Understanding the Mechanics of SPIGOT: Surrogate Gradients for Latent Structure Learning
Tsvetomila Mihaylova, Vlad Niculae, André F. T. Martins
Machine Learning for NLP Long Paper
You can open the pre-recorded video in a separate window.
Abstract:
Latent structure models are a powerful tool for modeling language data: they can mitigate the error propagation and annotation bottleneck in pipeline systems, while simultaneously uncovering linguistic insights about the data. One challenge with end-to-end training of these models is the argmax operation, which has null gradient. In this paper, we focus on surrogate gradients, a popular strategy to deal with this problem. We explore latent structure learning through the angle of pulling back the downstream learning objective. In this paradigm, we discover a principled motivation for both the straight-through estimator (STE) as well as the recently-proposed SPIGOT -- a variant of STE for structured models. Our perspective leads to new algorithms in the same family. We empirically compare the known and the novel pulled-back estimators against the popular alternatives, yielding new insight for practitioners and revealing intriguing failure cases.
NOTE: Video may display a random order of authors.
Correct author list is at the top of this page.
Connected Papers in EMNLP2020
Similar Papers
Optimus: Organizing Sentences via Pre-trained Modeling of a Latent Space
Chunyuan Li, Xiang Gao, Yuan Li, Baolin Peng, Xiujun Li, Yizhe Zhang, Jianfeng Gao,
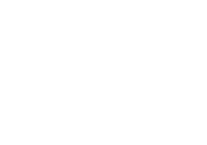
Cold-start Active Learning through Self-supervised Language Modeling
Michelle Yuan, Hsuan-Tien Lin, Jordan Boyd-Graber,

VCDM: Leveraging Variational Bi-encoding and Deep Contextualized Word Representations for Improved Definition Modeling
Machel Reid, Edison Marrese-Taylor, Yutaka Matsuo,

How do Decisions Emerge across Layers in Neural Models? Interpretation with Differentiable Masking
Nicola De Cao, Michael Sejr Schlichtkrull, Wilker Aziz, Ivan Titov,
