Deep Attentive Learning for Stock Movement Prediction From Social Media Text and Company Correlations
Ramit Sawhney, Shivam Agarwal, Arnav Wadhwa, Rajiv Ratn Shah
NLP Applications Long Paper
Abstract:
In the financial domain, risk modeling and profit generation heavily rely on the sophisticated and intricate stock movement prediction task. Stock forecasting is complex, given the stochastic dynamics and non-stationary behavior of the market. Stock movements are influenced by varied factors beyond the conventionally studied historical prices, such as social media and correlations among stocks. The rising ubiquity of online content and knowledge mandates an exploration of models that factor in such multimodal signals for accurate stock forecasting. We introduce an architecture that achieves a potent blend of chaotic temporal signals from financial data, social media, and inter-stock relationships via a graph neural network in a hierarchical temporal fashion. Through experiments on real-world S\&P 500 index data and English tweets, we show the practical applicability of our model as a tool for investment decision making and trading.
Connected Papers in EMNLP2020
Similar Papers
Public Sentiment Drift Analysis Based on Hierarchical Variational Auto-encoder
Wenyue Zhang, Xiaoli Li, Yang Li, Suge Wang, Deyu Li, Jian Liao, Jianxing Zheng,
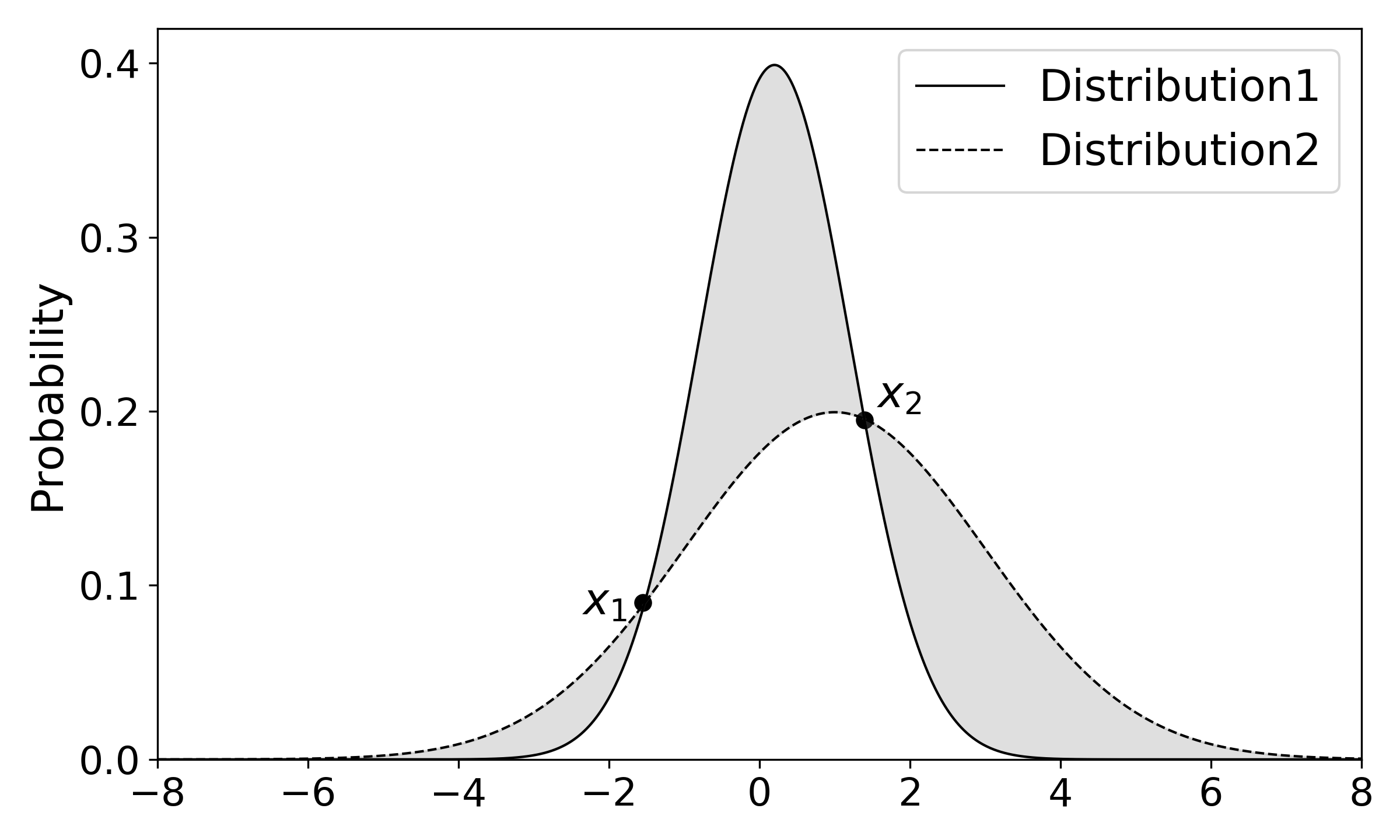
An Empirical Investigation of Contextualized Number Prediction
Taylor Berg-Kirkpatrick, Daniel Spokoyny,
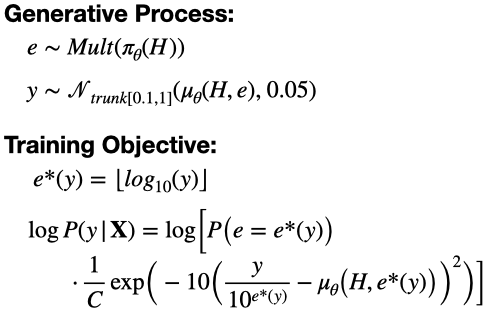
We Can Detect Your Bias: Predicting the Political Ideology of News Articles
Ramy Baly, Giovanni Da San Martino, James Glass, Preslav Nakov,
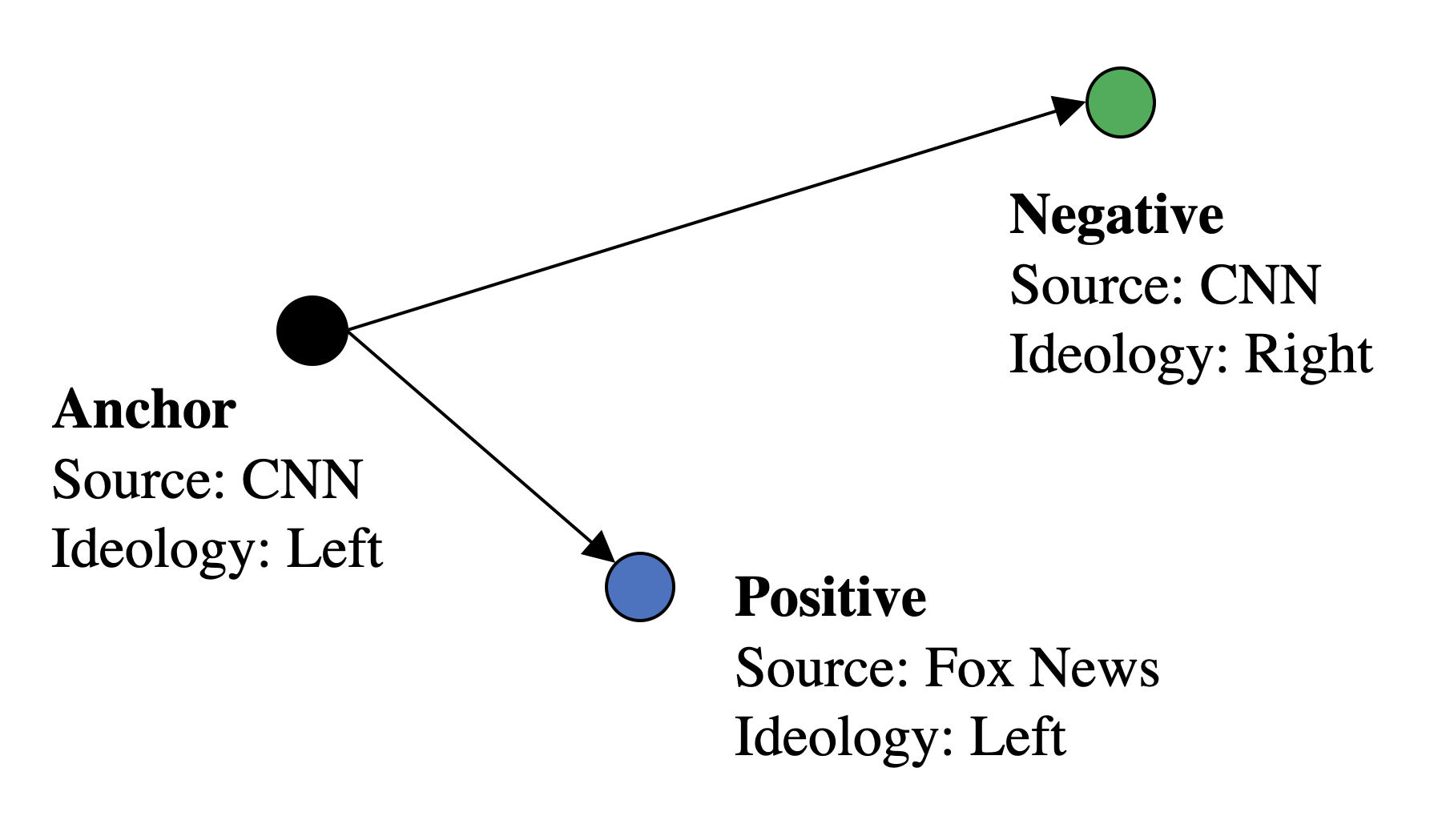
Hashtags, Emotions, and Comments: A Large-Scale Dataset to Understand Fine-Grained Social Emotions to Online Topics
Keyang Ding, Jing Li, Yuji Zhang,
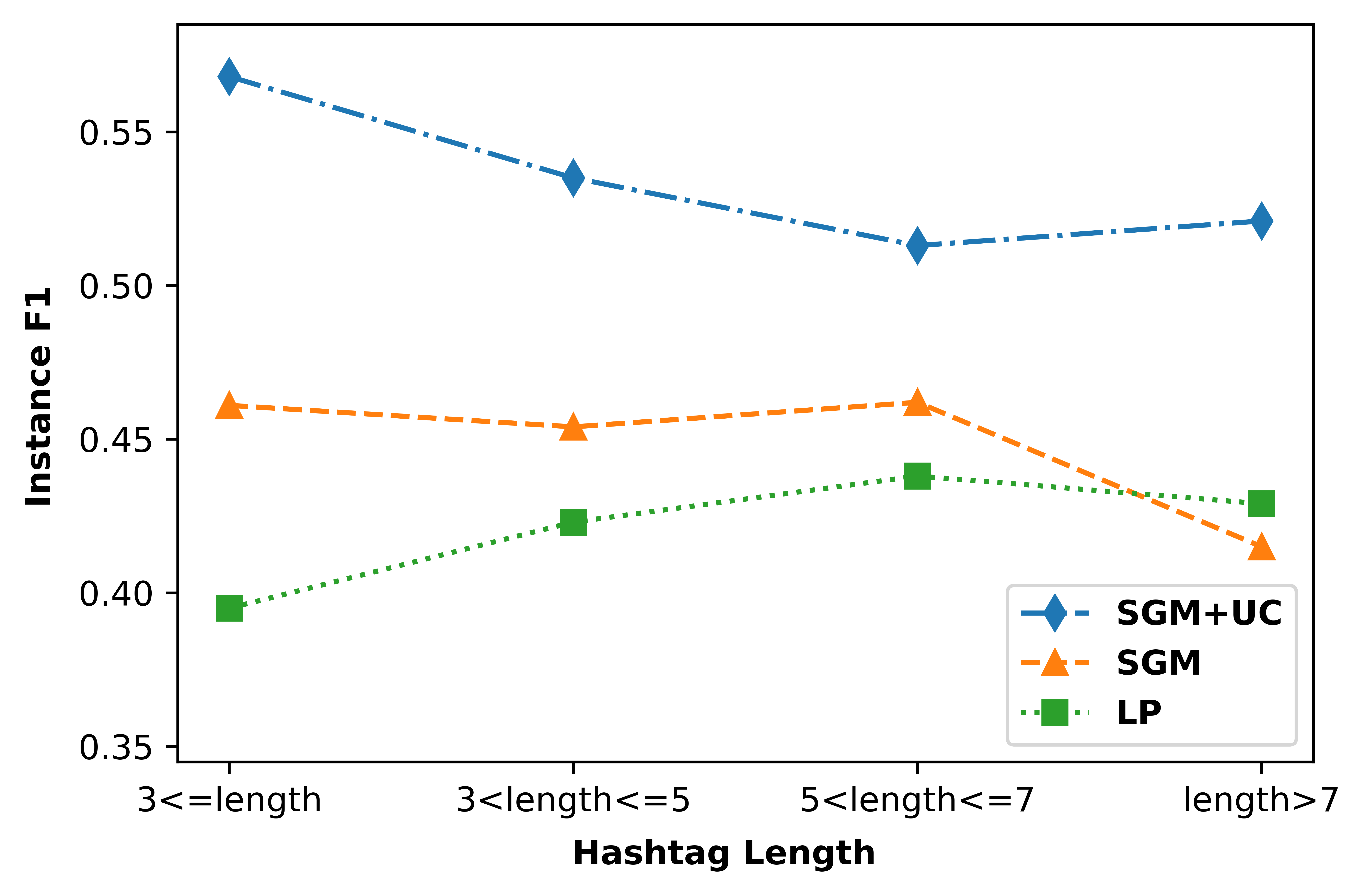