Statistical Power and Translationese in Machine Translation Evaluation
Yvette Graham, Barry Haddow, Philipp Koehn
Machine Translation and Multilinguality Long Paper
You can open the pre-recorded video in a separate window.
Abstract:
The term translationese has been used to describe features of translated text, and in this paper, we provide detailed analysis of potential adverse effects of translationese on machine translation evaluation. Our analysis shows differences in conclusions drawn from evaluations that include translationese in test data compared to experiments that tested only with text originally composed in that language. For this reason we recommend that reverse-created test data be omitted from future machine translation test sets. In addition, we provide a re-evaluation of a past machine translation evaluation claiming human-parity of MT. One important issue not previously considered is statistical power of significance tests applied to comparison of human and machine translation. Since the very aim of past evaluations was investigation of ties between human and MT systems, power analysis is of particular importance, to avoid, for example, claims of human parity simply corresponding to Type II error resulting from the application of a low powered test. We provide detailed analysis of tests used in such evaluations to provide an indication of a suitable minimum sample size for future studies.
NOTE: Video may display a random order of authors.
Correct author list is at the top of this page.
Connected Papers in EMNLP2020
Similar Papers
Can Automatic Post-Editing Improve NMT?
Shamil Chollampatt, Raymond Hendy Susanto, Liling Tan, Ewa Szymanska,
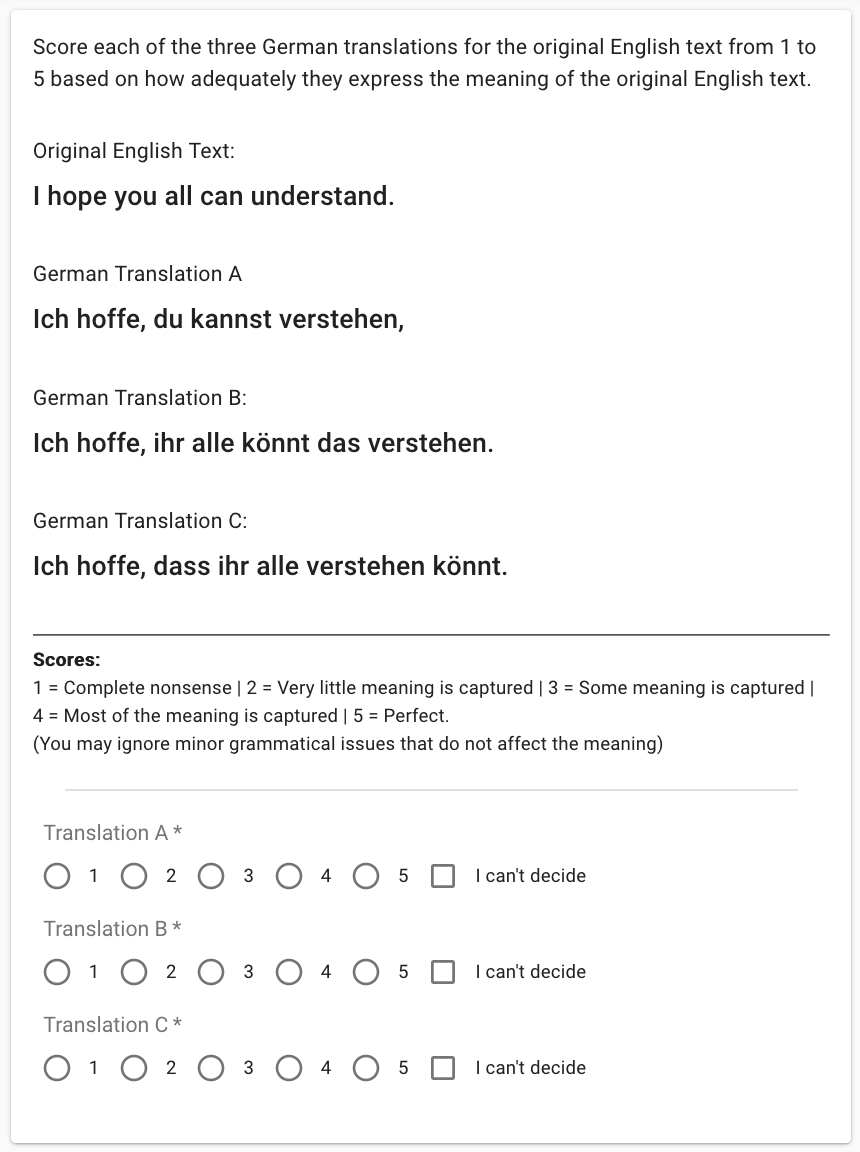
Automatic Machine Translation Evaluation in Many Languages via Zero-Shot Paraphrasing
Brian Thompson, Matt Post,

Consistent Transcription and Translation of Speech
Matthias Sperber, Hendra Setiawan, Christian Gollan, Udhay Nallasamy, Matthias Paulik,
