A Supervised Word Alignment Method based on Cross-Language Span Prediction using Multilingual BERT
Masaaki Nagata, Katsuki Chousa, Masaaki Nishino
Machine Translation and Multilinguality Long Paper
You can open the pre-recorded video in a separate window.
Abstract:
We present a novel supervised word alignment method based on cross-language span prediction. We first formalize a word alignment problem as a collection of independent predictions from a token in the source sentence to a span in the target sentence. Since this step is equivalent to a SQuAD v2.0 style question answering task, we solve it using the multilingual BERT, which is fine-tuned on manually created gold word alignment data. It is nontrivial to obtain accurate alignment from a set of independently predicted spans. We greatly improved the word alignment accuracy by adding to the question the source token's context and symmetrizing two directional predictions. In experiments using five word alignment datasets from among Chinese, Japanese, German, Romanian, French, and English, we show that our proposed method significantly outperformed previous supervised and unsupervised word alignment methods without any bitexts for pretraining. For example, we achieved 86.7 F1 score for the Chinese-English data, which is 13.3 points higher than the previous state-of-the-art supervised method.
NOTE: Video may display a random order of authors.
Correct author list is at the top of this page.
Connected Papers in EMNLP2020
Similar Papers
End-to-End Slot Alignment and Recognition for Cross-Lingual NLU
Weijia Xu, Batool Haider, Saab Mansour,

Accurate Word Alignment Induction from Neural Machine Translation
Yun Chen, Yang Liu, Guanhua Chen, Xin Jiang, Qun Liu,
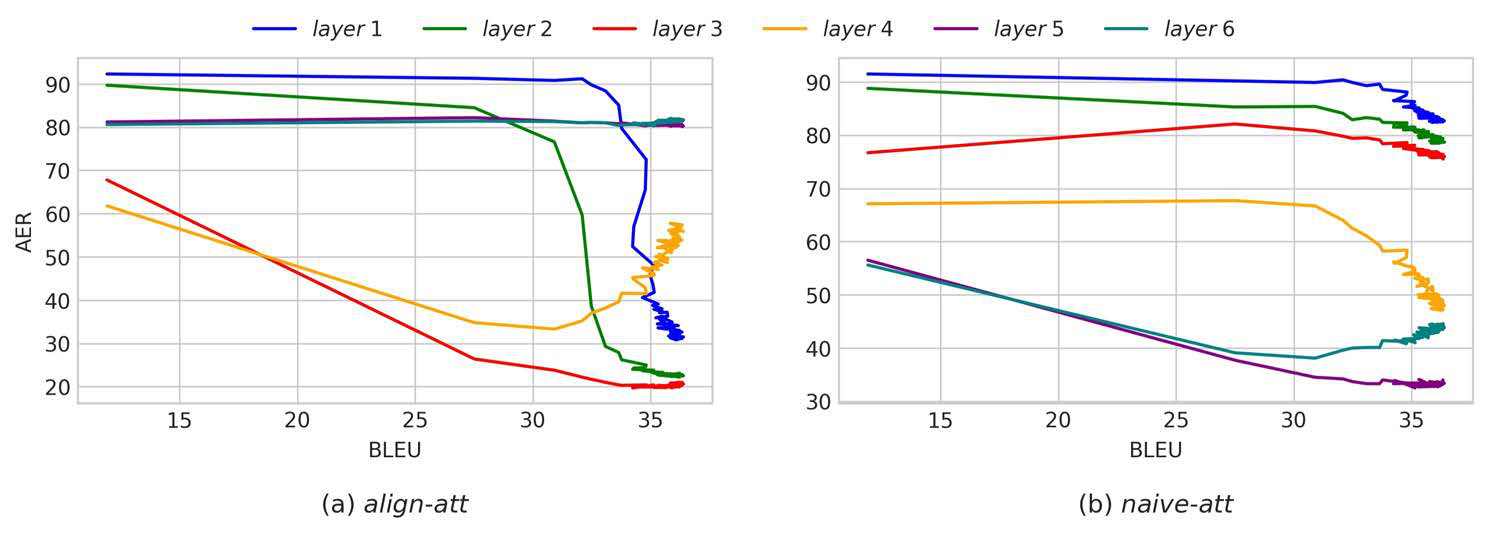
XL-WiC: A Multilingual Benchmark for Evaluating Semantic Contextualization
Alessandro Raganato, Tommaso Pasini, Jose Camacho-Collados, Mohammad Taher Pilehvar,
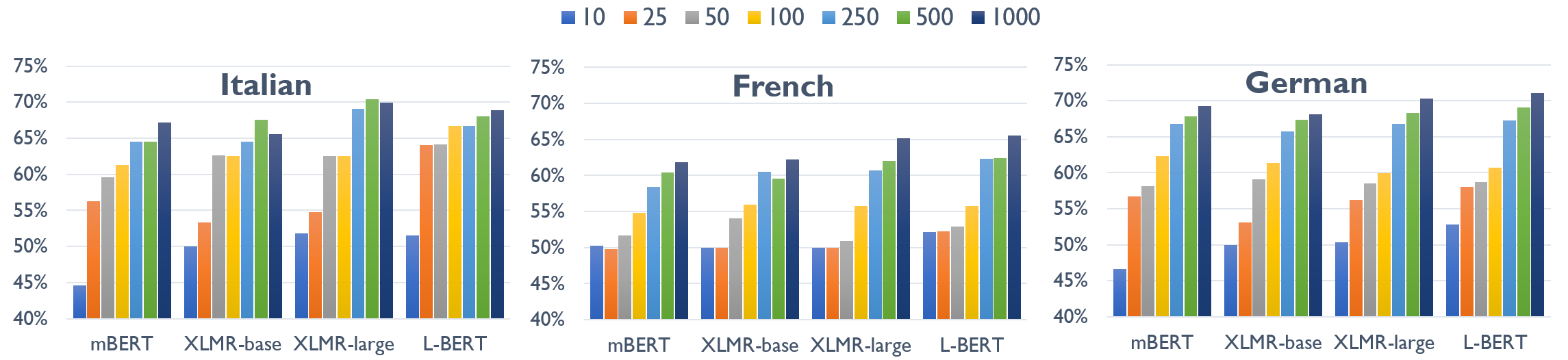