Understanding the Difficulty of Training Transformers
Liyuan Liu, Xiaodong Liu, Jianfeng Gao, Weizhu Chen, Jiawei Han
Machine Translation and Multilinguality Long Paper
You can open the pre-recorded video in a separate window.
Abstract:
Transformers have proved effective in many NLP tasks. However, their training requires non-trivial efforts regarding carefully designing cutting-edge optimizers and learning rate schedulers (e.g., conventional SGD fails to train Transformers effectively). Our objective here is to understand __what complicates Transformer training__ from both empirical and theoretical perspectives. Our analysis reveals that unbalanced gradients are not the root cause of the instability of training. Instead, we identify an amplification effect that influences training substantially—for each layer in a multi-layer Transformer model, heavy dependency on its residual branch makes training unstable, since it amplifies small parameter perturbations (e.g., parameter updates) and results in significant disturbances in the model output. Yet we observe that a light dependency limits the model potential and leads to inferior trained models. Inspired by our analysis, we propose Admin (Adaptive model initialization) to stabilize the early stage’s training and unleash its full potential in the late stage. Extensive experiments show that Admin is more stable, converges faster, and leads to better performance
NOTE: Video may display a random order of authors.
Correct author list is at the top of this page.
Connected Papers in EMNLP2020
Similar Papers
AdapterHub: A Framework for Adapting Transformers
Jonas Pfeiffer, Andreas Rücklé, Clifton Poth, Aishwarya Kamath, Ivan Vulić, Sebastian Ruder, Kyunghyun Cho, Iryna Gurevych,
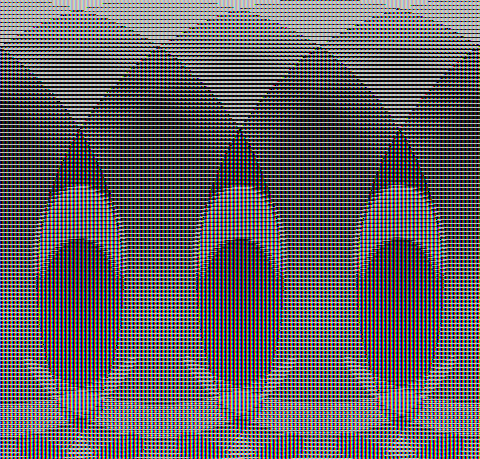
Analyzing Redundancy in Pretrained Transformer Models
Fahim Dalvi, Hassan Sajjad, Nadir Durrani, Yonatan Belinkov,
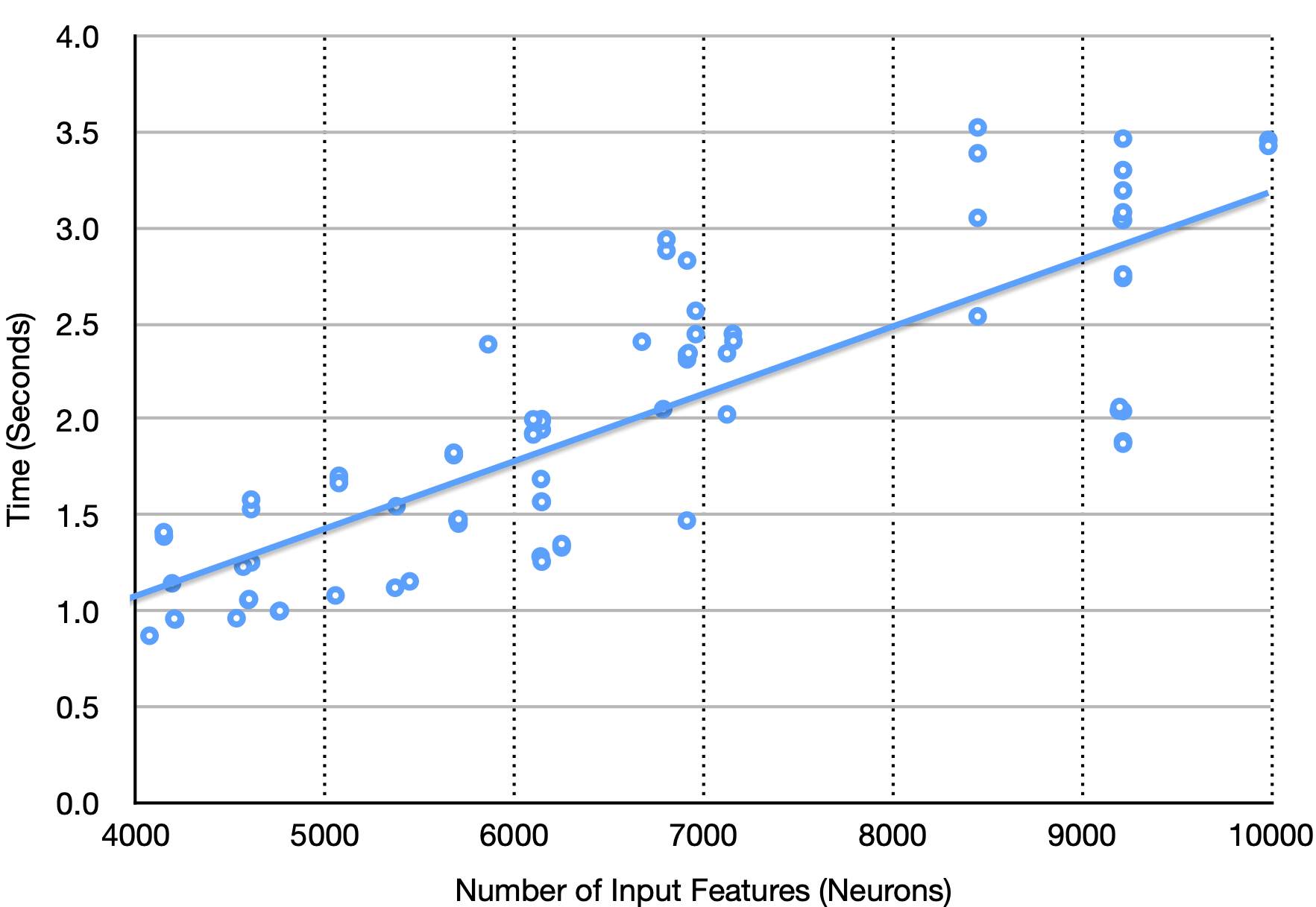
Losing Heads in the Lottery: Pruning Transformer Attention in Neural Machine Translation
Maximiliana Behnke, Kenneth Heafield,
