Masking as an Efficient Alternative to Finetuning for Pretrained Language Models
Mengjie Zhao, Tao Lin, Fei Mi, Martin Jaggi, Hinrich Schütze
Machine Learning for NLP Long Paper
You can open the pre-recorded video in a separate window.
Abstract:
We present an efficient method of utilizing pretrained language models, where we learn selective binary masks for pretrained weights in lieu of modifying them through finetuning. Extensive evaluations of masking BERT, RoBERTa, and DistilBERT on eleven diverse NLP tasks show that our masking scheme yields performance comparable to finetuning, yet has a much smaller memory footprint when several tasks need to be inferred. Intrinsic evaluations show that representations computed by our binary masked language models encode information necessary for solving downstream tasks. Analyzing the loss landscape, we show that masking and finetuning produce models that reside in minima that can be connected by a line segment with nearly constant test accuracy. This confirms that masking can be utilized as an efficient alternative to finetuning.
NOTE: Video may display a random order of authors.
Correct author list is at the top of this page.
Connected Papers in EMNLP2020
Similar Papers
Neural Mask Generator: Learning to Generate Adaptive Word Maskings for Language Model Adaptation
Minki Kang, Moonsu Han, Sung Ju Hwang,
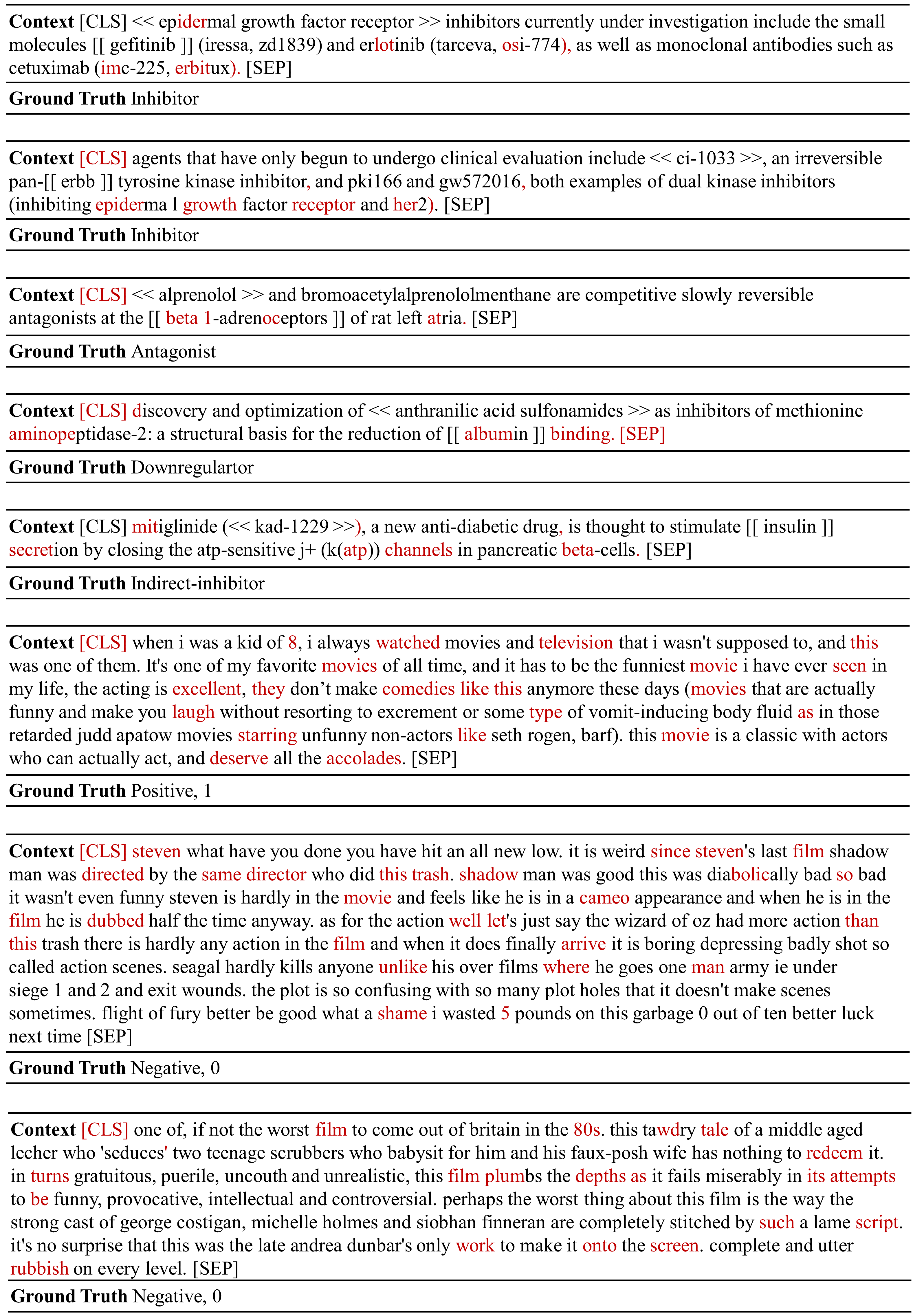
NeuSpell: A Neural Spelling Correction Toolkit
Sai Muralidhar Jayanthi, Danish Pruthi, Graham Neubig,
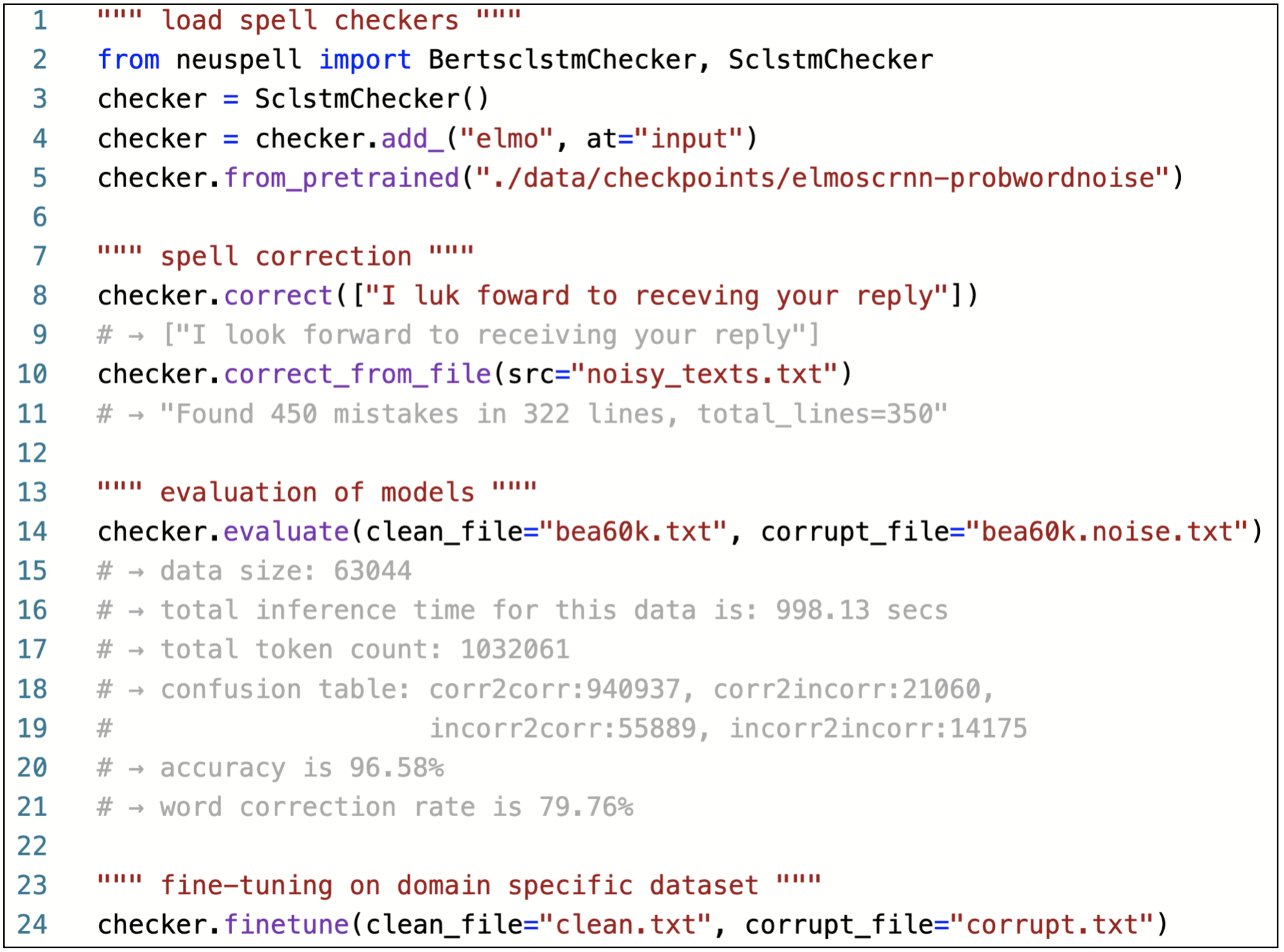
Train No Evil: Selective Masking for Task-Guided Pre-Training
Yuxian Gu, Zhengyan Zhang, Xiaozhi Wang, Zhiyuan Liu, Maosong Sun,
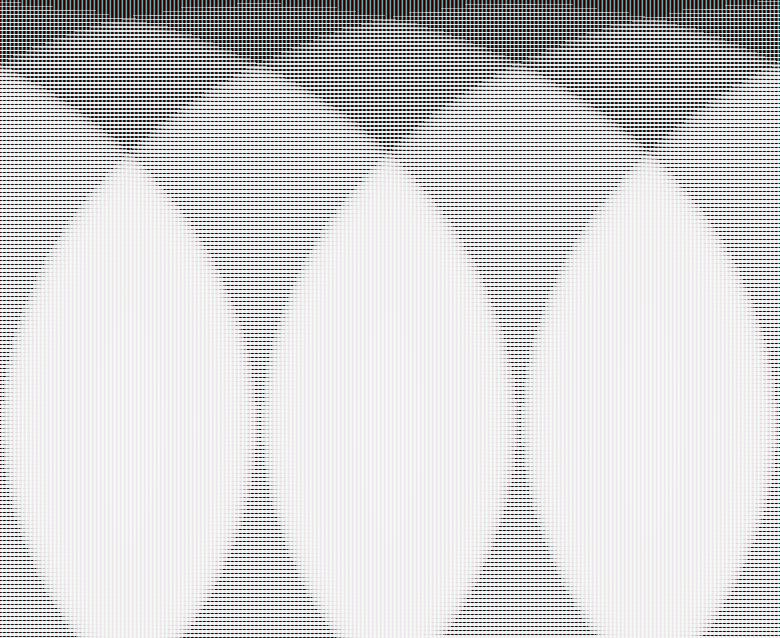