An Empirical Study on Robustness to Spurious Correlations using Pre-trained Language Models
Lifu Tu, Garima Lalwani, Spandana Gella, He He
Semantics: Sentence-level Semantics, Textual Inference and Other areas Tacl Paper
You can open the pre-recorded video in a separate window.
Abstract:
Recent work has shown that pre-trained language models such as BERT improve robustness to spurious correlations in the dataset. Intrigued by these results, we find that the key to their success is generalization from a small amount of counterexamples where the spurious correlations do not hold. When such minority examples are scarce, pre-trained models perform as poorly as models trained from scratch. In the case of extreme minority, we propose to use multi-task learning (MTL) to improve generalization. Our experiments on natural language inference and paraphrase identification show that MTL with the right auxiliary tasks significantly improves performance on challenging examples without hurting the in-distribution performance. Further, we show that the gain from MTL mainly comes from improved generalization from the minority examples. Our results highlight the importance of data diversity for overcoming spurious correlations.
NOTE: Video may display a random order of authors.
Correct author list is at the top of this page.
Connected Papers in EMNLP2020
Similar Papers
oLMpics - On what Language Model Pre-training Captures
Alon Talmor, Yanai Elazar, Yoav Goldberg, Jonathan Berant,

Data Weighted Training Strategies for Grammatical Error Correction
Jared Lichtarge, Chris Alberti, Shankar Kumar,

An Empirical Investigation Towards Efficient Multi-Domain Language Model Pre-training
Kristjan Arumae, Qing Sun, Parminder Bhatia,
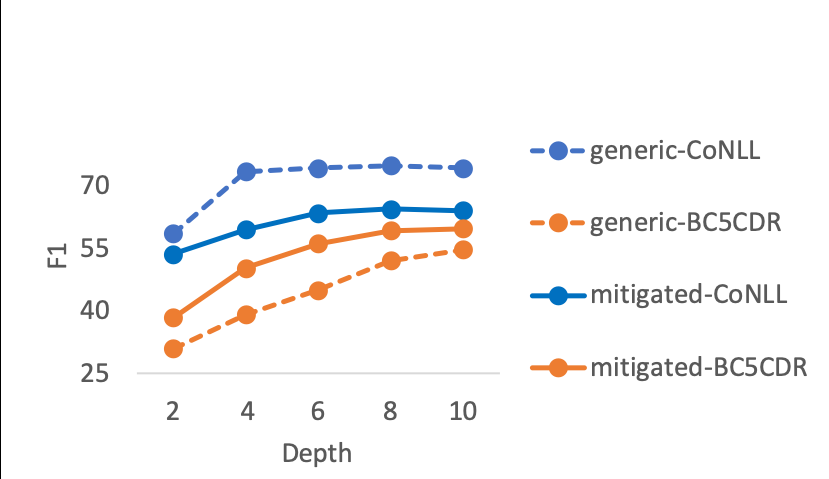
Learning to Contrast the Counterfactual Samples for Robust Visual Question Answering
Zujie Liang, Weitao Jiang, Haifeng Hu, Jiaying Zhu,
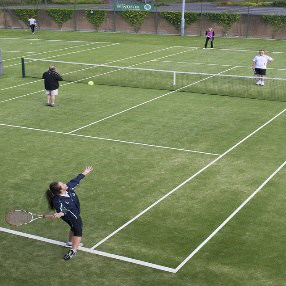