Mind Your Inflections! Improving NLP for Non-Standard Englishes with Base-Inflection Encoding
Samson Tan, Shafiq Joty, Lav Varshney, Min-Yen Kan
Phonology, Morphology and Word Segmentation Long Paper
You can open the pre-recorded video in a separate window.
Abstract:
Inflectional variation is a common feature of World Englishes such as Colloquial Singapore English and African American Vernacular English. Although comprehension by human readers is usually unimpaired by non-standard inflections, current NLP systems are not yet robust. We propose Base-Inflection Encoding (BITE), a method to tokenize English text by reducing inflected words to their base forms before reinjecting the grammatical information as special symbols. Fine-tuning pretrained NLP models for downstream tasks using our encoding defends against inflectional adversaries while maintaining performance on clean data. Models using BITE generalize better to dialects with non-standard inflections without explicit training and translation models converge faster when trained with BITE. Finally, we show that our encoding improves the vocabulary efficiency of popular data-driven subword tokenizers. Since there has been no prior work on quantitatively evaluating vocabulary efficiency, we propose metrics to do so.
NOTE: Video may display a random order of authors.
Correct author list is at the top of this page.
Connected Papers in EMNLP2020
Similar Papers
IGT2P: From Interlinear Glossed Texts to Paradigms
Sarah Moeller, Ling Liu, Changbing Yang, Katharina Kann, Mans Hulden,
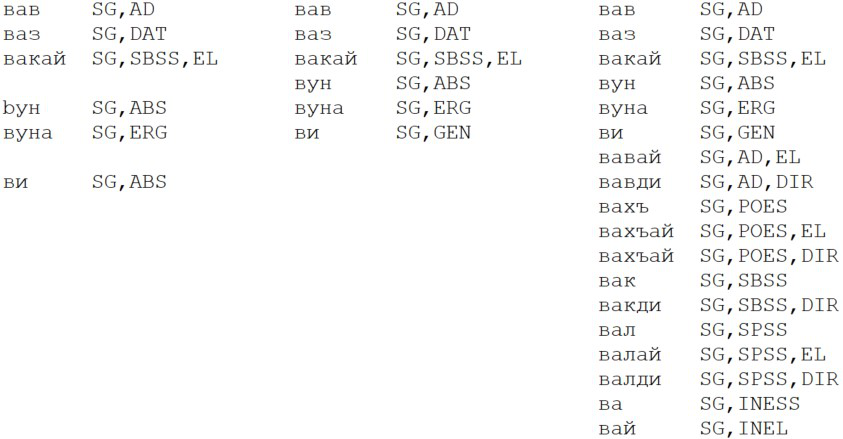
Towards Enhancing Faithfulness for Neural Machine Translation
Rongxiang Weng, Heng Yu, Xiangpeng Wei, Weihua Luo,
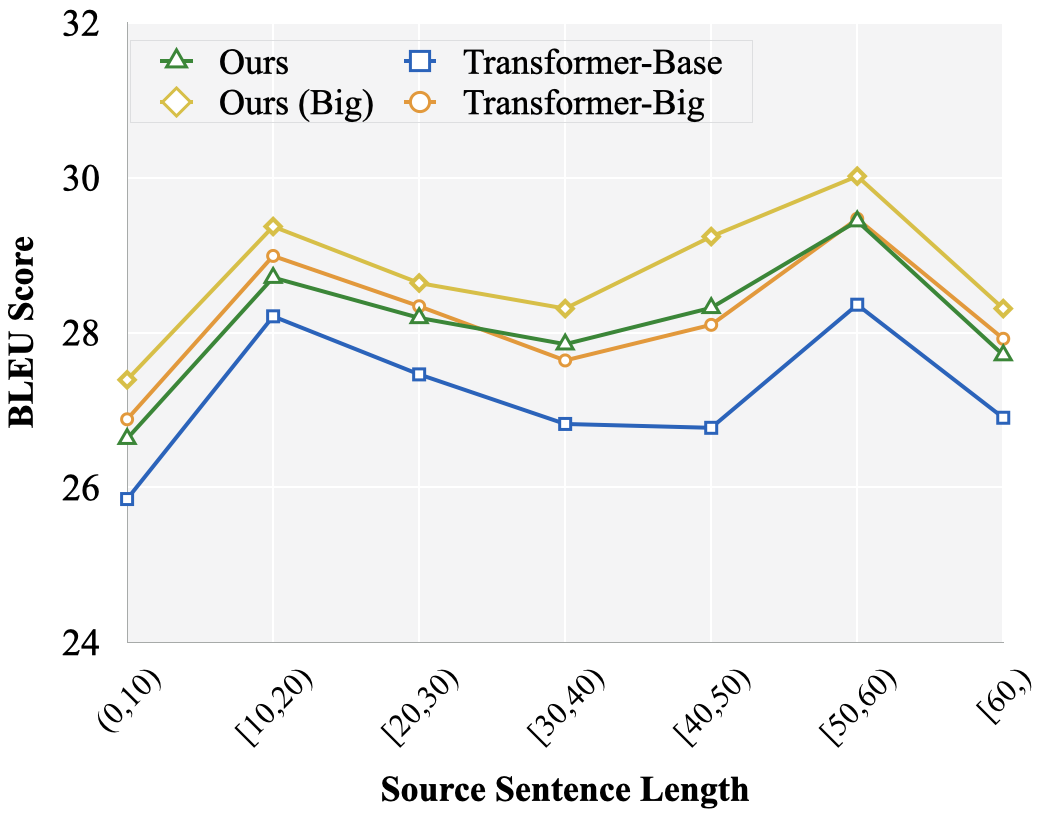
Generationary or “How We Went beyond Word Sense Inventories and Learned to Gloss”
Michele Bevilacqua, Marco Maru, Roberto Navigli,
