Social Media Attributions in the Context of Water Crisis
Rupak Sarkar, Sayantan Mahinder, Hirak Sarkar, Ashiqur KhudaBukhsh
Computational Social Science and Social Media Long Paper
You can open the pre-recorded video in a separate window.
Abstract:
Attribution of natural disasters/collective misfortune is a widely-studied political science problem. However, such studies typically rely on surveys, or expert opinions, or external signals such as voting outcomes. In this paper, we explore the viability of using unstructured, noisy social media data to complement traditional surveys through automatically extracting attribution factors. We present a novel prediction task of \emph{attribution tie detection} of identifying the factors (e.g., poor city planning, exploding population etc.) held responsible for the crisis in a social media document. We focus on the 2019 Chennai water crisis that rapidly escalated into a discussion topic with global importance following alarming water-crisis statistics. On a challenging data set constructed from YouTube comments (72,098 comments posted by 43,859 users on 623 videos relevant to the crisis), we present a neural baseline to identify attribution ties that achieves a reasonable performance (accuracy: 87.34\% on attribution detection and 81.37\% on attribution resolution). We release the first annotated data set of 2,500 comments in this important domain.
NOTE: Video may display a random order of authors.
Correct author list is at the top of this page.
Connected Papers in EMNLP2020
Similar Papers
Unsupervised stance detection for arguments from consequences
Jonathan Kobbe, Ioana Hulpus, Heiner Stuckenschmidt,

We Can Detect Your Bias: Predicting the Political Ideology of News Articles
Ramy Baly, Giovanni Da San Martino, James Glass, Preslav Nakov,
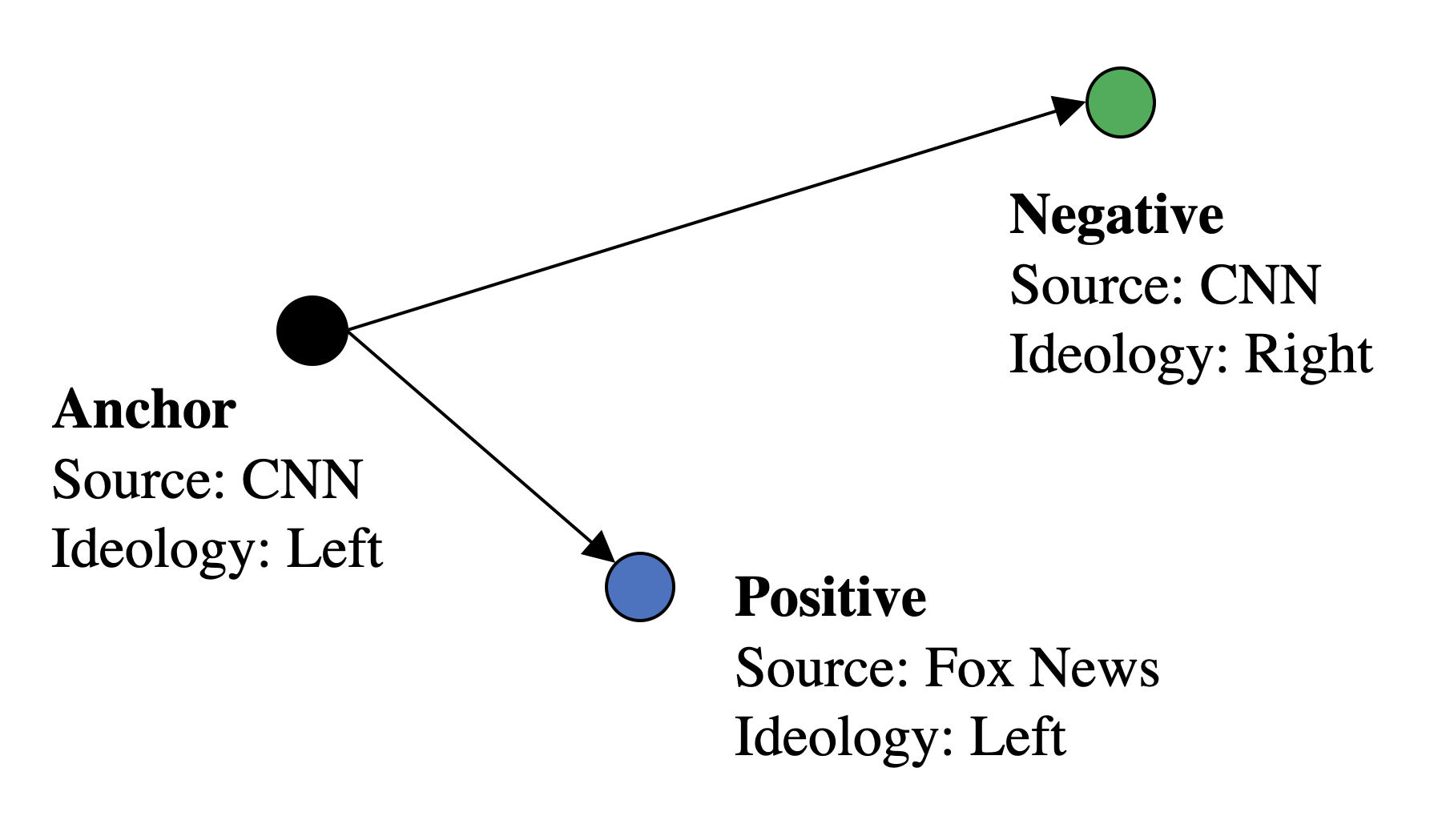
Weakly Supervised Learning of Nuanced Frames for Analyzing Polarization in News Media
Shamik Roy, Dan Goldwasser,
