Towards Medical Machine Reading Comprehension with Structural Knowledge and Plain Text
Dongfang Li, Baotian Hu, Qingcai Chen, Weihua Peng, Anqi Wang
NLP Applications Long Paper
Abstract:
Machine reading comprehension (MRC) has achieved significant progress on the open domain in recent years, mainly due to large-scale pre-trained language models. However, it performs much worse in specific domains such as the medical field due to the lack of extensive training data and professional structural knowledge neglect. As an effort, we first collect a large scale medical multi-choice question dataset (more than 21k instances) for the National Licensed Pharmacist Examination in China. It is a challenging medical examination with a passing rate of less than 14.2% in 2018. Then we propose a novel reading comprehension model KMQA, which can fully exploit the structural medical knowledge (i.e., medical knowledge graph) and the reference medical plain text (i.e., text snippets retrieved from reference books). The experimental results indicate that the KMQA outperforms existing competitive models with a large margin and passes the exam with 61.8% accuracy rate on the test set.
Connected Papers in EMNLP2020
Similar Papers
New Protocols and Negative Results for Textual Entailment Data Collection
Samuel R. Bowman, Jennimaria Palomaki, Livio Baldini Soares, Emily Pitler,

X-FACTR: Multilingual Factual Knowledge Retrieval from Pretrained Language Models
Zhengbao Jiang, Antonios Anastasopoulos, Jun Araki, Haibo Ding, Graham Neubig,

COMETA: A Corpus for Medical Entity Linking in the Social Media
Marco Basaldella, Fangyu Liu, Ehsan Shareghi, Nigel Collier,

Learning from Context or Names? An Empirical Study on Neural Relation Extraction
Hao Peng, Tianyu Gao, Xu Han, Yankai Lin, Peng Li, Zhiyuan Liu, Maosong Sun, Jie Zhou,
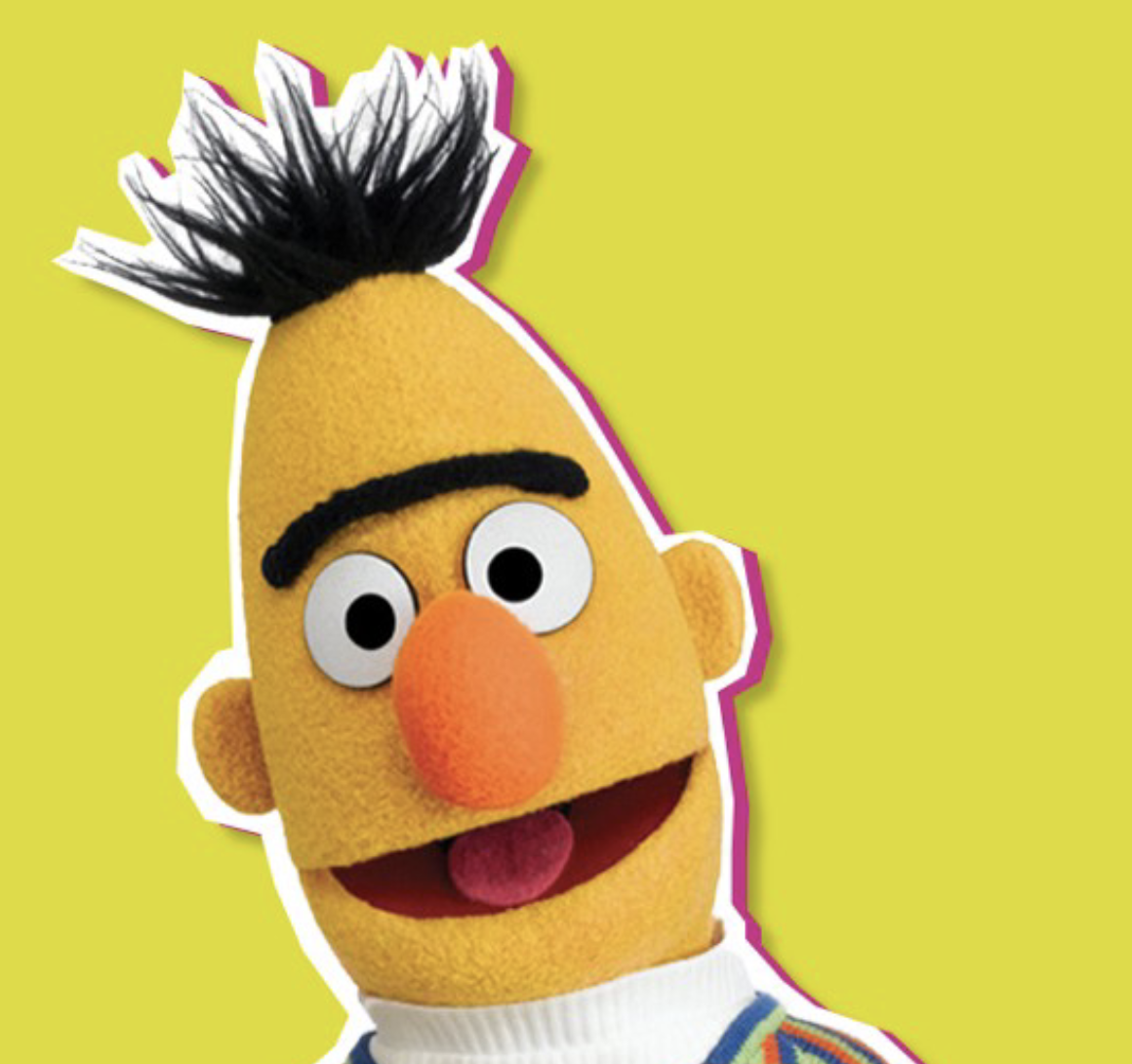