Beyond Instructional Videos: Probing for More Diverse Visual-Textual Grounding on YouTube
Jack Hessel, Zhenhai Zhu, Bo Pang, Radu Soricut
Language Grounding to Vision, Robotics and Beyond Short Paper
You can open the pre-recorded video in a separate window.
Abstract:
Pretraining from unlabelled web videos has quickly become the de-facto means of achieving high performance on many video understanding tasks. Features are learned via prediction of grounded relationships between visual content and automatic speech recognition (ASR) tokens. However, prior pretraining work has been limited to only instructional videos; a priori, we expect this domain to be relatively "easy:" speakers in instructional videos will often reference the literal objects/actions being depicted. We ask: can similar models be trained on more diverse video corpora? And, if so, what types of videos are "grounded" and what types are not? We fit a representative pretraining model to the diverse YouTube8M dataset, and study its success and failure cases. We find that visual-textual grounding is indeed possible across previously unexplored video categories, and that pretraining on a more diverse set results in representations that generalize to both non-instructional and instructional domains.
NOTE: Video may display a random order of authors.
Correct author list is at the top of this page.
Connected Papers in EMNLP2020
Similar Papers
What is More Likely to Happen Next? Video-and-Language Future Event Prediction
Jie Lei, Licheng Yu, Tamara Berg, Mohit Bansal,
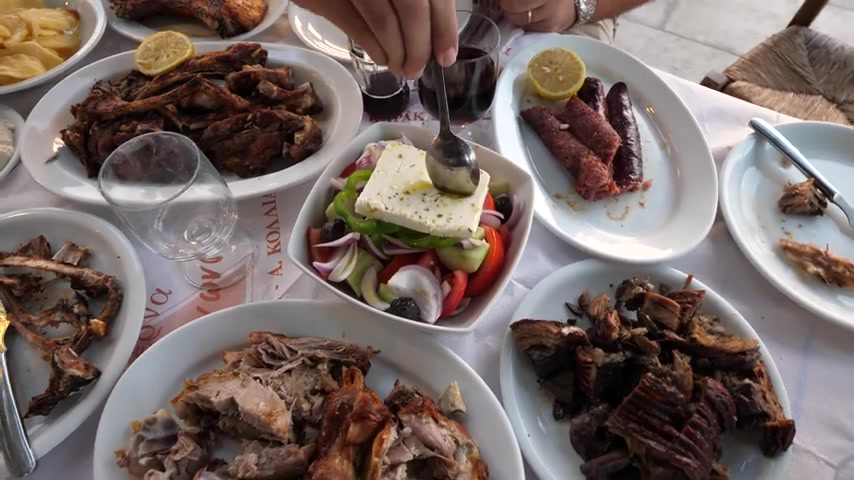
Reading Between the Lines: Exploring Infilling in Visual Narratives
Khyathi Raghavi Chandu, Ruo-Ping Dong, Alan W Black,
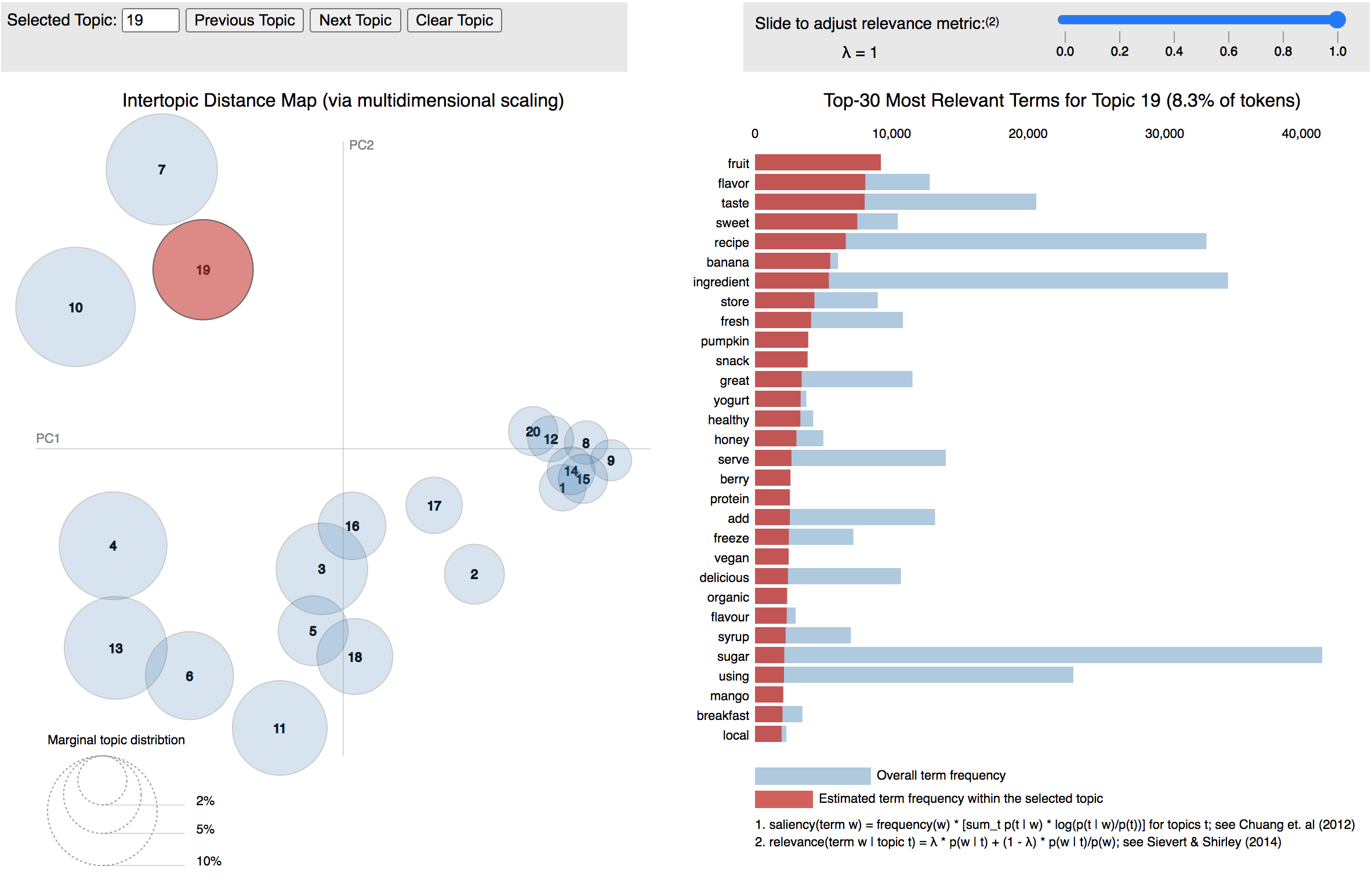
MovieChats: Chat like Humans in a Closed Domain
Hui Su, Xiaoyu Shen, Zhou Xiao, Zheng Zhang, Ernie Chang, Cheng Zhang, Cheng Niu, Jie Zhou,
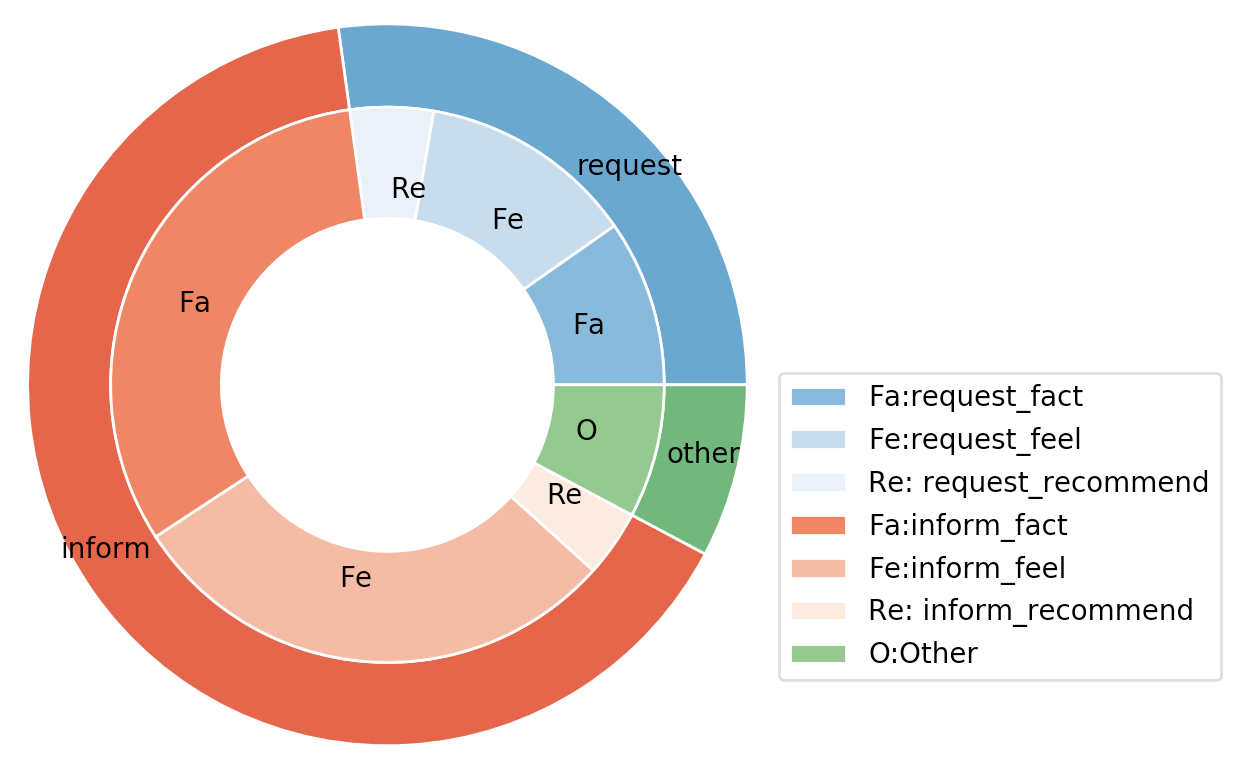
Video2Commonsense: Generating Commonsense Descriptions to Enrich Video Captioning
Zhiyuan Fang, Tejas Gokhale, Pratyay Banerjee, Chitta Baral, Yezhou Yang,
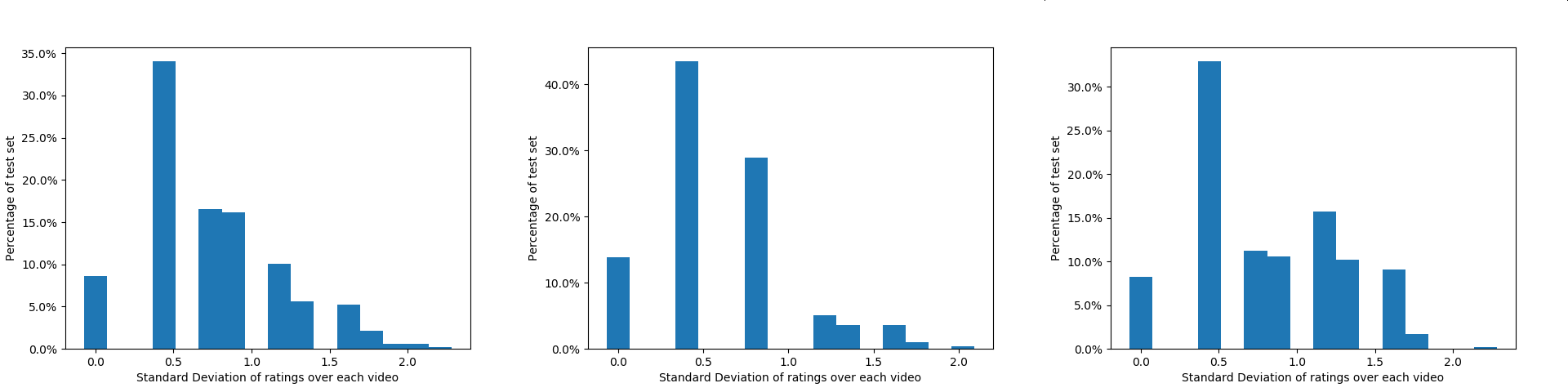