Identifying Elements Essential for BERT's Multilinguality
Philipp Dufter, Hinrich Schütze
Machine Translation and Multilinguality Long Paper
You can open the pre-recorded video in a separate window.
Abstract:
It has been shown that multilingual BERT (mBERT) yields high quality multilingual representations and enables effective zero-shot transfer. This is surprising given that mBERT does not use any crosslingual signal during training. While recent literature has studied this phenomenon, the reasons for the multilinguality are still somewhat obscure. We aim to identify architectural properties of BERT and linguistic properties of languages that are necessary for BERT to become multilingual. To allow for fast experimentation we propose an efficient setup with small BERT models trained on a mix of synthetic and natural data. Overall, we identify four architectural and two linguistic elements that influence multilinguality. Based on our insights, we experiment with a multilingual pretraining setup that modifies the masking strategy using VecMap, i.e., unsupervised embedding alignment. Experiments on XNLI with three languages indicate that our findings transfer from our small setup to larger scale settings.
NOTE: Video may display a random order of authors.
Correct author list is at the top of this page.
Connected Papers in EMNLP2020
Similar Papers
Revisiting Modularized Multilingual NMT to Meet Industrial Demands
Sungwon Lyu, Bokyung Son, Kichang Yang, Jaekyoung Bae,

The Thieves on Sesame Street are Polyglots - Extracting Multilingual Models from Monolingual APIs
Nitish Shirish Keskar, Bryan McCann, Caiming Xiong, Richard Socher,
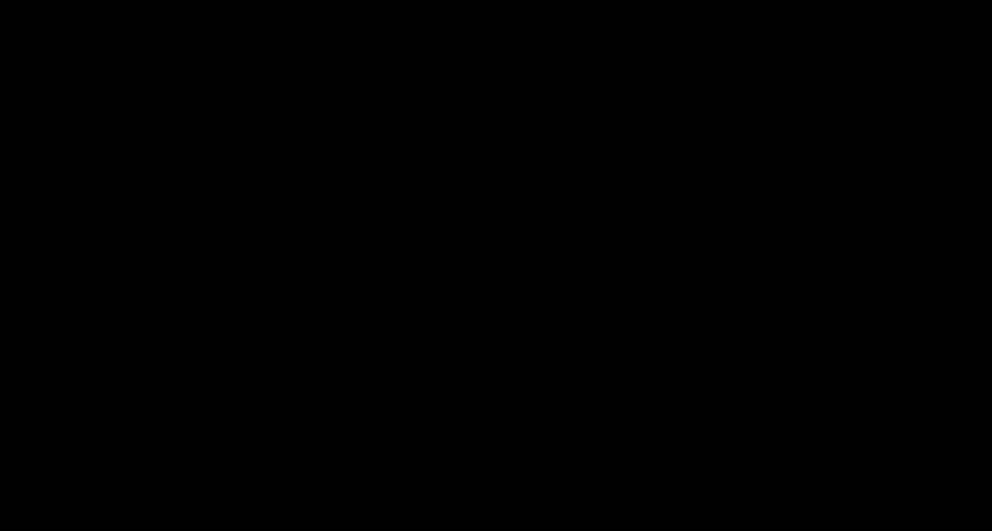
Improving Multilingual Models with Language-Clustered Vocabularies
Hyung Won Chung, Dan Garrette, Kiat Chuan Tan, Jason Riesa,

Multilingual Denoising Pre-training for Neural Machine Translation
Jiatao Gu, Yinhan Liu, Naman Goyal, Xian Li, Sergey Edunov, Marjan Ghazvininejad, Mike Lewis, Luke Zettlemoyer,
