On Negative Interference in Multilingual Models: Findings and A Meta-Learning Treatment
Zirui Wang, Zachary C. Lipton, Yulia Tsvetkov
Machine Translation and Multilinguality Long Paper
You can open the pre-recorded video in a separate window.
Abstract:
Modern multilingual models are trained on concatenated text from multiple languages in hopes of conferring benefits to each (positive transfer), with the most pronounced benefits accruing to low-resource languages. However, recent work has shown that this approach can degrade performance on high-resource languages, a phenomenon known as negative interference. In this paper, we present the first systematic study of negative interference. We show that, contrary to previous belief, negative interference also impacts low-resource languages. While parameters are maximally shared to learn language-universal structures, we demonstrate that language-specific parameters do exist in multilingual models and they are a potential cause of negative interference. Motivated by these observations, we also present a meta-learning algorithm that obtains better cross-lingual transferability and alleviates negative interference, by adding language-specific layers as meta-parameters and training them in a manner that explicitly improves shared layers' generalization on all languages. Overall, our results show that negative interference is more common than previously known, suggesting new directions for improving multilingual representations.
NOTE: Video may display a random order of authors.
Correct author list is at the top of this page.
Connected Papers in EMNLP2020
Similar Papers
Reusing a Pretrained Language Model on Languages with Limited Corpora for Unsupervised NMT
Alexandra Chronopoulou, Dario Stojanovski, Alexander Fraser,
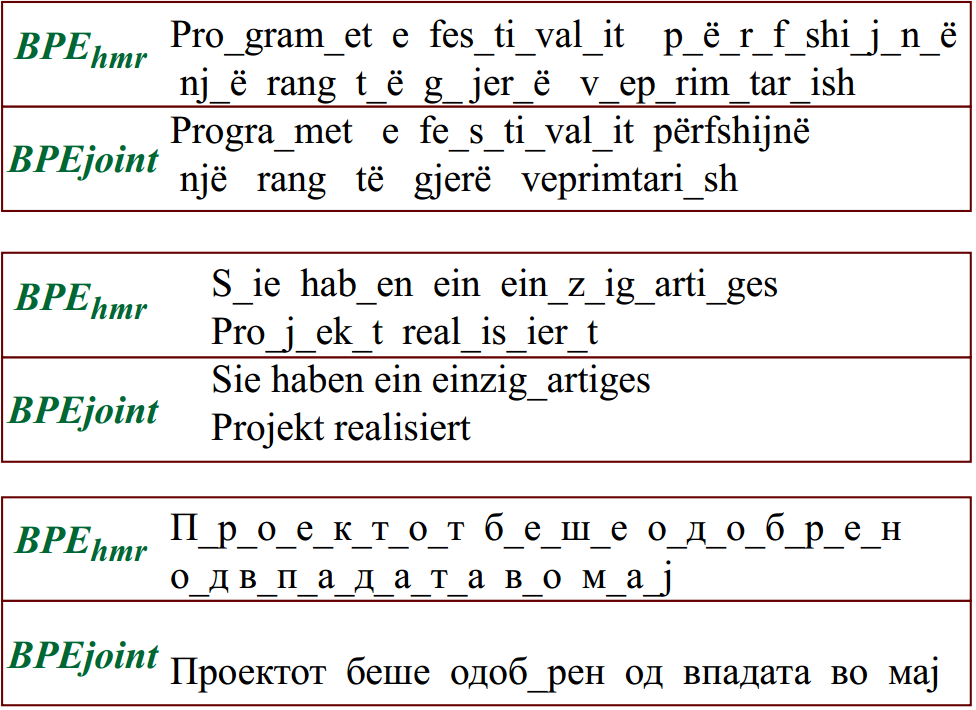
Language Model Prior for Low-Resource Neural Machine Translation
Christos Baziotis, Barry Haddow, Alexandra Birch,
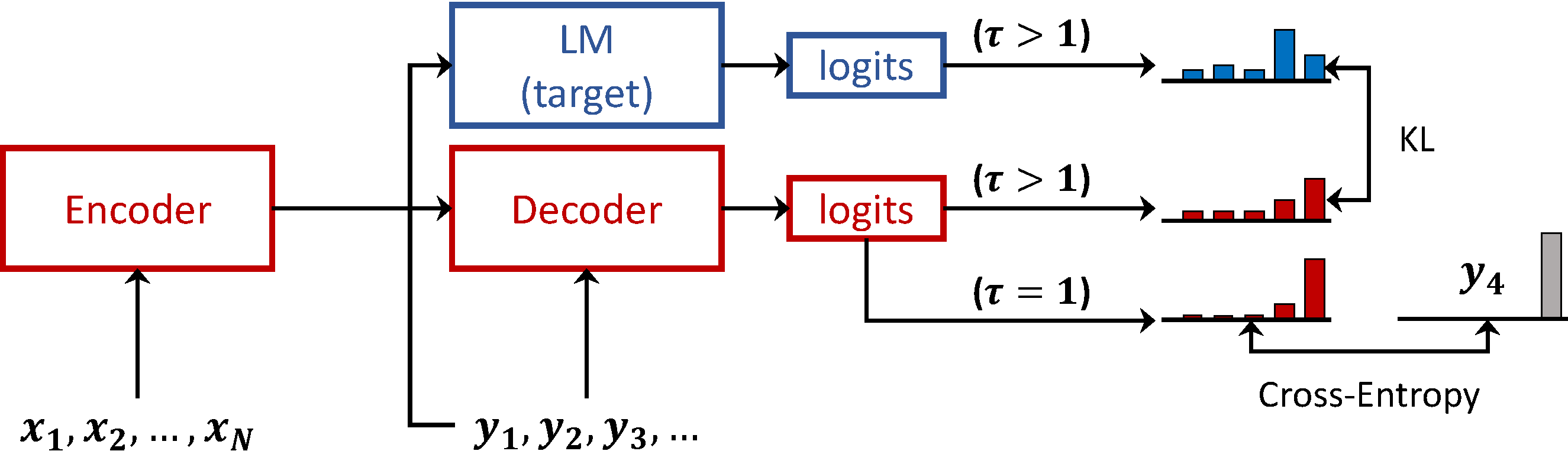
X-FACTR: Multilingual Factual Knowledge Retrieval from Pretrained Language Models
Zhengbao Jiang, Antonios Anastasopoulos, Jun Araki, Haibo Ding, Graham Neubig,

Pre-training Multilingual Neural Machine Translation by Leveraging Alignment Information
Zehui Lin, Xiao Pan, Mingxuan Wang, Xipeng Qiu, Jiangtao Feng, Hao Zhou, Lei Li,
