Improving Out-of-Scope Detection in Intent Classification by Using Embeddings of the Word Graph Space of the Classes
Paulo Cavalin, Victor Henrique Alves Ribeiro, Ana Appel, Claudio Pinhanez
Dialog and Interactive Systems Long Paper
You can open the pre-recorded video in a separate window.
Abstract:
This paper explores how intent classification can be improved by representing the class labels not as a discrete set of symbols but as a space where the word graphs associated to each class are mapped using typical graph embedding techniques. The approach, inspired by a previous algorithm used for an inverse dictionary task, allows the classification algorithm to take in account inter-class similarities provided by the repeated occurrence of some words in the training examples of the different classes. The classification is carried out by mapping text embeddings to the word graph embeddings of the classes. Focusing solely on improving the representation of the class label set, we show in experiments conducted in both private and public intent classification datasets, that better detection of out-of-scope examples (OOS) is achieved and, as a consequence, that the overall accuracy of intent classification is also improved. In particular, using the recently-released \emph{Larson dataset}, an error of about 9.9% has been achieved for OOS detection, beating the previous state-of-the-art result by more than 31 percentage points.
NOTE: Video may display a random order of authors.
Correct author list is at the top of this page.
Connected Papers in EMNLP2020
Similar Papers
Text Classification Using Label Names Only: A Language Model Self-Training Approach
Yu Meng, Yunyi Zhang, Jiaxin Huang, Chenyan Xiong, Heng Ji, Chao Zhang, Jiawei Han,
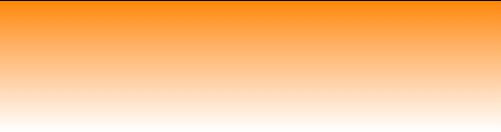
Learning from Context or Names? An Empirical Study on Neural Relation Extraction
Hao Peng, Tianyu Gao, Xu Han, Yankai Lin, Peng Li, Zhiyuan Liu, Maosong Sun, Jie Zhou,
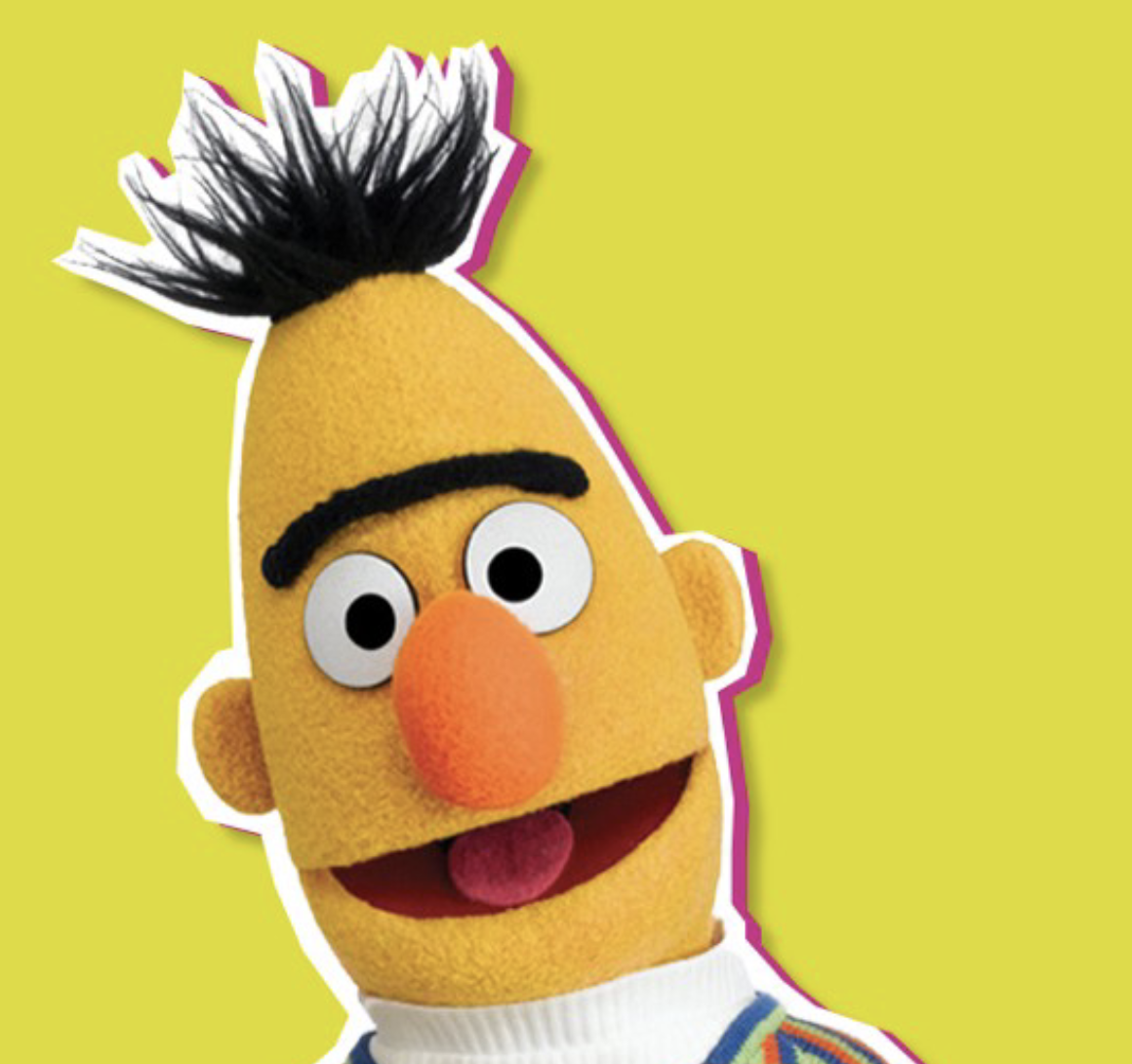
VCDM: Leveraging Variational Bi-encoding and Deep Contextualized Word Representations for Improved Definition Modeling
Machel Reid, Edison Marrese-Taylor, Yutaka Matsuo,

Don't Neglect the Obvious: On the Role of Unambiguous Words in Word Sense Disambiguation
Daniel Loureiro, Jose Camacho-Collados,
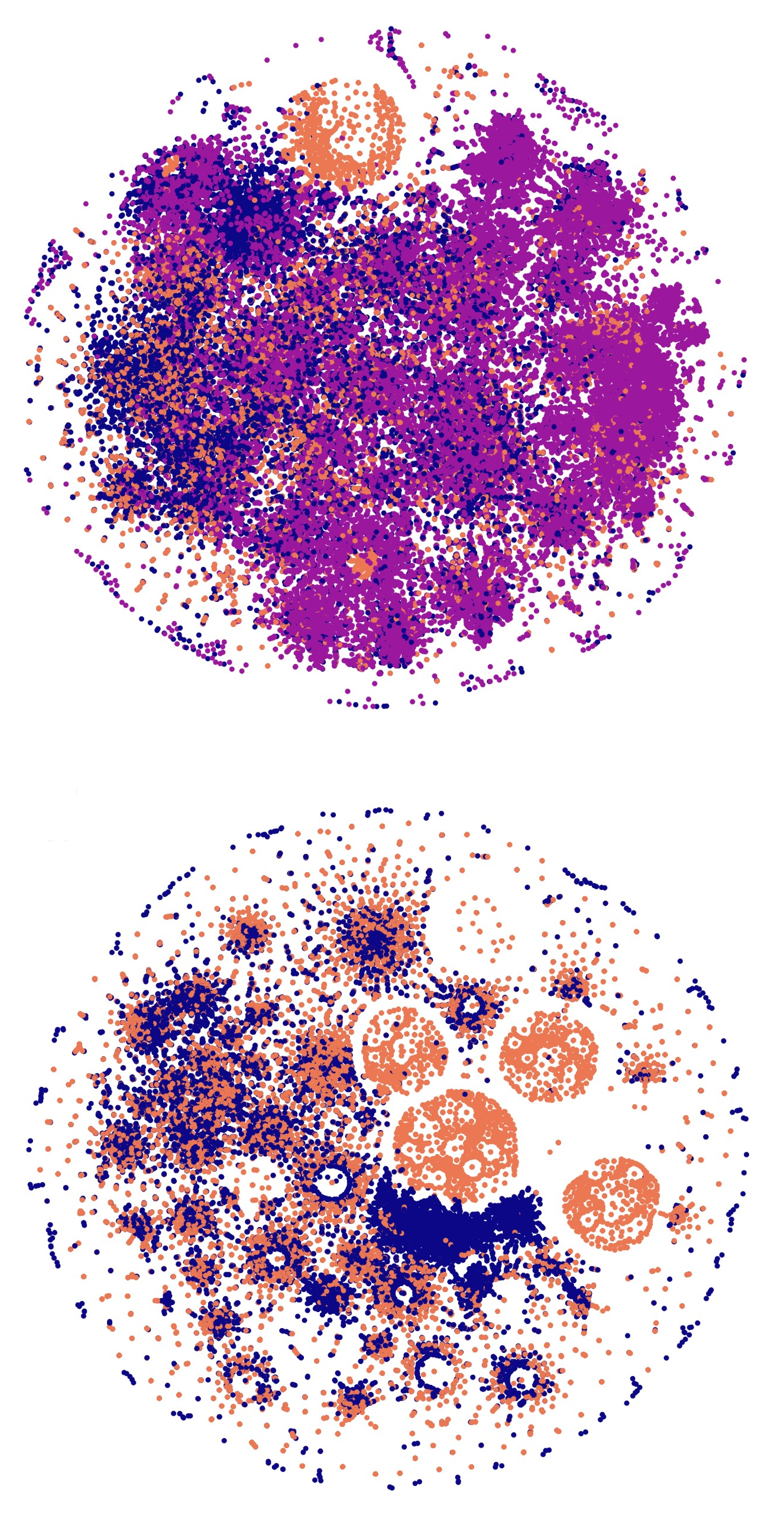