TeaForN: Teacher-Forcing with N-grams
Sebastian Goodman, Nan Ding, Radu Soricut
Language Generation Long Paper
You can open the pre-recorded video in a separate window.
Abstract:
Sequence generation models trained with teacher-forcing suffer from issues related to exposure bias and lack of differentiability across timesteps. Our proposed method, Teacher-Forcing with N-grams (TeaForN), addresses both these problems directly, through the use of a stack of N decoders trained to decode along a secondary time axis that allows model-parameter updates based on N prediction steps. TeaForN can be used with a wide class of decoder architectures and requires minimal modifications from a standard teacher-forcing setup. Empirically, we show that TeaForN boosts generation quality on one Machine Translation benchmark, WMT 2014 English-French, and two News Summarization benchmarks, CNN/Dailymail and Gigaword.
NOTE: Video may display a random order of authors.
Correct author list is at the top of this page.
Connected Papers in EMNLP2020
Similar Papers
Improving Text Generation with Student-Forcing Optimal Transport
Jianqiao Li, Chunyuan Li, Guoyin Wang, Hao Fu, Yuhchen Lin, Liqun Chen, Yizhe Zhang, Chenyang Tao, Ruiyi Zhang, Wenlin Wang, Dinghan Shen, Qian Yang, Lawrence Carin,
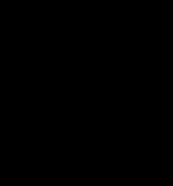
PERL: Pivot-based Domain Adaptation for Pre-trained Deep Contextualized Embedding Models
Roi Reichart, Eyal Ben David, Carmel Rabinovitz,

Multi-Stage Pre-training for Low-Resource Domain Adaptation
Rong Zhang, Revanth Gangi Reddy, Md Arafat Sultan, Vittorio Castelli, Anthony Ferritto, Radu Florian, Efsun Sarioglu Kayi, Salim Roukos, Avi Sil, Todd Ward,
