SSCR: Iterative Language-Based Image Editing via Self-Supervised Counterfactual Reasoning
Tsu-Jui Fu, Xin Wang, Scott Grafton, Miguel Eckstein, William Yang Wang
Language Grounding to Vision, Robotics and Beyond Long Paper
You can open the pre-recorded video in a separate window.
Abstract:
Iterative Language-Based Image Editing (ILBIE) tasks follow iterative instructions to edit images step by step. Data scarcity is a significant issue for ILBIE as it is challenging to collect large-scale examples of images before and after instruction-based changes. Yet, humans still accomplish these editing tasks even when presented with an unfamiliar image-instruction pair. Such ability results from counterfactual thinking, the ability to think about possible alternatives to events that have happened already. In this paper, we introduce a Self-Supervised Counterfactual Reasoning (SSCR) framework that incorporates counterfactual thinking to overcome data scarcity. SSCR allows the model to consider out-of-distribution instructions paired with previous images. With the help of cross-task consistency (CTC), we train these counterfactual instructions in a self-supervised scenario. Extensive results show that SSCR improves the correctness of ILBIE in terms of both object identity and position, establishing a new state of the art (SOTA) on two IBLIE datasets (i-CLEVR and CoDraw). Even with only 50\% of the training data, SSCR achieves a comparable result to using complete data.
NOTE: Video may display a random order of authors.
Correct author list is at the top of this page.
Connected Papers in EMNLP2020
Similar Papers
X-LXMERT: Paint, Caption and Answer Questions with Multi-Modal Transformers
Jaemin Cho, Jiasen Lu, Dustin Schwenk, Hannaneh Hajishirzi, Aniruddha Kembhavi,
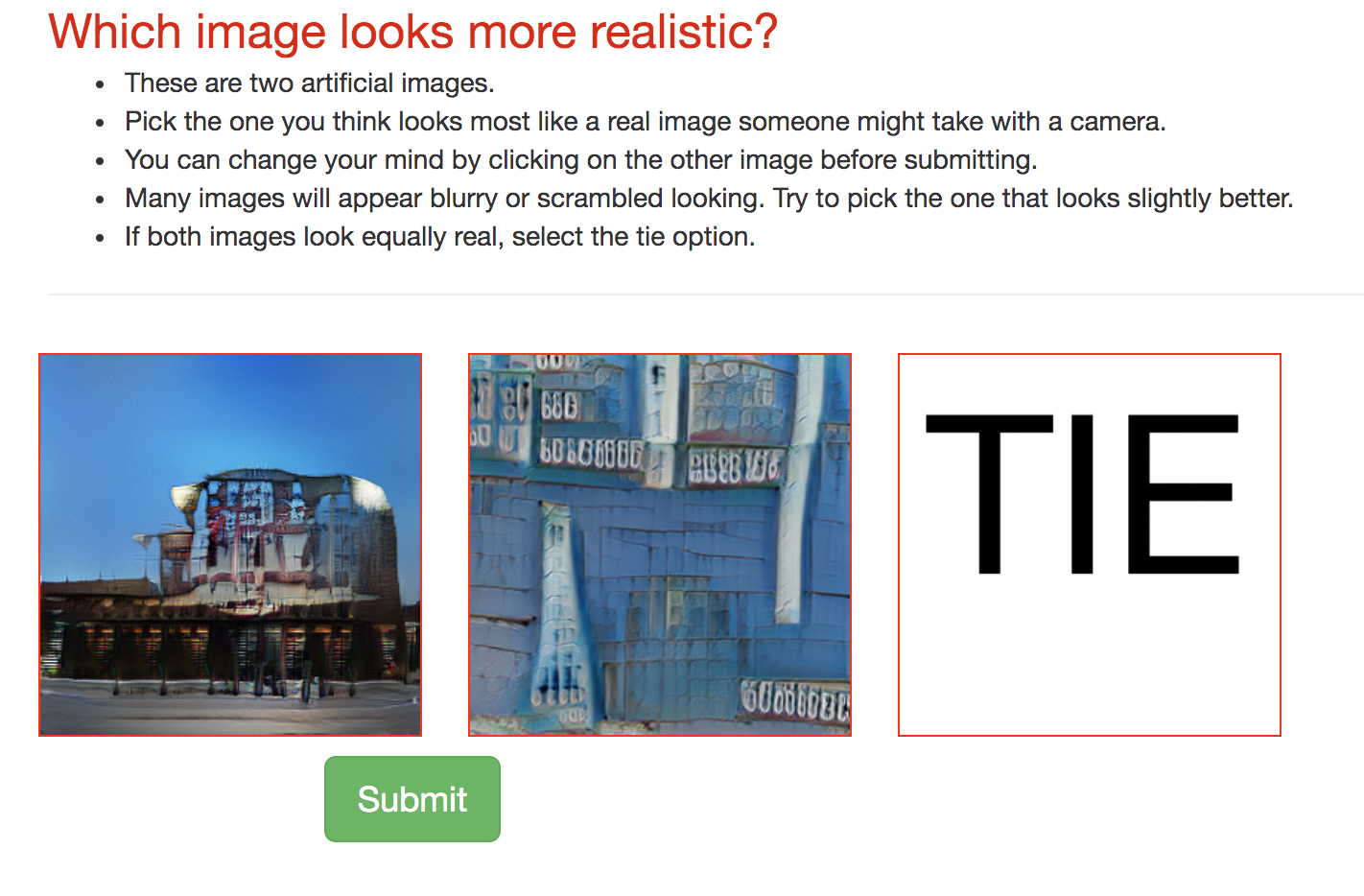
Generating Image Descriptions via Sequential Cross-Modal Alignment Guided by Human Gaze
Ece Takmaz, Sandro Pezzelle, Lisa Beinborn, Raquel Fernández,
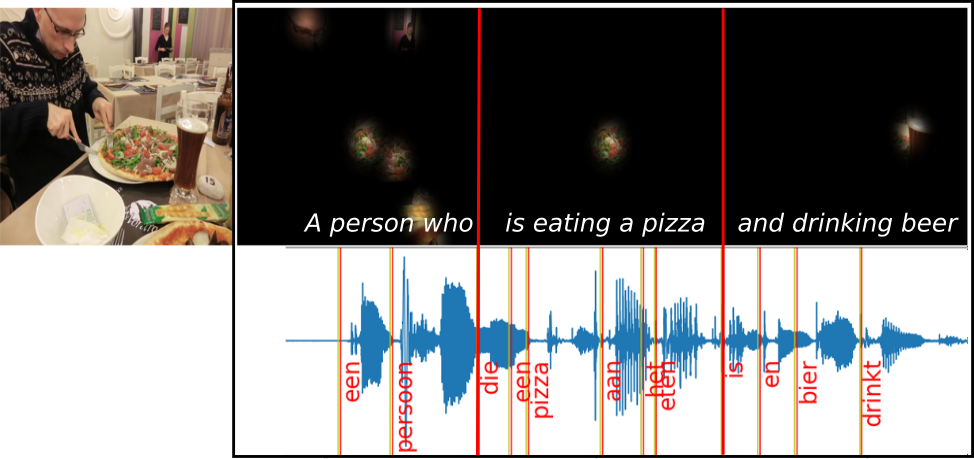
PowerTransformer: Unsupervised Controllable Revision for Biased Language Correction
Xinyao Ma, Maarten Sap, Hannah Rashkin, Yejin Choi,
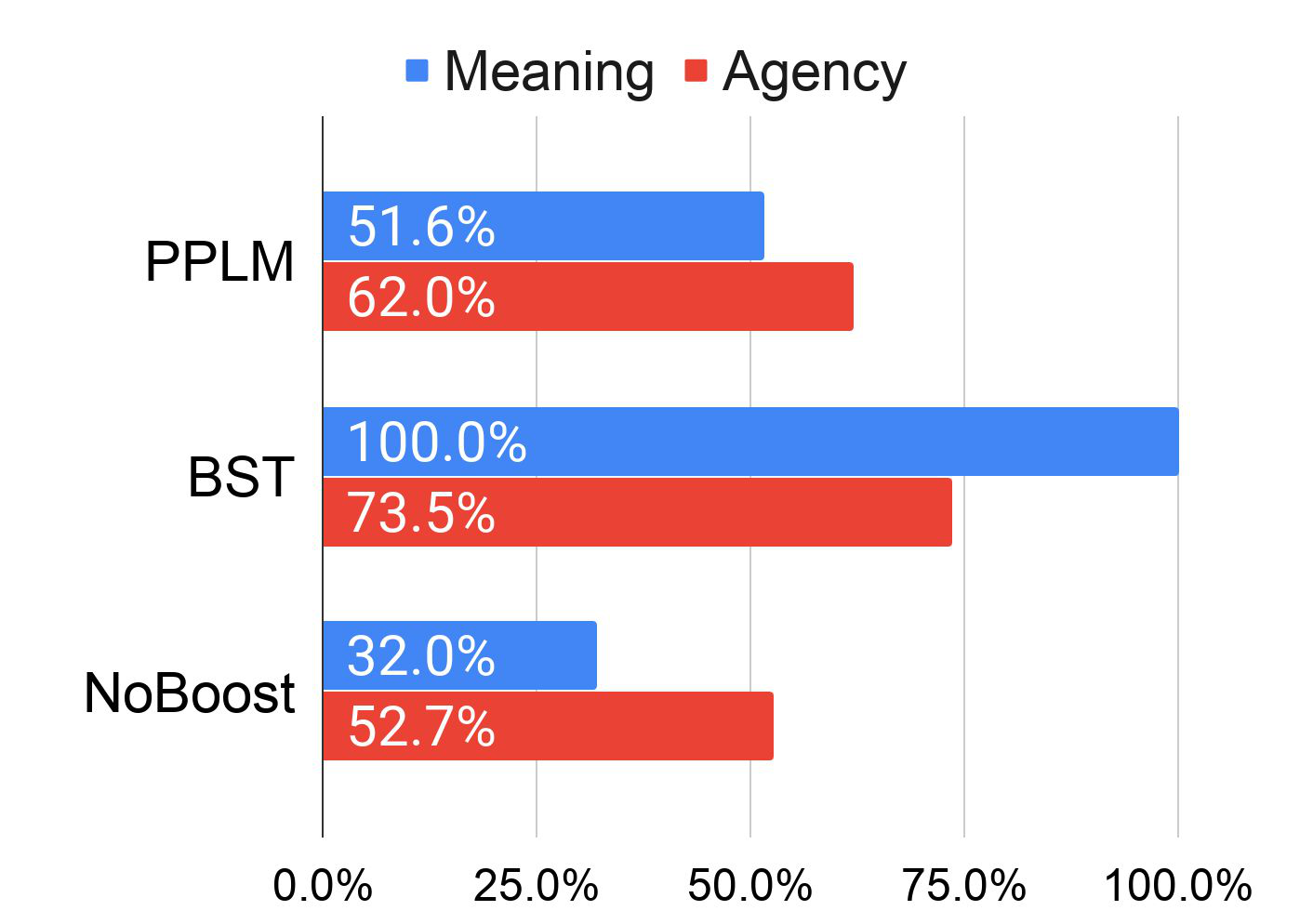
Substance over Style: Document-Level Targeted Content Transfer
Allison Hegel, Sudha Rao, Asli Celikyilmaz, Bill Dolan,
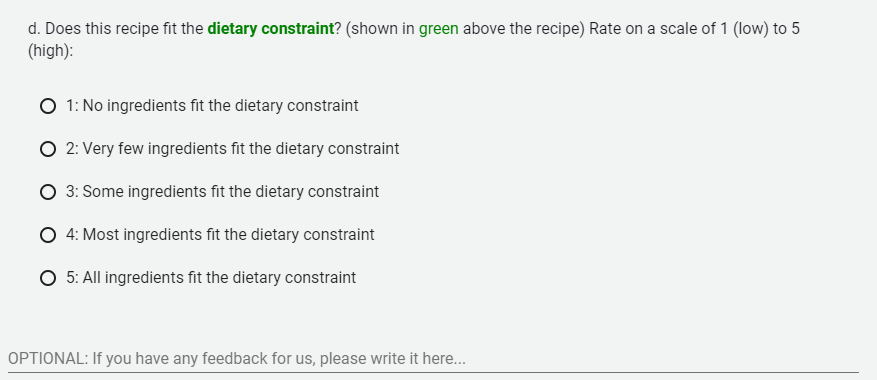