Generating Image Descriptions via Sequential Cross-Modal Alignment Guided by Human Gaze
Ece Takmaz, Sandro Pezzelle, Lisa Beinborn, Raquel Fernández
Linguistic Theories, Cognitive Modeling and Psycholinguistics Long Paper
You can open the pre-recorded video in a separate window.
Abstract:
When speakers describe an image, they tend to look at objects before mentioning them. In this paper, we investigate such sequential cross-modal alignment by modelling the image description generation process computationally. We take as our starting point a state-of-the-art image captioning system and develop several model variants that exploit information from human gaze patterns recorded during language production. In particular, we propose the first approach to image description generation where visual processing is modelled sequentially. Our experiments and analyses confirm that better descriptions can be obtained by exploiting gaze-driven attention and shed light on human cognitive processes by comparing different ways of aligning the gaze modality with language production. We find that processing gaze data sequentially leads to descriptions that are better aligned to those produced by speakers, more diverse, and more natural---particularly when gaze is encoded with a dedicated recurrent component.
NOTE: Video may display a random order of authors.
Correct author list is at the top of this page.
Connected Papers in EMNLP2020
Similar Papers
Simultaneous Machine Translation with Visual Context
Ozan Caglayan, Julia Ive, Veneta Haralampieva, Pranava Madhyastha, Loïc Barrault, Lucia Specia,
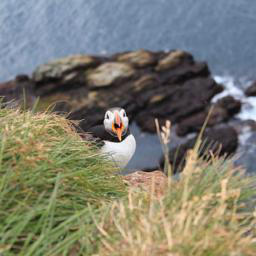
Room-Across-Room: Multilingual Vision-and-Language Navigation with Dense Spatiotemporal Grounding
Alexander Ku, Peter Anderson, Roma Patel, Eugene Ie, Jason Baldridge,
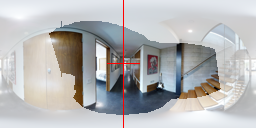
Where Are You? Localization from Embodied Dialog
Meera Hahn, Jacob Krantz, Dhruv Batra, Devi Parikh, James Rehg, Stefan Lee, Peter Anderson,
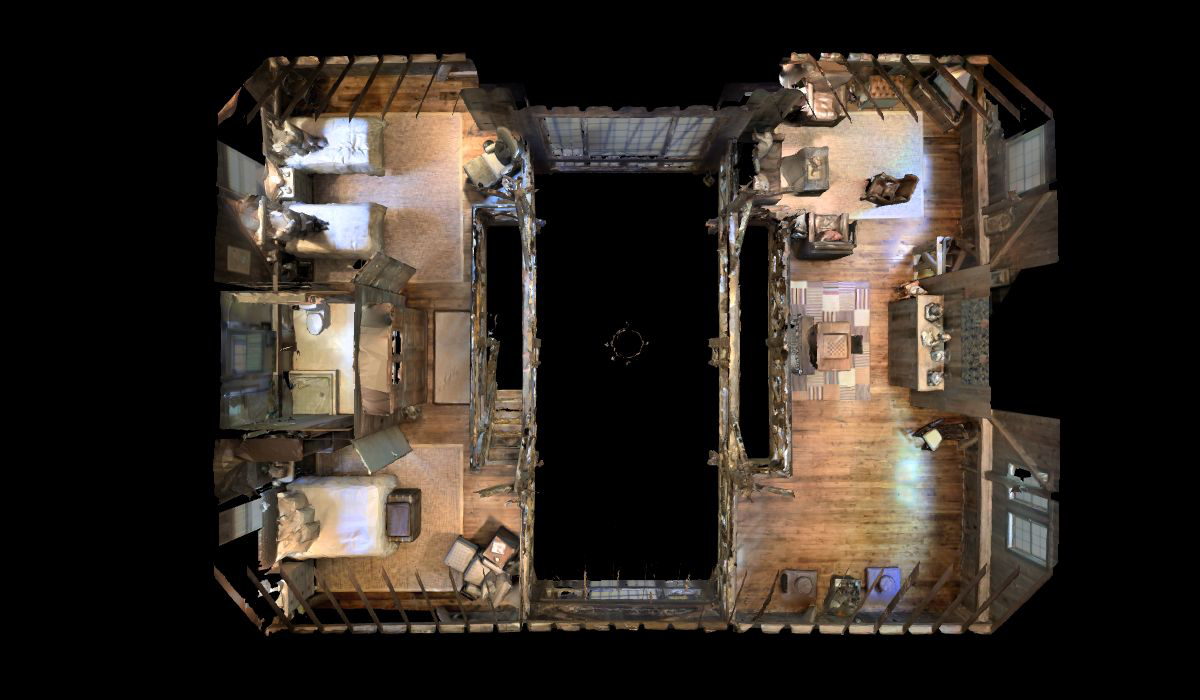