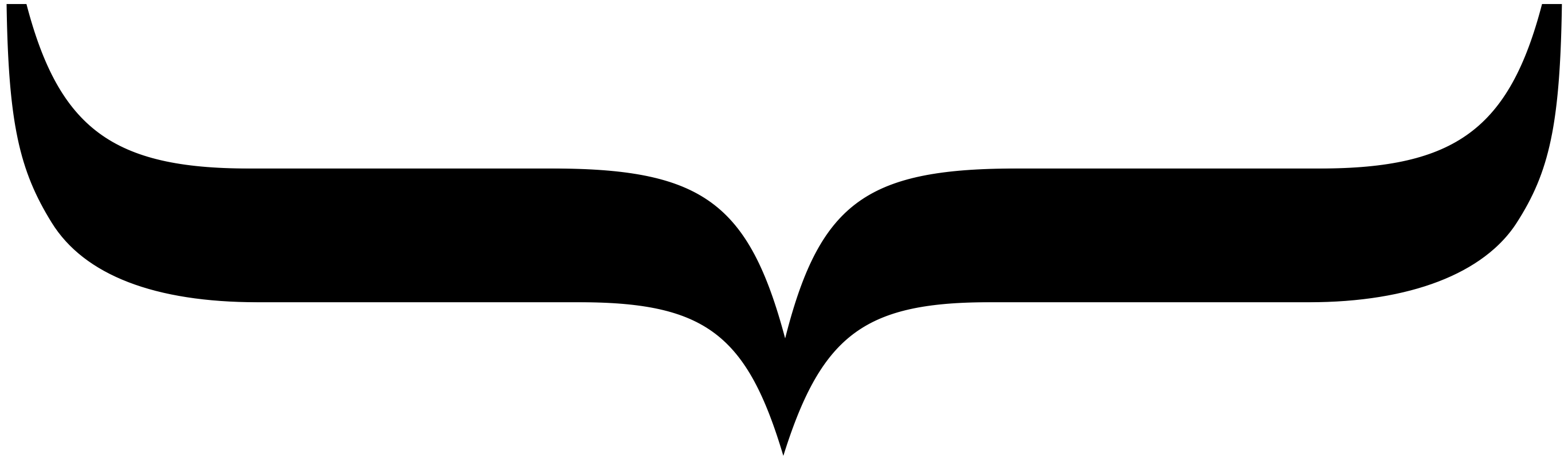