Diversified Multiple Instance Learning for Document-Level Multi-Aspect Sentiment Classification
Yunjie Ji, Hao Liu, Bolei He, Xinyan Xiao, Hua Wu, Yanhua Yu
Sentiment Analysis, Stylistic Analysis, and Argument Mining Long Paper
You can open the pre-recorded video in a separate window.
Abstract:
Neural Document-level Multi-aspect Sentiment Classification (DMSC) usually requires a lot of manual aspect-level sentiment annotations, which is time-consuming and laborious. As document-level sentiment labeled data are widely available from online service, it is valuable to perform DMSC with such free document-level annotations. To this end, we propose a novel Diversified Multiple Instance Learning Network (D-MILN), which is able to achieve aspect-level sentiment classification with only document-level weak supervision. Specifically, we connect aspect-level and document-level sentiment by formulating this problem as multiple instance learning, providing a way to learn aspect-level classifier from the back propagation of document-level supervision. Two diversified regularizations are further introduced in order to avoid the overfitting on document-level signals during training. Diversified textual regularization encourages the classifier to select aspect-relevant snippets, and diversified sentimental regularization prevents the aspect-level sentiments from being overly consistent with document-level sentiment. Experimental results on TripAdvisor and BeerAdvocate datasets show that D-MILN remarkably outperforms recent weakly-supervised baselines, and is also comparable to the supervised method.
NOTE: Video may display a random order of authors.
Correct author list is at the top of this page.
Connected Papers in EMNLP2020
Similar Papers
Multi-Instance Multi-Label Learning Networks for Aspect-Category Sentiment Analysis
Yuncong Li, Cunxiang Yin, Sheng-hua Zhong, Xu Pan,

Aspect Sentiment Classification with Aspect-Specific Opinion Spans
Lu Xu, Lidong Bing, Wei Lu, Fei Huang,
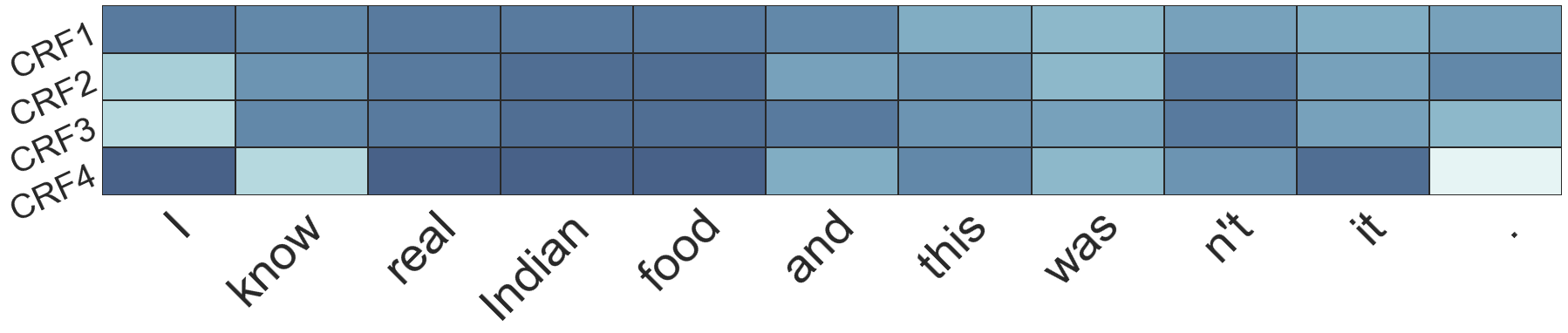
Inducing Target-Specific Latent Structures for Aspect Sentiment Classification
Chenhua Chen, Zhiyang Teng, Yue Zhang,
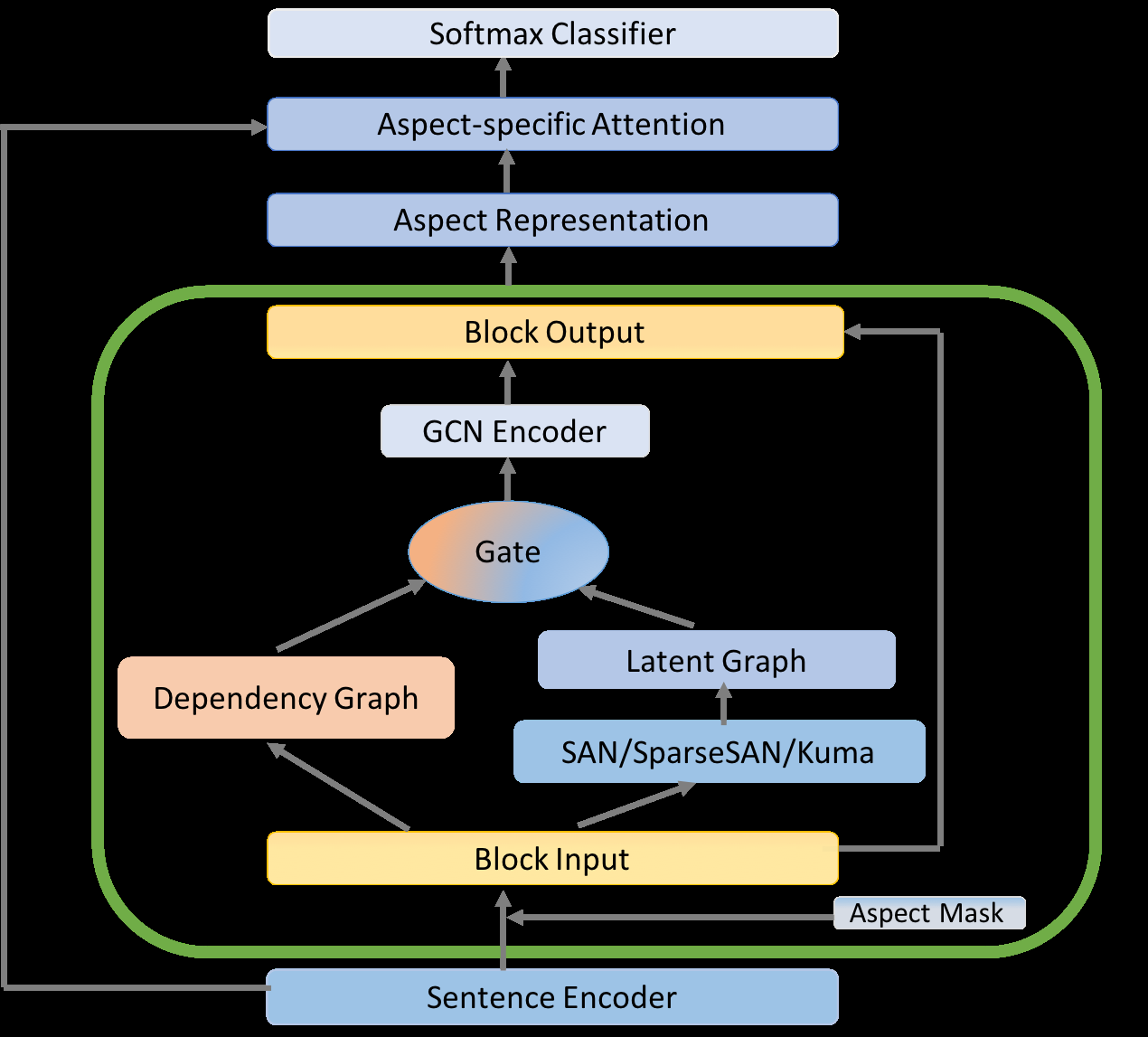