Less is More: Attention Supervision with Counterfactuals for Text Classification
Seungtaek Choi, Haeju Park, Jinyoung Yeo, Seung-won Hwang
Interpretability and Analysis of Models for NLP Long Paper
You can open the pre-recorded video in a separate window.
Abstract:
We aim to leverage human and machine intelligence together for attention supervision. Specifically, we show that human annotation cost can be kept reasonably low, while its quality can be enhanced by machine self-supervision. Specifically, for this goal, we explore the advantage of counterfactual reasoning, over associative reasoning typically used in attention supervision. Our empirical results show that this machine-augmented human attention supervision is more effective than existing methods requiring a higher annotation cost, in text classification tasks, including sentiment analysis and news categorization.
NOTE: Video may display a random order of authors.
Correct author list is at the top of this page.
Connected Papers in EMNLP2020
Similar Papers
DAGA: Data Augmentation with a Generation Approach forLow-resource Tagging Tasks
Bosheng Ding, Linlin Liu, Lidong Bing, Canasai Kruengkrai, Thien Hai Nguyen, Shafiq Joty, Luo Si, Chunyan Miao,
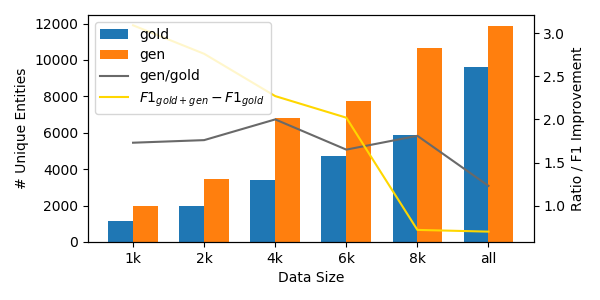
DualTKB: A Dual Learning Bridge between Text and Knowledge Base
Pierre Dognin, Igor Melnyk, Inkit Padhi, Cicero Nogueira dos Santos, Payel Das,
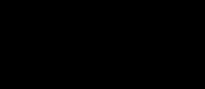
Combining Self-Training and Self-Supervised Learning for Unsupervised Disfluency Detection
Shaolei Wang, Zhongyuan Wang, Wanxiang Che, Ting Liu,
