VCDM: Leveraging Variational Bi-encoding and Deep Contextualized Word Representations for Improved Definition Modeling
Machel Reid, Edison Marrese-Taylor, Yutaka Matsuo
Discourse and Pragmatics Long Paper
You can open the pre-recorded video in a separate window.
Abstract:
In this paper, we tackle the task of definition modeling, where the goal is to learn to generate definitions of words and phrases. Existing approaches for this task are discriminative, combining distributional and lexical semantics in an implicit rather than direct way. To tackle this issue we propose a generative model for the task, introducing a continuous latent variable to explicitly model the underlying relationship between a phrase used within a context and its definition. We rely on variational inference for estimation and leverage contextualized word embeddings for improved performance. Our approach is evaluated on four existing challenging benchmarks with the addition of two new datasets, "Cambridge" and the first non-English corpus "Robert", which we release to complement our empirical study. Our Variational Contextual Definition Modeler (VCDM) achieves state-of-the-art performance in terms of automatic and human evaluation metrics, demonstrating the effectiveness of our approach.
NOTE: Video may display a random order of authors.
Correct author list is at the top of this page.
Connected Papers in EMNLP2020
Similar Papers
Grounded Compositional Outputs for Adaptive Language Modeling
Nikolaos Pappas, Phoebe Mulcaire, Noah A. Smith,
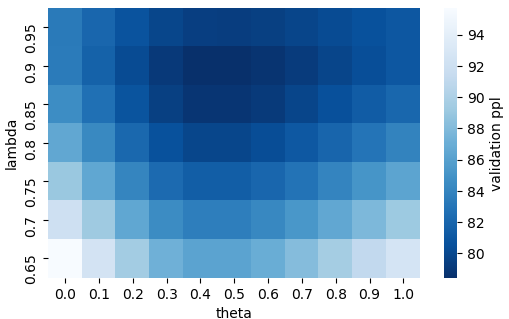
Generationary or “How We Went beyond Word Sense Inventories and Learned to Gloss”
Michele Bevilacqua, Marco Maru, Roberto Navigli,

XL-WiC: A Multilingual Benchmark for Evaluating Semantic Contextualization
Alessandro Raganato, Tommaso Pasini, Jose Camacho-Collados, Mohammad Taher Pilehvar,
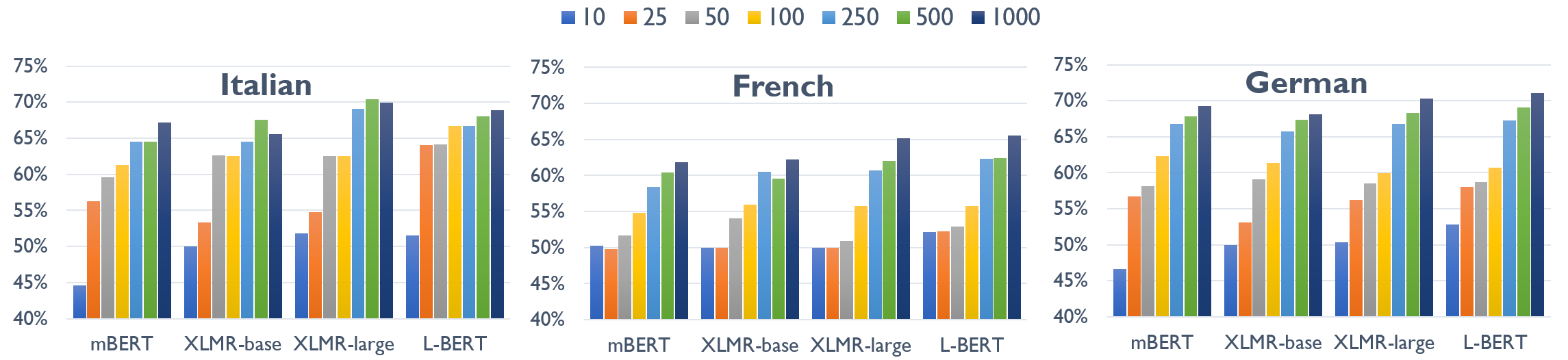
On the Sentence Embeddings from Pre-trained Language Models
Bohan Li, Hao Zhou, Junxian He, Mingxuan Wang, Yiming Yang, Lei Li,
