An Empirical Study of Generation Order for Machine Translation
William Chan, Mitchell Stern, Jamie Kiros, Jakob Uszkoreit
Machine Translation and Multilinguality Long Paper
You can open the pre-recorded video in a separate window.
Abstract:
In this work, we present an empirical study of generation order for machine translation. Building on recent advances in insertion-based modeling, we first introduce a soft order-reward framework that enables us to train models to follow arbitrary oracle generation policies. We then make use of this framework to explore a large variety of generation orders, including uninformed orders, location-based orders, frequency-based orders, content-based orders, and model-based orders. Curiously, we find that for the WMT'14 English $\to$ German and WMT'18 English $\to$ Chinese translation tasks, order does not have a substantial impact on output quality. Moreover, for English $\to$ German, we even discover that unintuitive orderings such as alphabetical and shortest-first can match the performance of a standard Transformer, suggesting that traditional left-to-right generation may not be necessary to achieve high performance.
NOTE: Video may display a random order of authors.
Correct author list is at the top of this page.
Connected Papers in EMNLP2020
Similar Papers
POINTER: Constrained Progressive Text Generation via Insertion-based Generative Pre-training
Yizhe Zhang, Guoyin Wang, Chunyuan Li, Zhe Gan, Chris Brockett, Bill Dolan,
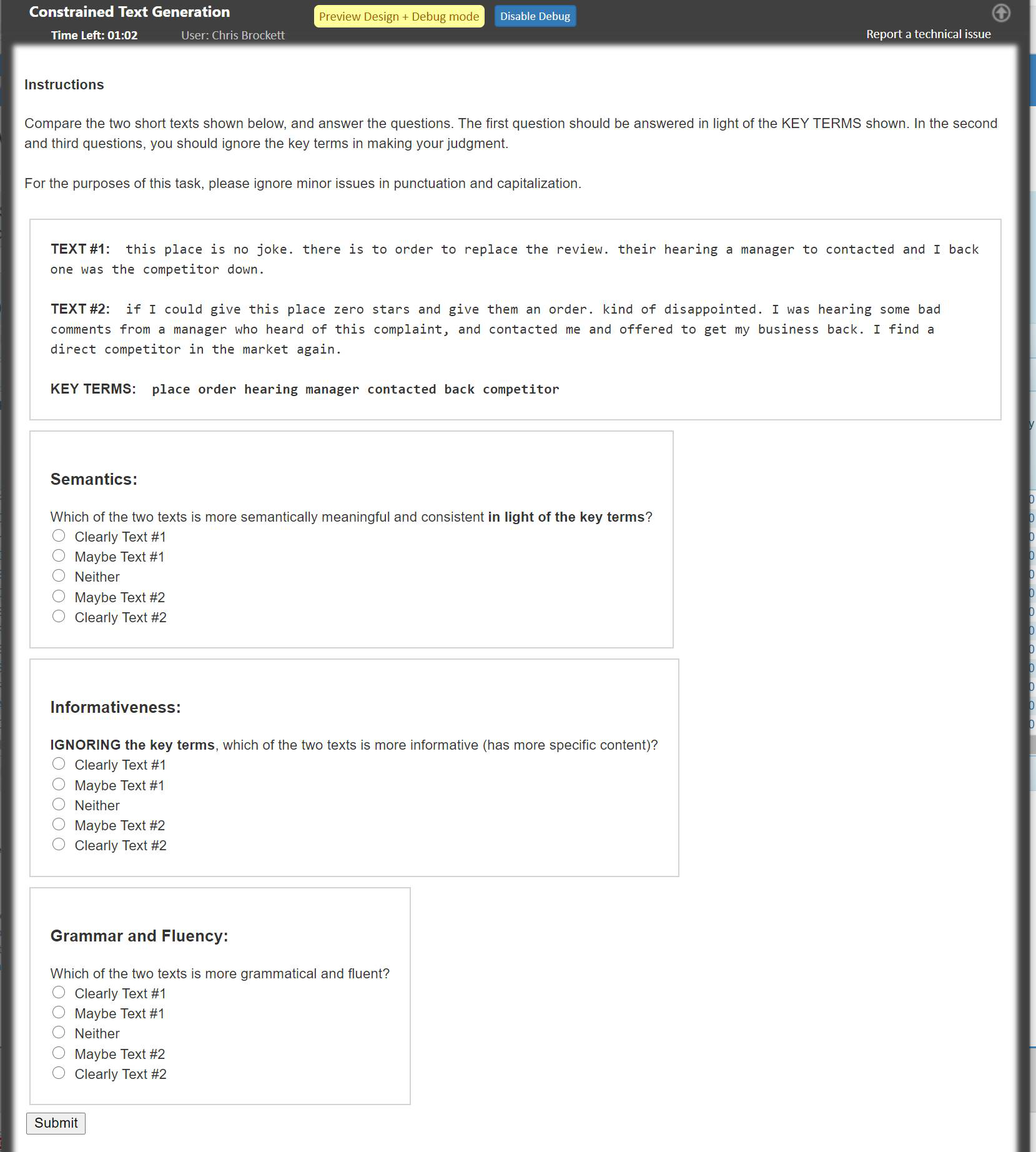
Substance over Style: Document-Level Targeted Content Transfer
Allison Hegel, Sudha Rao, Asli Celikyilmaz, Bill Dolan,
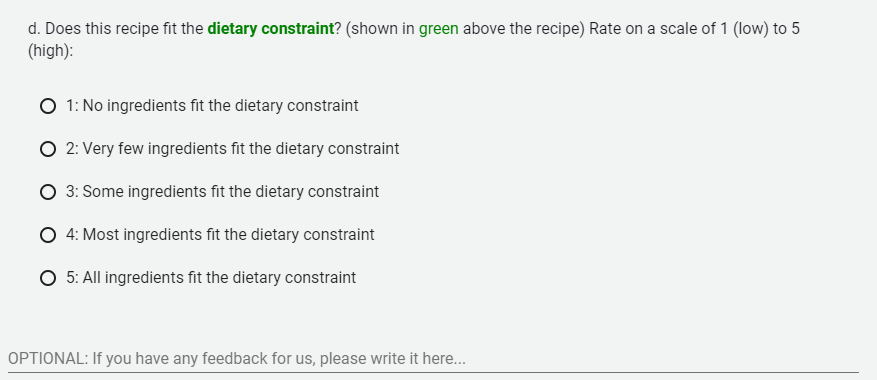