Multi-label Few/Zero-shot Learning with Knowledge Aggregated from Multiple Label Graphs
Jueqing Lu, Lan Du, Ming Liu, Joanna Dipnall
Machine Learning for NLP Short Paper
You can open the pre-recorded video in a separate window.
Abstract:
Few/Zero-shot learning is a big challenge of many classifications tasks, where a classifier is required to recognise instances of classes that have very few or even no training samples. It becomes more difficult in multi-label classification, where each instance is labelled with more than one class. In this paper, we present a simple multi-graph aggregation model that fuses knowledge from multiple label graphs encoding different semantic label relationships in order to study how the aggregated knowledge can benefit multi-label zero/few-shot document classification. The model utilises three kinds of semantic information, i.e., the pre-trained word embeddings, label description, and pre-defined label relations. Experimental results derived on two large clinical datasets (i.e., MIMIC-II and MIMIC-III ) and the EU legislation dataset show that methods equipped with the multi-graph knowledge aggregation achieve significant performance improvement across almost all the measures on few/zero-shot labels.
NOTE: Video may display a random order of authors.
Correct author list is at the top of this page.
Connected Papers in EMNLP2020
Similar Papers
Self-Supervised Meta-Learning for Few-Shot Natural Language Classification Tasks
Trapit Bansal, Rishikesh Jha, Tsendsuren Munkhdalai, Andrew McCallum,
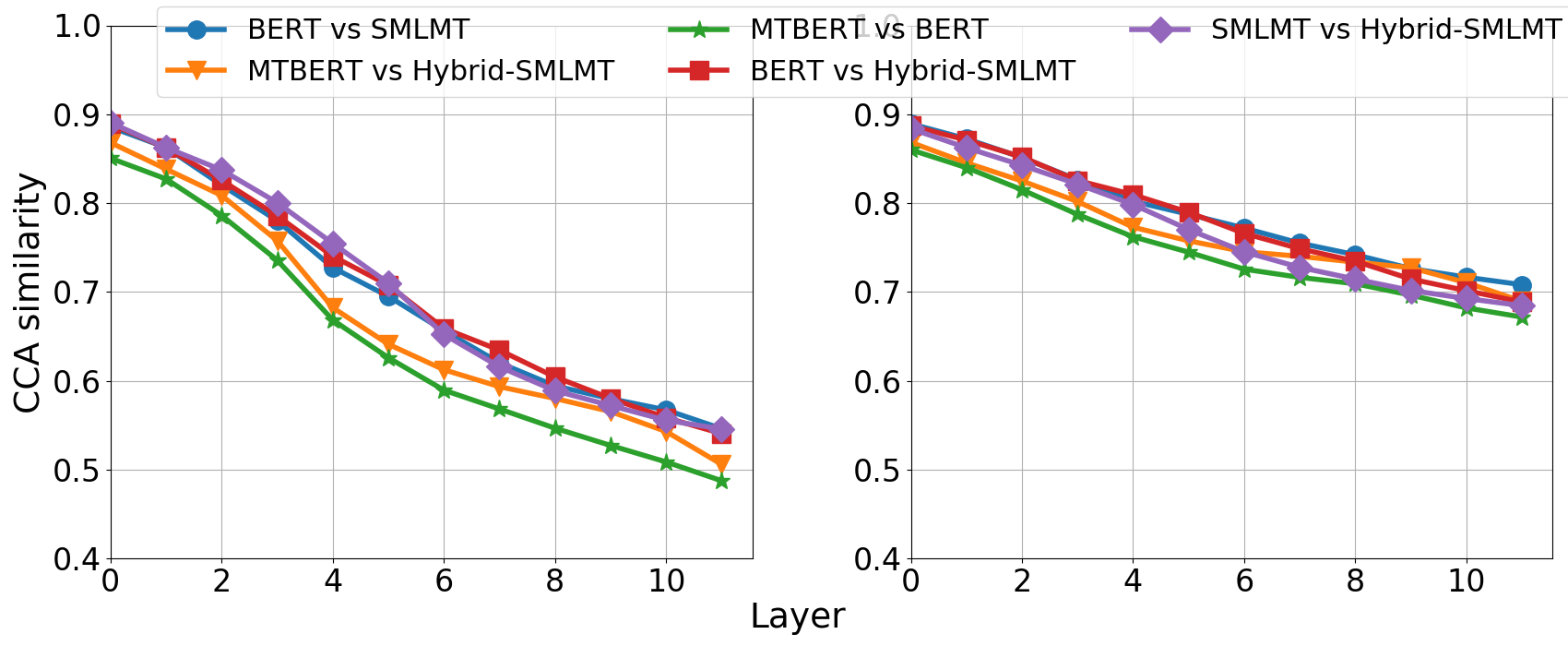
MultiCQA: Zero-Shot Transfer of Self-Supervised Text Matching Models on a Massive Scale
Andreas Rücklé, Jonas Pfeiffer, Iryna Gurevych,

Augmented Natural Language for Generative Sequence Labeling
Ben Athiwaratkun, Cicero Nogueira dos Santos, Jason Krone, Bing Xiang,

Text Classification Using Label Names Only: A Language Model Self-Training Approach
Yu Meng, Yunyi Zhang, Jiaxin Huang, Chenyan Xiong, Heng Ji, Chao Zhang, Jiawei Han,
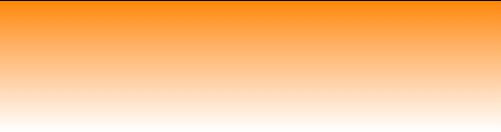