Task-oriented Domain-specific Meta-Embedding for Text Classification
Xin Wu, Yi Cai, Yang Kai, Tao Wang, Qing Li
Semantics: Lexical Semantics Short Paper
You can open the pre-recorded video in a separate window.
Abstract:
Meta-embedding learning, which combines complementary information in different word embeddings, have shown superior performances across different Natural Language Processing tasks. However, domain-specific knowledge is still ignored by existing meta-embedding methods, which results in unstable performances across specific domains. Moreover, the importance of general and domain word embeddings is related to downstream tasks, how to regularize meta-embedding to adapt downstream tasks is an unsolved problem. In this paper, we propose a method to incorporate both domain-specific and task-oriented information into meta-embeddings. We conducted extensive experiments on four text classification datasets and the results show the effectiveness of our proposed method.
NOTE: Video may display a random order of authors.
Correct author list is at the top of this page.
Connected Papers in EMNLP2020
Similar Papers
Be More with Less: Hypergraph Attention Networks for Inductive Text Classification
Kaize Ding, Jianling Wang, Jundong Li, Dingcheng Li, Huan Liu,

Multi-Stage Pre-training for Low-Resource Domain Adaptation
Rong Zhang, Revanth Gangi Reddy, Md Arafat Sultan, Vittorio Castelli, Anthony Ferritto, Radu Florian, Efsun Sarioglu Kayi, Salim Roukos, Avi Sil, Todd Ward,

PERL: Pivot-based Domain Adaptation for Pre-trained Deep Contextualized Embedding Models
Roi Reichart, Eyal Ben David, Carmel Rabinovitz,

Named Entity Recognition for Social Media Texts with Semantic Augmentation
Yuyang Nie, Yuanhe Tian, Xiang Wan, Yan Song, Bo Dai,
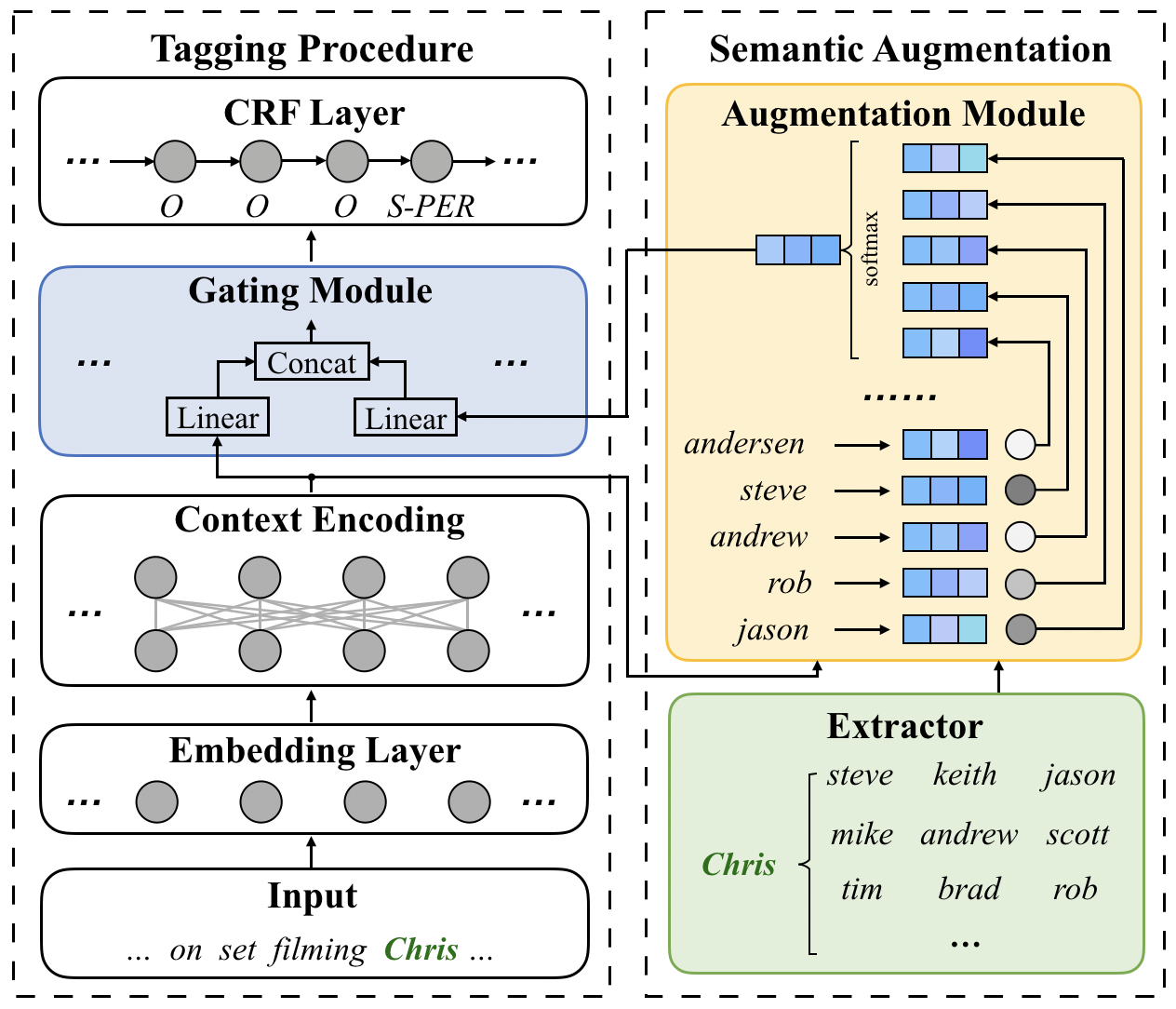