Unsupervised Discovery of Implicit Gender Bias
Anjalie Field, Yulia Tsvetkov
Computational Social Science and Social Media Long Paper
You can open the pre-recorded video in a separate window.
Abstract:
Despite their prevalence in society, social biases are difficult to identify, primarily because human judgements in this domain can be unreliable. We take an unsupervised approach to identifying gender bias against women at a comment level and present a model that can surface text likely to contain bias. Our main challenge is forcing the model to focus on signs of implicit bias, rather than other artifacts in the data. Thus, our methodology involves reducing the influence of confounds through propensity matching and adversarial learning. Our analysis shows how biased comments directed towards female politicians contain mixed criticisms, while comments directed towards other female public figures focus on appearance and sexualization. Ultimately, our work offers a way to capture subtle biases in various domains without relying on subjective human judgements.
NOTE: Video may display a random order of authors.
Correct author list is at the top of this page.
Connected Papers in EMNLP2020
Similar Papers
Multi-Dimensional Gender Bias Classification
Emily Dinan, Angela Fan, Ledell Wu, Jason Weston, Douwe Kiela, Adina Williams,
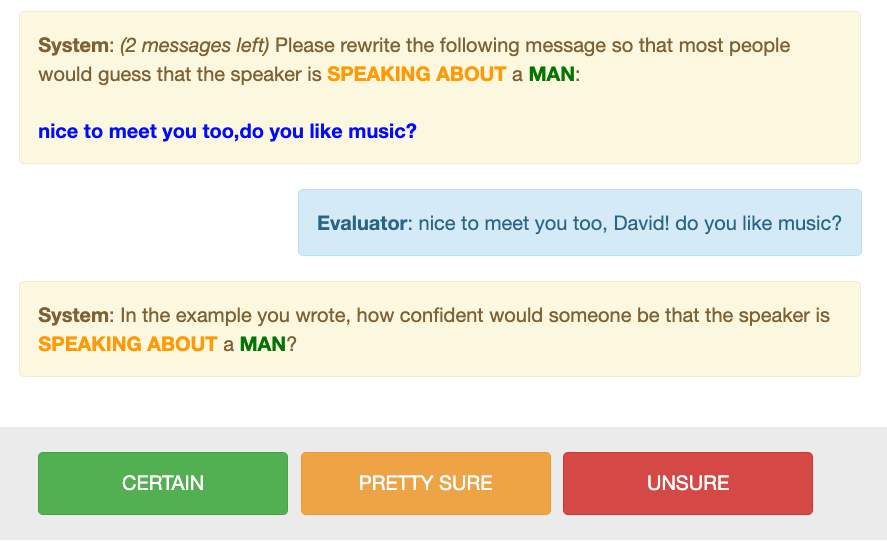
Joint Estimation and Analysis of Risk Behavior Ratings in Movie Scripts
Victor Martinez, Krishna Somandepalli, Yalda Tehranian-Uhls, Shrikanth Narayanan,
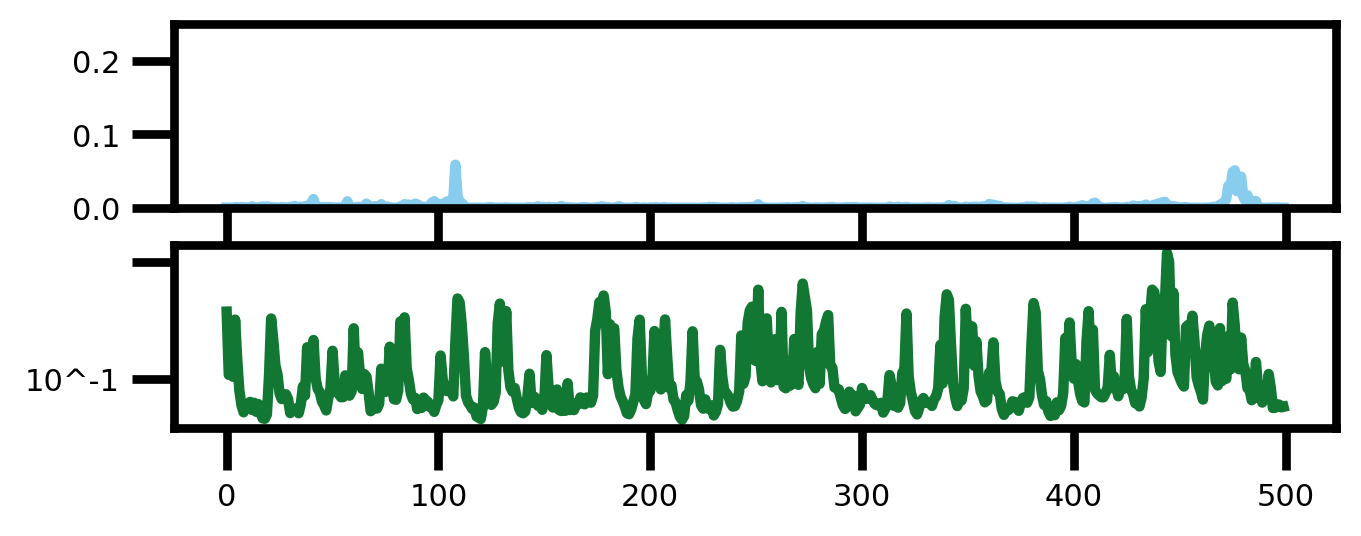
On the Reliability and Validity of Detecting Approval of Political Actors in Tweets
Indira Sen, Fabian Flöck, Claudia Wagner,
