DyERNIE: Dynamic Evolution of Riemannian Manifold Embeddings for Temporal Knowledge Graph Completion
Zhen Han, Peng Chen, Yunpu Ma, Volker Tresp
Machine Learning for NLP Long Paper
You can open the pre-recorded video in a separate window.
Abstract:
There has recently been increasing interest in learning representations of temporal knowledge graphs (KGs), which record the dynamic relationships between entities over time. Temporal KGs often exhibit multiple simultaneous non-Euclidean structures, such as hierarchical and cyclic structures. However, existing embedding approaches for temporal KGs typically learn entity representations and their dynamic evolution in the Euclidean space, which might not capture such intrinsic structures very well. To this end, we propose DyERNIE, a non-Euclidean embedding approach that learns evolving entity representations in a product of Riemannian manifolds, where the composed spaces are estimated from the sectional curvatures of underlying data. Product manifolds enable our approach to better reflect a wide variety of geometric structures on temporal KGs. Besides, to capture the evolutionary dynamics of temporal KGs, we let the entity representations evolve according to a velocity vector defined in the tangent space at each timestamp. We analyze in detail the contribution of geometric spaces to representation learning of temporal KGs and evaluate our model on temporal knowledge graph completion tasks. Extensive experiments on three real-world datasets demonstrate significantly improved performance, indicating that the dynamics of multi-relational graph data can be more properly modeled by the evolution of embeddings on Riemannian manifolds.
NOTE: Video may display a random order of authors.
Correct author list is at the top of this page.
Connected Papers in EMNLP2020
Similar Papers
TeMP: Temporal Message Passing for Temporal Knowledge Graph Completion
Jiapeng Wu, Meng Cao, Jackie Chi Kit Cheung, William L. Hamilton,

Embedding Words in Non-Vector Space with Unsupervised Graph Learning
Max Ryabinin, Sergei Popov, Liudmila Prokhorenkova, Elena Voita,
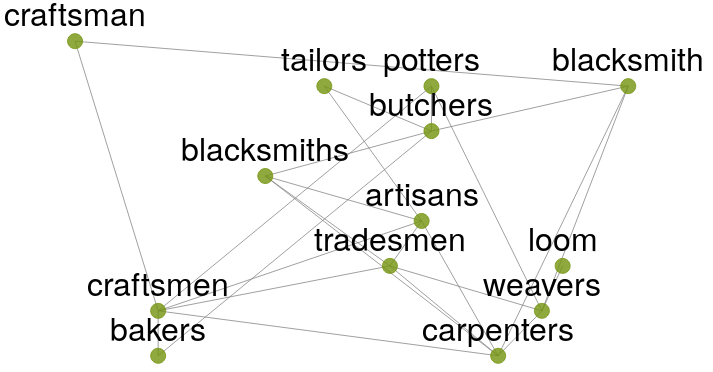