Evaluating the Calibration of Knowledge Graph Embeddings for Trustworthy Link Prediction
Tara Safavi, Danai Koutra, Edgar Meij
Information Retrieval and Text Mining Long Paper
Abstract:
Little is known about the trustworthiness of predictions made by knowledge graph embedding (KGE) models. In this paper we take initial steps toward this direction by investigating the calibration of KGE models, or the extent to which they output confidence scores that reflect the expected correctness of predicted knowledge graph triples. We first conduct an evaluation under the standard closed-world assumption (CWA), in which predicted triples not already in the knowledge graph are considered false, and show that existing calibration techniques are effective for KGE under this common but narrow assumption. Next, we introduce the more realistic but challenging open-world assumption (OWA), in which unobserved predictions are not considered true or false until ground-truth labels are obtained. Here, we show that existing calibration techniques are much less effective under the OWA than the CWA, and provide explanations for this discrepancy. Finally, to motivate the utility of calibration for KGE from a practitioner's perspective, we conduct a unique case study of human-AI collaboration, showing that calibrated predictions can improve human performance in a knowledge graph completion task.
Connected Papers in EMNLP2020
Similar Papers
Message Passing for Hyper-Relational Knowledge Graphs
Mikhail Galkin, Priyansh Trivedi, Gaurav Maheshwari, Ricardo Usbeck, Jens Lehmann,
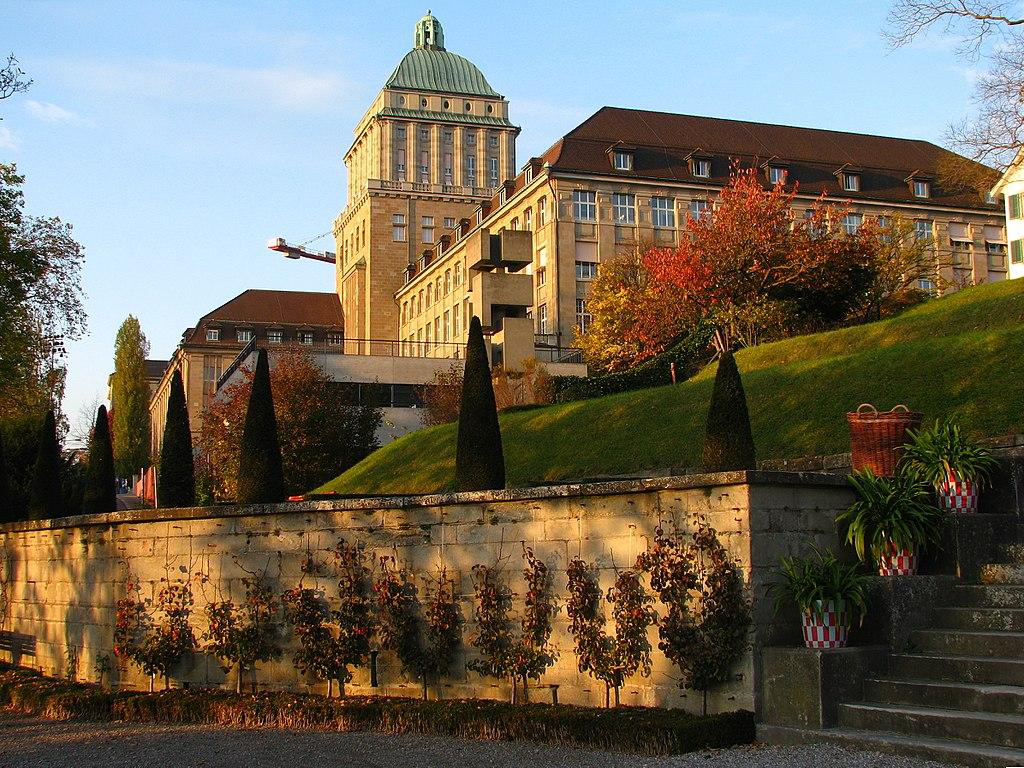
Structure Aware Negative Sampling in Knowledge Graphs
Kian Ahrabian, Aarash Feizi, Yasmin Salehi, William L. Hamilton, Avishek Joey Bose,
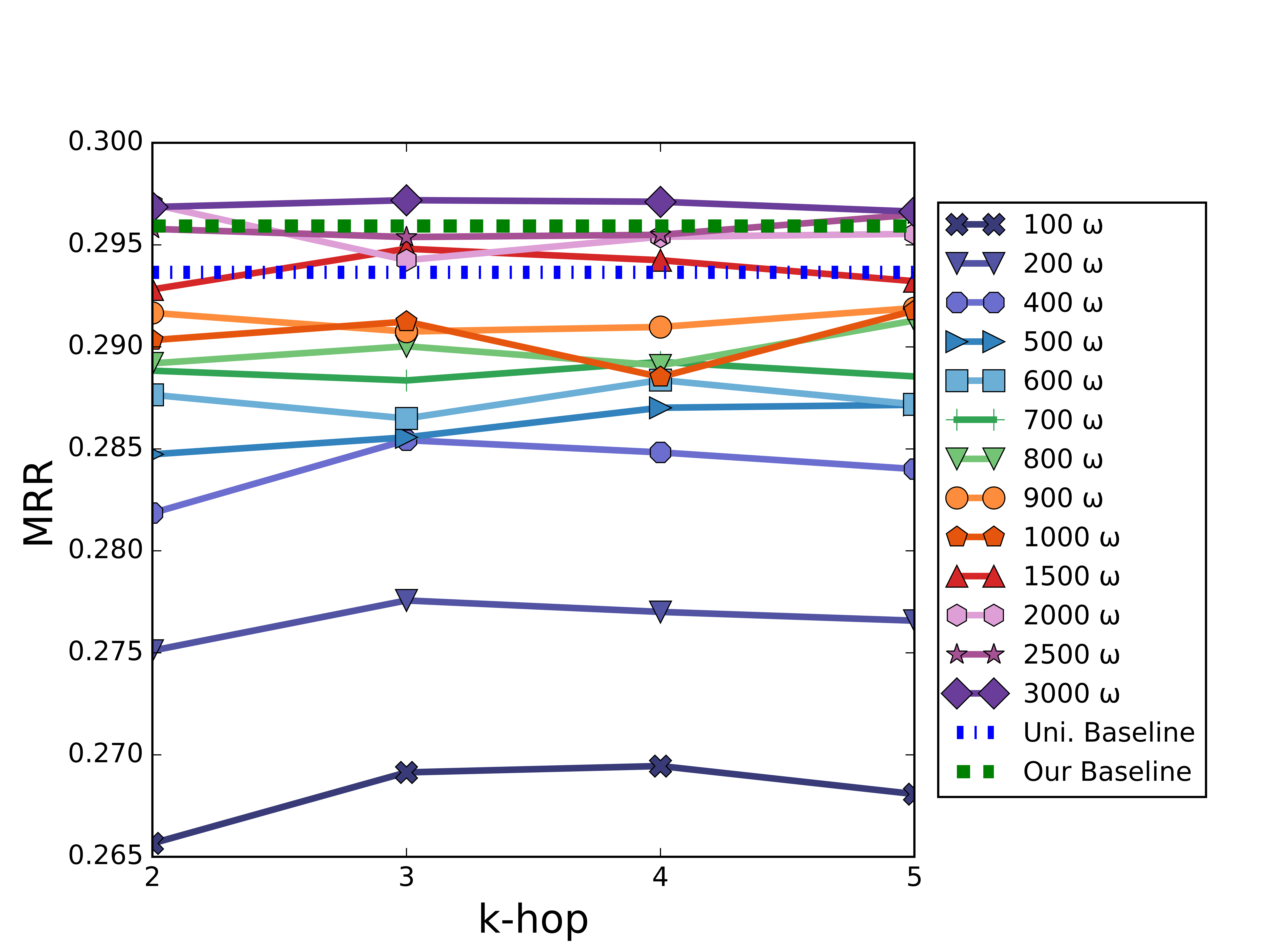
Dynamic Anticipation and Completion for Multi-Hop Reasoning over Sparse Knowledge Graph
Xin Lv, Xu Han, Lei Hou, Juanzi Li, Zhiyuan Liu, Wei Zhang, Yichi Zhang, Hao Kong, Suhui Wu,
