MEGA RST Discourse Treebanks with Structure and Nuclearity from Scalable Distant Sentiment Supervision
Patrick Huber, Giuseppe Carenini
Discourse and Pragmatics Long Paper
You can open the pre-recorded video in a separate window.
Abstract:
The lack of large and diverse discourse treebanks hinders the application of data-driven approaches, such as deep-learning, to RST-style discourse parsing. In this work, we present a novel scalable methodology to automatically generate discourse treebanks using distant supervision from sentiment annotated datasets, creating and publishing MEGA-DT, a new large-scale discourse-annotated corpus. Our approach generates discourse trees incorporating structure and nuclearity for documents of arbitrary length by relying on an efficient heuristic beam-search strategy, extended with a stochastic component. Experiments on multiple datasets indicate that a discourse parser trained on our MEGA-DT treebank delivers promising inter-domain performance gains when compared to parsers trained on human-annotated discourse corpora.
NOTE: Video may display a random order of authors.
Correct author list is at the top of this page.
Connected Papers in EMNLP2020
Similar Papers
Syntactic Structure Distillation Pretraining for Bidirectional Encoders
Adhiguna Kuncoro, Lingpeng Kong, Daniel Fried, Dani Yogatama, Laura Rimell, Chris Dyer, Phil Blunsom,

TED-CDB: A Large-Scale Chinese Discourse Relation Dataset on TED Talks
Wanqiu Long, Bonnie Webber, Deyi Xiong,
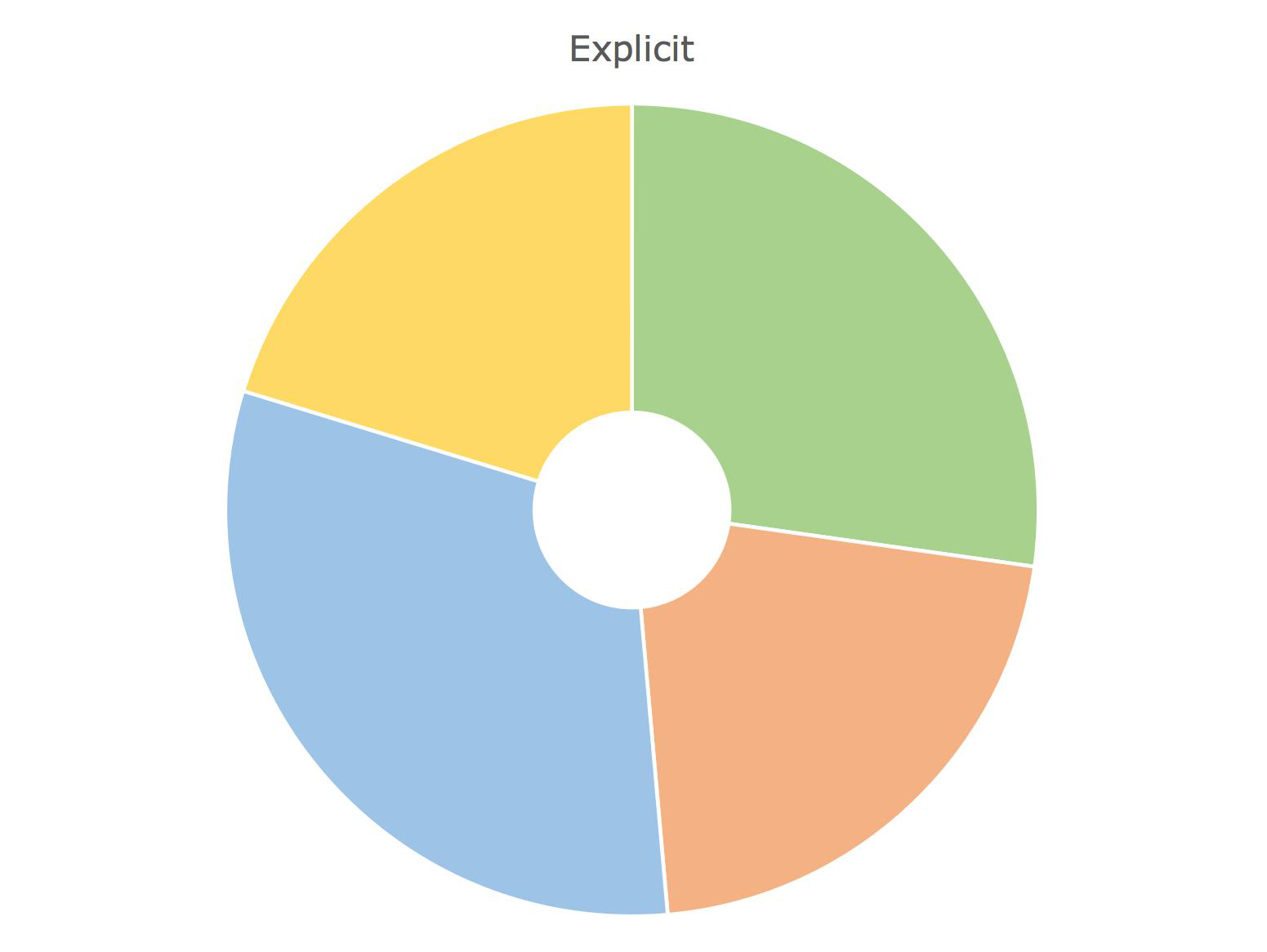
SLURP: A Spoken Language Understanding Resource Package
Emanuele Bastianelli, Andrea Vanzo, Pawel Swietojanski, Verena Rieser,

Structured Attention for Unsupervised Dialogue Structure Induction
Liang Qiu, Yizhou Zhao, Weiyan Shi, Yuan Liang, Feng Shi, Tao Yuan, Zhou Yu, Song-Chun Zhu,
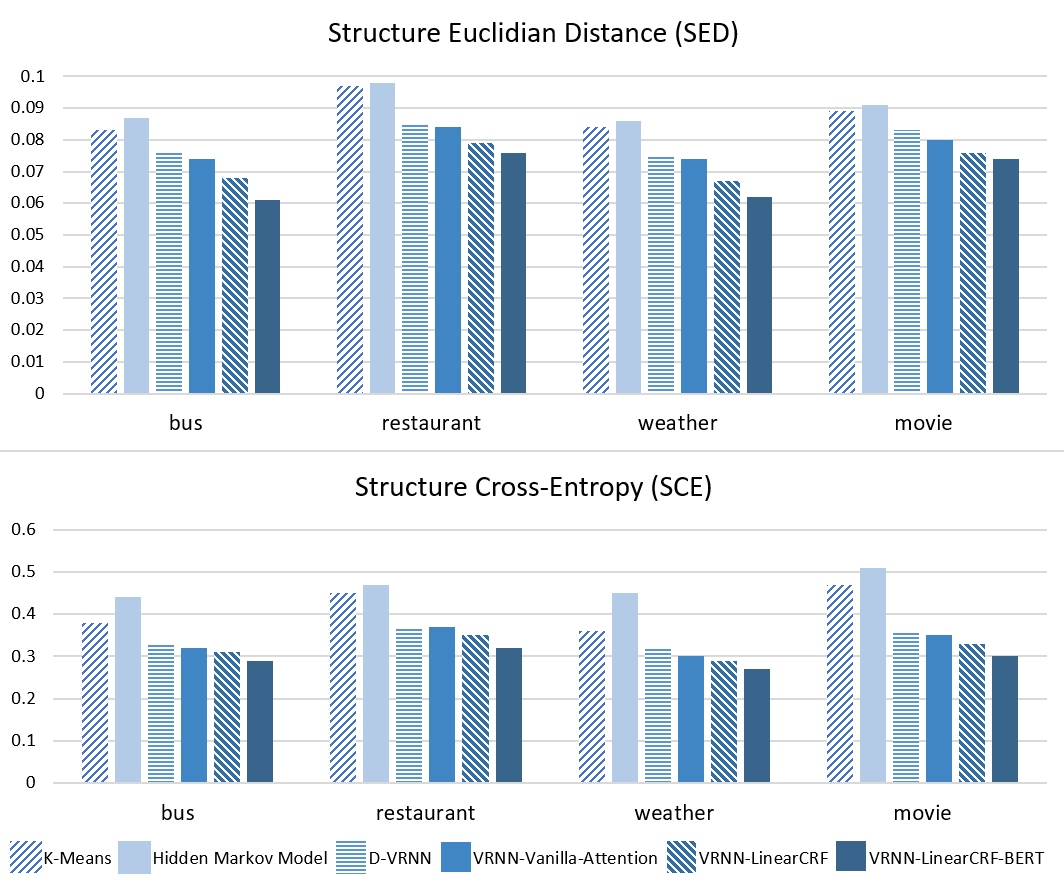