TeMP: Temporal Message Passing for Temporal Knowledge Graph Completion
Jiapeng Wu, Meng Cao, Jackie Chi Kit Cheung, William L. Hamilton
Information Extraction Long Paper
You can open the pre-recorded video in a separate window.
Abstract:
Inferring missing facts in temporal knowledge graphs (TKGs) is a fundamental and challenging task. Previous works have approached this problem by augmenting methods for static knowledge graphs to leverage time-dependent representations. However, these methods do not explicitly leverage multi-hop structural information and temporal facts from recent time steps to enhance their predictions. Additionally, prior work does not explicitly address the temporal sparsity and variability of entity distributions in TKGs. We propose the Temporal Message Passing (TeMP) framework to address these challenges by combining graph neural networks, temporal dynamics models, data imputation and frequency-based gating techniques. Experiments on standard TKG tasks show that our approach provides substantial gains compared to the previous state of the art, achieving a 10.7% average relative improvement in Hits@10 across three standard benchmarks. Our analysis also reveals important sources of variability both within and across TKG datasets, and we introduce several simple but strong baselines that outperform the prior state of the art in certain settings.
NOTE: Video may display a random order of authors.
Correct author list is at the top of this page.
Connected Papers in EMNLP2020
Similar Papers
Temporal Knowledge Base Completion: New Algorithms and Evaluation Protocols
Prachi Jain, Sushant Rathi, Mausam, Soumen Chakrabarti,
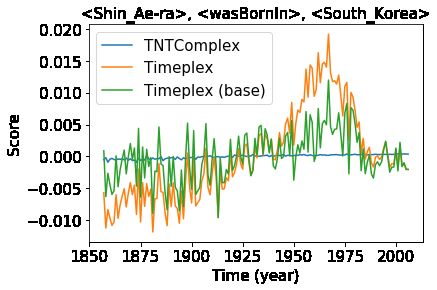
Domain Knowledge Empowered Structured Neural Net for End-to-End Event Temporal Relation Extraction
Rujun Han, Yichao Zhou, Nanyun Peng,
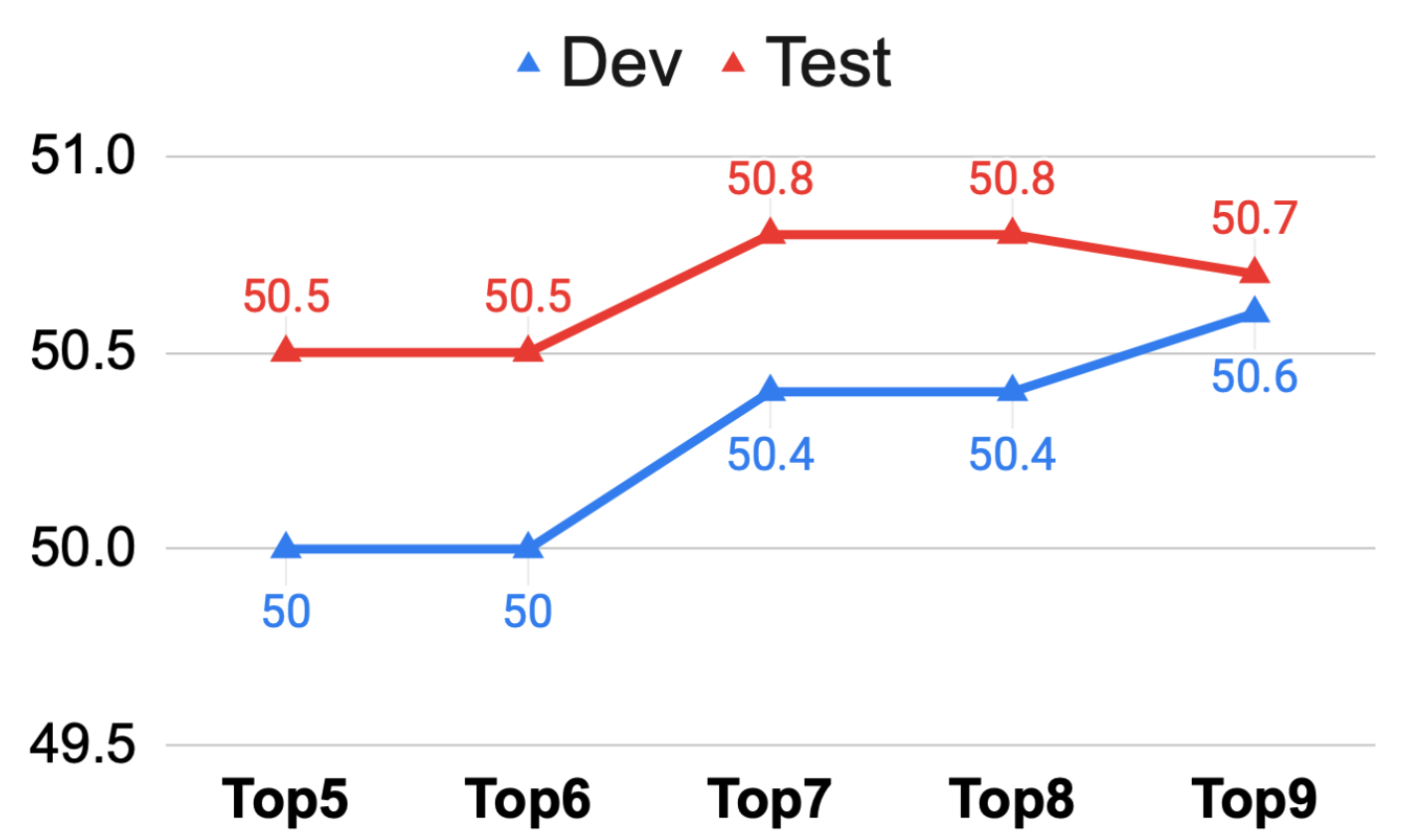
Dynamic Anticipation and Completion for Multi-Hop Reasoning over Sparse Knowledge Graph
Xin Lv, Xu Han, Lei Hou, Juanzi Li, Zhiyuan Liu, Wei Zhang, Yichi Zhang, Hao Kong, Suhui Wu,

Adaptive Attentional Network for Few-Shot Knowledge Graph Completion
Jiawei Sheng, Shu Guo, Zhenyu Chen, Juwei Yue, Lihong Wang, Tingwen Liu, Hongbo Xu,
