Quantitative argument summarization and beyond: Cross-domain key point analysis
Roy Bar-Haim, Yoav Kantor, Lilach Eden, Roni Friedman, Dan Lahav, Noam Slonim
Sentiment Analysis, Stylistic Analysis, and Argument Mining Long Paper
You can open the pre-recorded video in a separate window.
Abstract:
When summarizing a collection of views, arguments or opinions on some topic, it is often desirable not only to extract the most salient points, but also to quantify their prevalence. Work on multi-document summarization has traditionally focused on creating textual summaries, which lack this quantitative aspect. Recent work has proposed to summarize arguments by mapping them to a small set of expert-generated key points, where the salience of each key point corresponds to the number of its matching arguments. The current work advances key point analysis in two important respects: first, we develop a method for automatic extraction of key points, which enables fully automatic analysis, and is shown to achieve performance comparable to a human expert. Second, we demonstrate that the applicability of key point analysis goes well beyond argumentation data. Using models trained on publicly available argumentation datasets, we achieve promising results in two additional domains: municipal surveys and user reviews. An additional contribution is an in-depth evaluation of argument-to-key point matching models, where we substantially outperform previous results.
NOTE: Video may display a random order of authors.
Correct author list is at the top of this page.
Connected Papers in EMNLP2020
Similar Papers
Better Highlighting: Creating Sub-Sentence Summary Highlights
Sangwoo Cho, Kaiqiang Song, Chen Li, Dong Yu, Hassan Foroosh, Fei Liu,
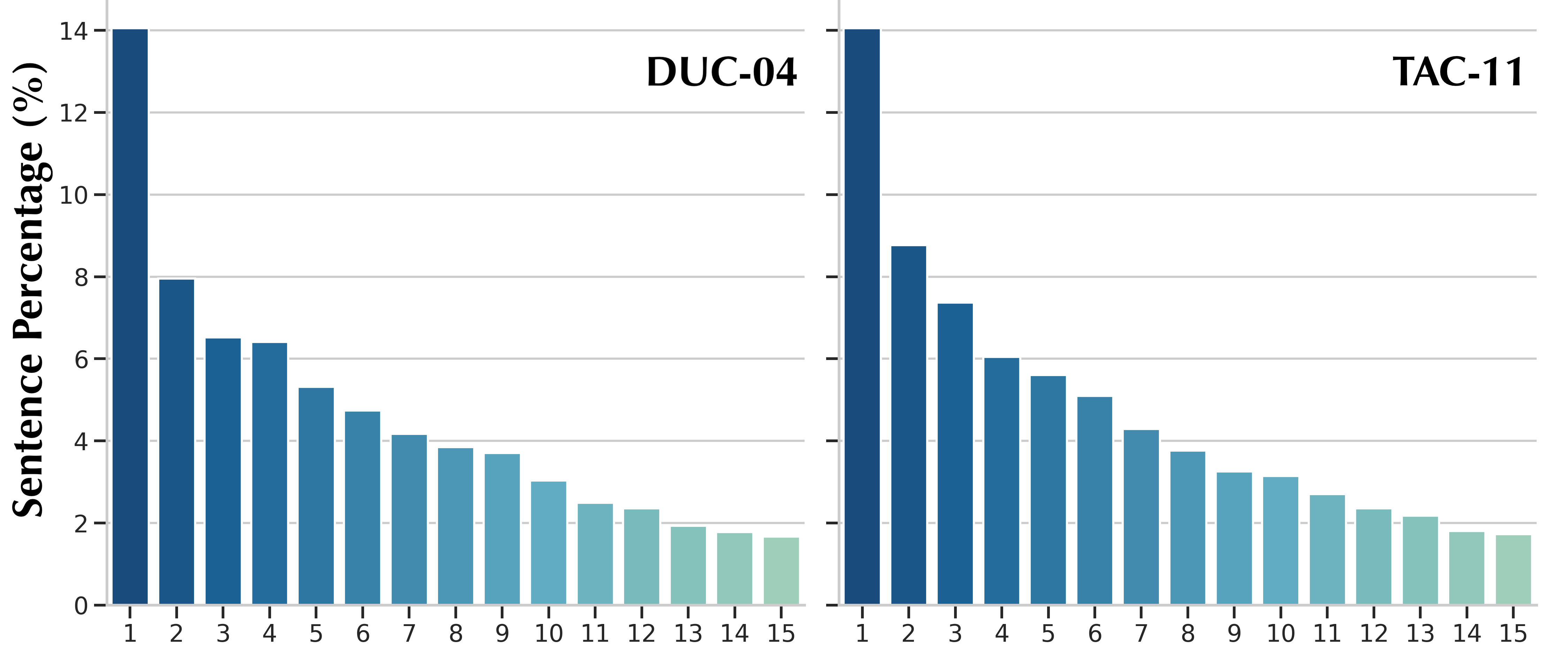
Evaluating the Factual Consistency of Abstractive Text Summarization
Wojciech Kryscinski, Bryan McCann, Caiming Xiong, Richard Socher,

What Have We Achieved on Text Summarization?
Dandan Huang, Leyang Cui, Sen Yang, Guangsheng Bao, Kun Wang, Jun Xie, Yue Zhang,

Learning from Context or Names? An Empirical Study on Neural Relation Extraction
Hao Peng, Tianyu Gao, Xu Han, Yankai Lin, Peng Li, Zhiyuan Liu, Maosong Sun, Jie Zhou,
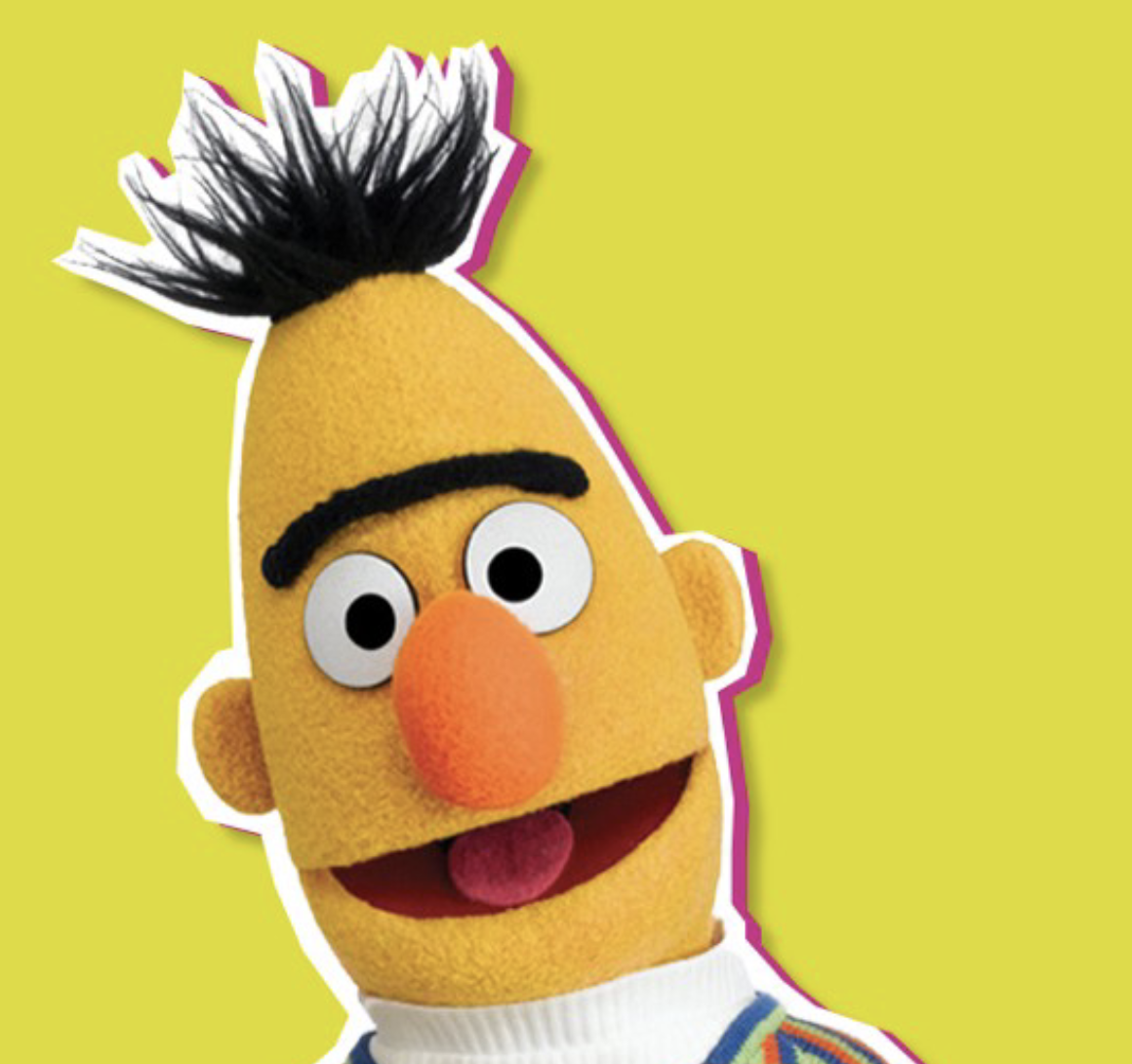