Inference Strategies for Machine Translation with Conditional Masking
Julia Kreutzer, George Foster, Colin Cherry
Machine Translation and Multilinguality Short Paper
You can open the pre-recorded video in a separate window.
Abstract:
Conditional masked language model (CMLM) training has proven successful for non-autoregressive and semi-autoregressive sequence generation tasks, such as machine translation. Given a trained CMLM, however, it is not clear what the best inference strategy is. We formulate masked inference as a factorization of conditional probabilities of partial sequences, show that this does not harm performance, and investigate a number of simple heuristics motivated by this perspective. We identify a thresholding strategy that has advantages over the standard ``mask-predict'' algorithm, and provide analyses of its behavior on machine translation tasks.
NOTE: Video may display a random order of authors.
Correct author list is at the top of this page.
Connected Papers in EMNLP2020
Similar Papers
Consistency of a Recurrent Language Model With Respect to Incomplete Decoding
Sean Welleck, Ilia Kulikov, Jaedeok Kim, Richard Yuanzhe Pang, Kyunghyun Cho,
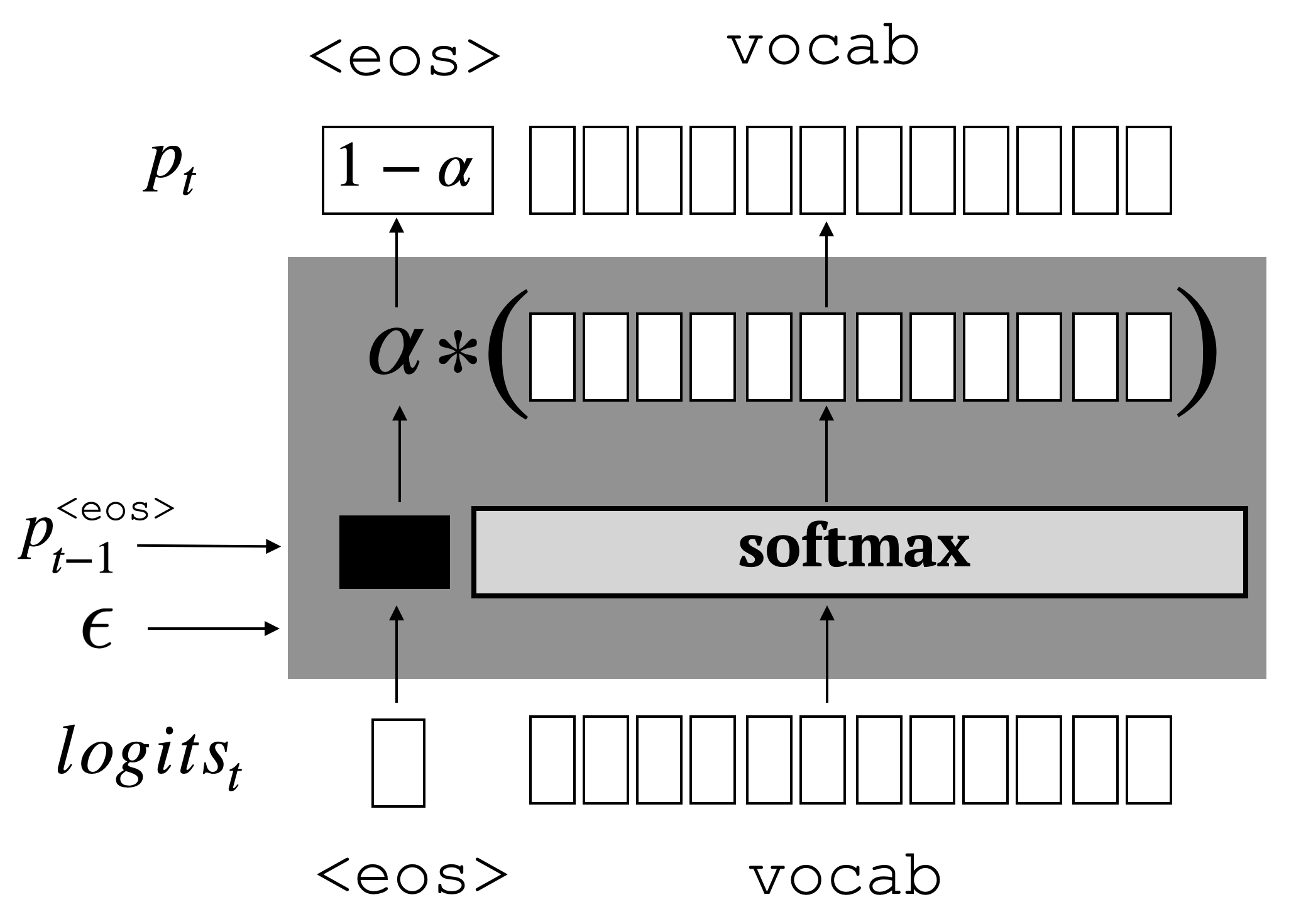
Neural Mask Generator: Learning to Generate Adaptive Word Maskings for Language Model Adaptation
Minki Kang, Moonsu Han, Sung Ju Hwang,
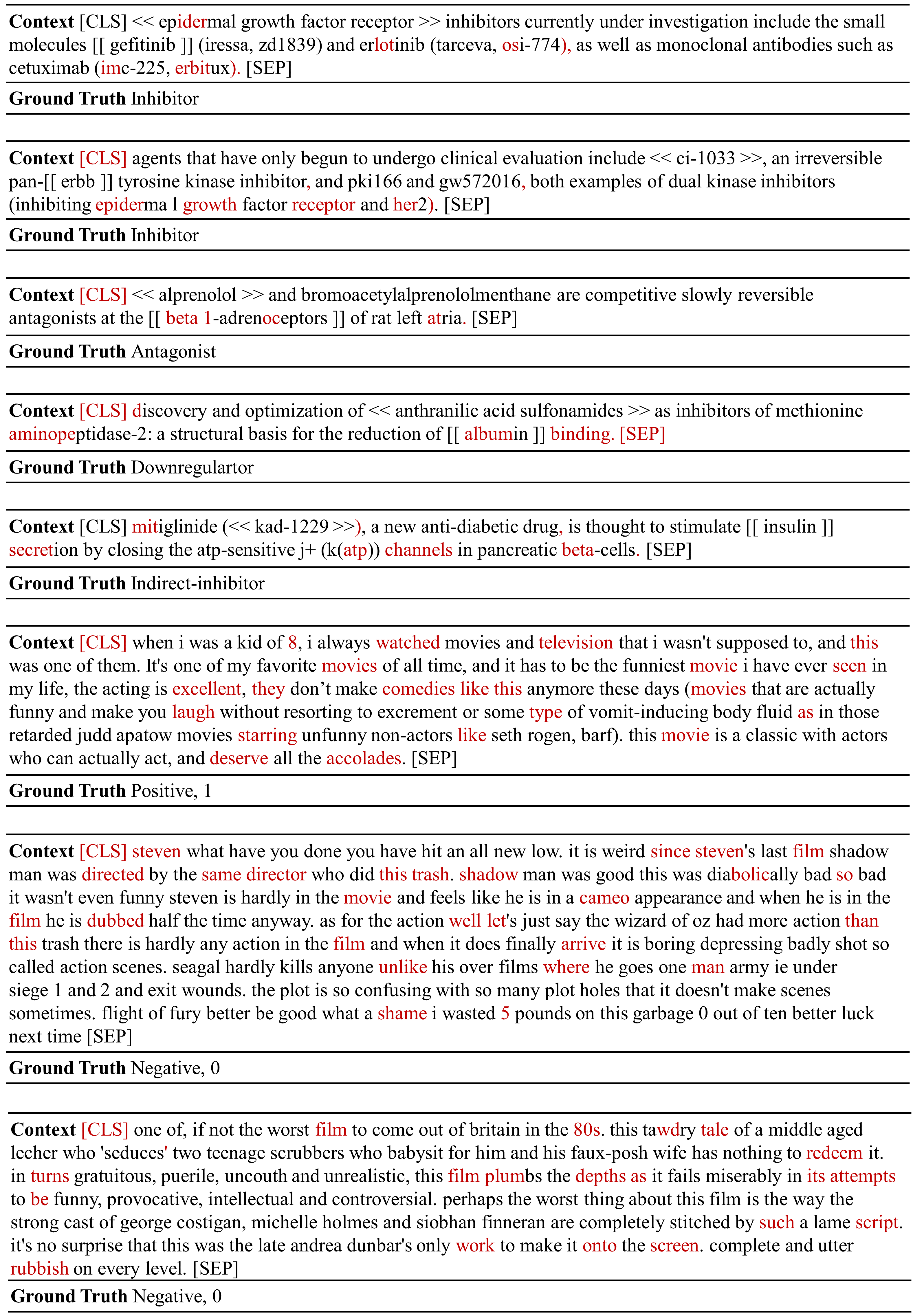
PALM: Pre-training an Autoencoding&Autoregressive Language Model for Context-conditioned Generation
Bin Bi, Chenliang Li, Chen Wu, Ming Yan, Wei Wang, Songfang Huang, Fei Huang, Luo Si,
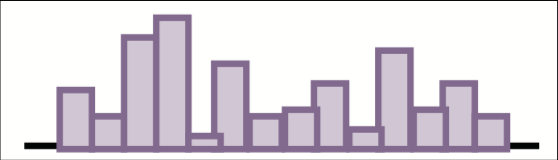