Translation Artifacts in Cross-lingual Transfer Learning
Mikel Artetxe, Gorka Labaka, Eneko Agirre
Machine Translation and Multilinguality Long Paper
You can open the pre-recorded video in a separate window.
Abstract:
Both human and machine translation play a central role in cross-lingual transfer learning: many multilingual datasets have been created through professional translation services, and using machine translation to translate either the test set or the training set is a widely used transfer technique. In this paper, we show that such translation process can introduce subtle artifacts that have a notable impact in existing cross-lingual models. For instance, in natural language inference, translating the premise and the hypothesis independently can reduce the lexical overlap between them, which current models are highly sensitive to. We show that some previous findings in cross-lingual transfer learning need to be reconsidered in the light of this phenomenon. Based on the gained insights, we also improve the state-of-the-art in XNLI for the translate-test and zero-shot approaches by 4.3 and 2.8 points, respectively.
NOTE: Video may display a random order of authors.
Correct author list is at the top of this page.
Connected Papers in EMNLP2020
Similar Papers
Pre-training Multilingual Neural Machine Translation by Leveraging Alignment Information
Zehui Lin, Xiao Pan, Mingxuan Wang, Xipeng Qiu, Jiangtao Feng, Hao Zhou, Lei Li,

Dynamic Data Selection and Weighting for Iterative Back-Translation
Zi-Yi Dou, Antonios Anastasopoulos, Graham Neubig,
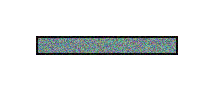
Towards Enhancing Faithfulness for Neural Machine Translation
Rongxiang Weng, Heng Yu, Xiangpeng Wei, Weihua Luo,
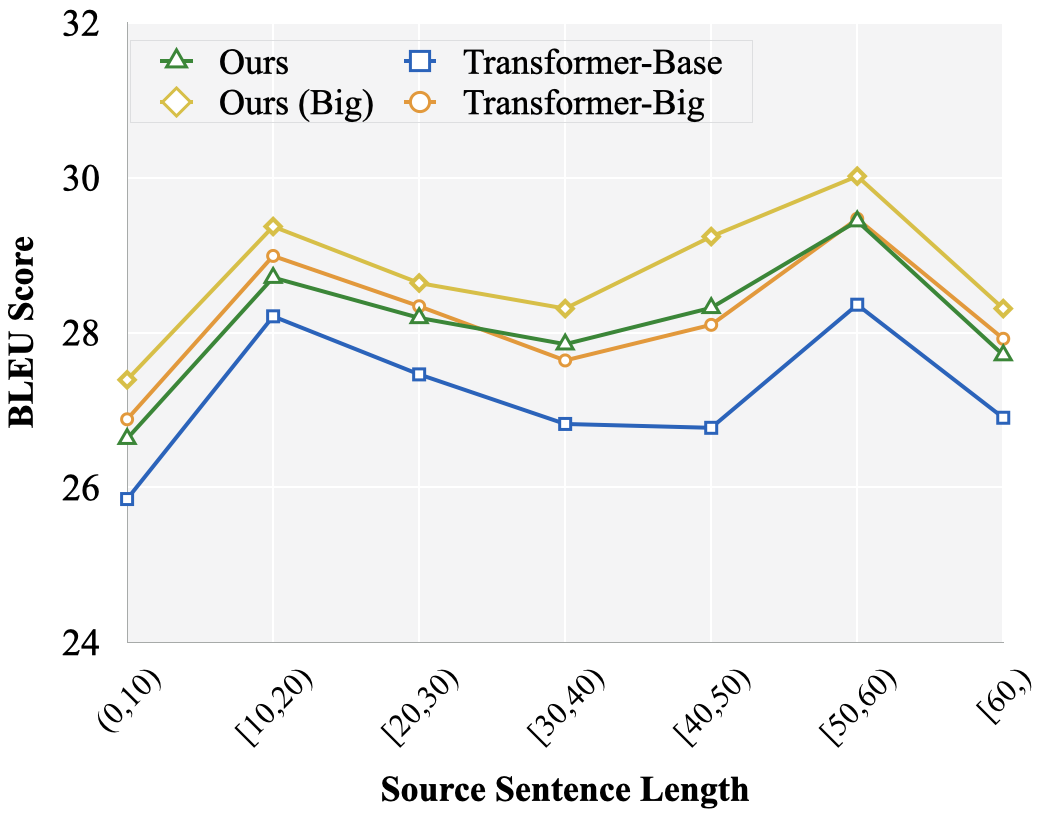
Automatic Machine Translation Evaluation in Many Languages via Zero-Shot Paraphrasing
Brian Thompson, Matt Post,
