Entities as Experts: Sparse Memory Access with Entity Supervision
Thibault Févry, Livio Baldini Soares, Nicholas FitzGerald, Eunsol Choi, Tom Kwiatkowski
Machine Learning for NLP Long Paper
You can open the pre-recorded video in a separate window.
Abstract:
We focus on the problem of capturing declarative knowledge about entities in the learned parameters of a language model. We introduce a new model---Entities as Experts (EaE)---that can access distinct memories of the entities mentioned in a piece of text. Unlike previous efforts to integrate entity knowledge into sequence models, EaE's entity representations are learned directly from text. We show that EaE's learned representations capture sufficient knowledge to answer TriviaQA questions such as "Which Dr. Who villain has been played by Roger Delgado, Anthony Ainley, Eric Roberts?'', outperforming an encoder-generator Transformer model with 10x the parameters on this task. According to the Lama knowledge probes, EaE contains more factual knowledge than a similar sized Bert, as well as previous approaches that integrate external sources of entity knowledge.Because EaE associates parameters with specific entities, it only needs to access a fraction of its parameters at inference time, and we show that the correct identification and representation of entities is essential to EaE's performance.
NOTE: Video may display a random order of authors.
Correct author list is at the top of this page.
Connected Papers in EMNLP2020
Similar Papers
Learning from Context or Names? An Empirical Study on Neural Relation Extraction
Hao Peng, Tianyu Gao, Xu Han, Yankai Lin, Peng Li, Zhiyuan Liu, Maosong Sun, Jie Zhou,
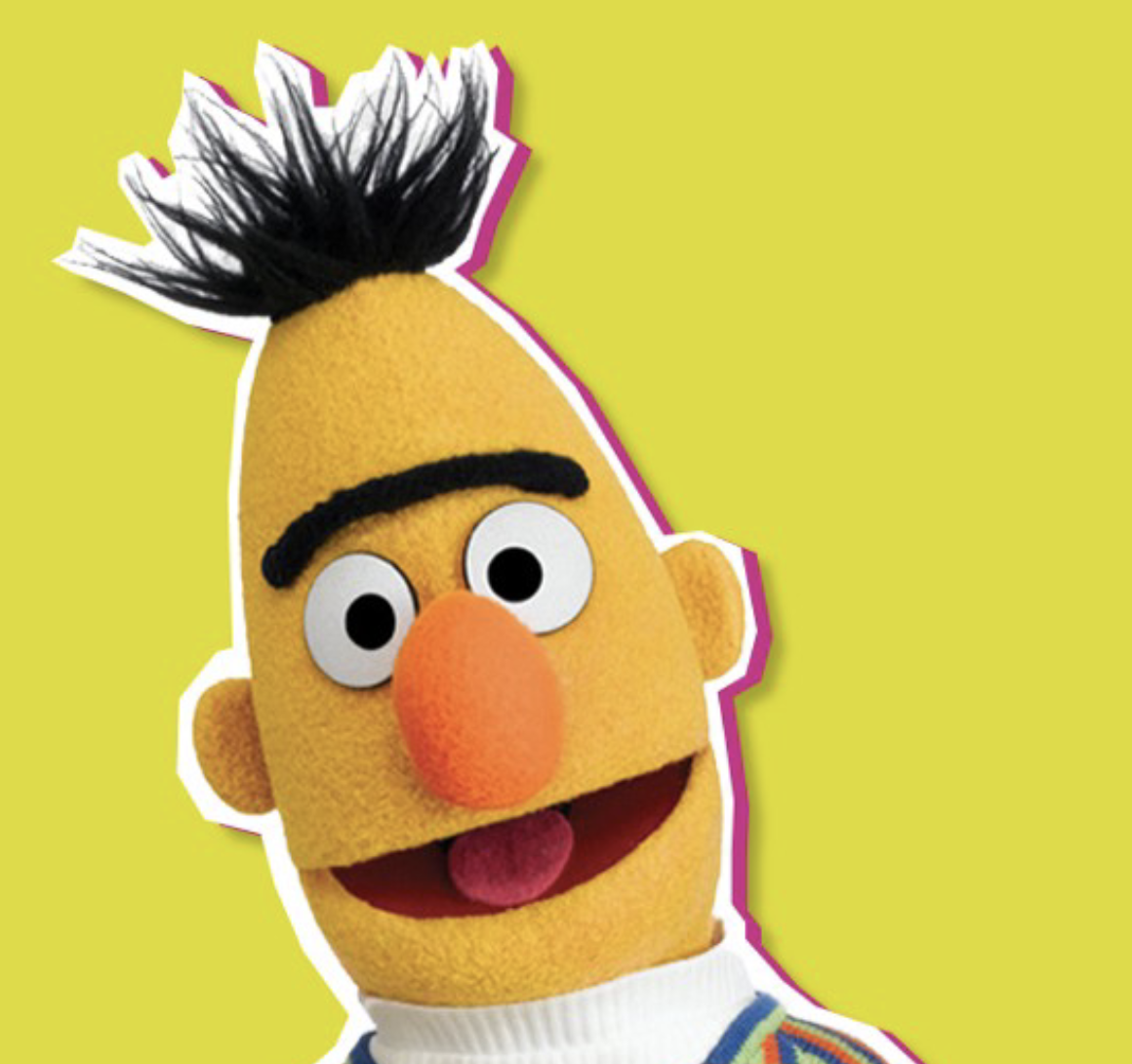
Exploiting Structured Knowledge in Text via Graph-Guided Representation Learning
Tao Shen, Yi Mao, Pengcheng He, Guodong Long, Adam Trischler, Weizhu Chen,

Understanding Procedural Text using Interactive Entity Networks
Jizhi Tang, Yansong Feng, Dongyan Zhao,
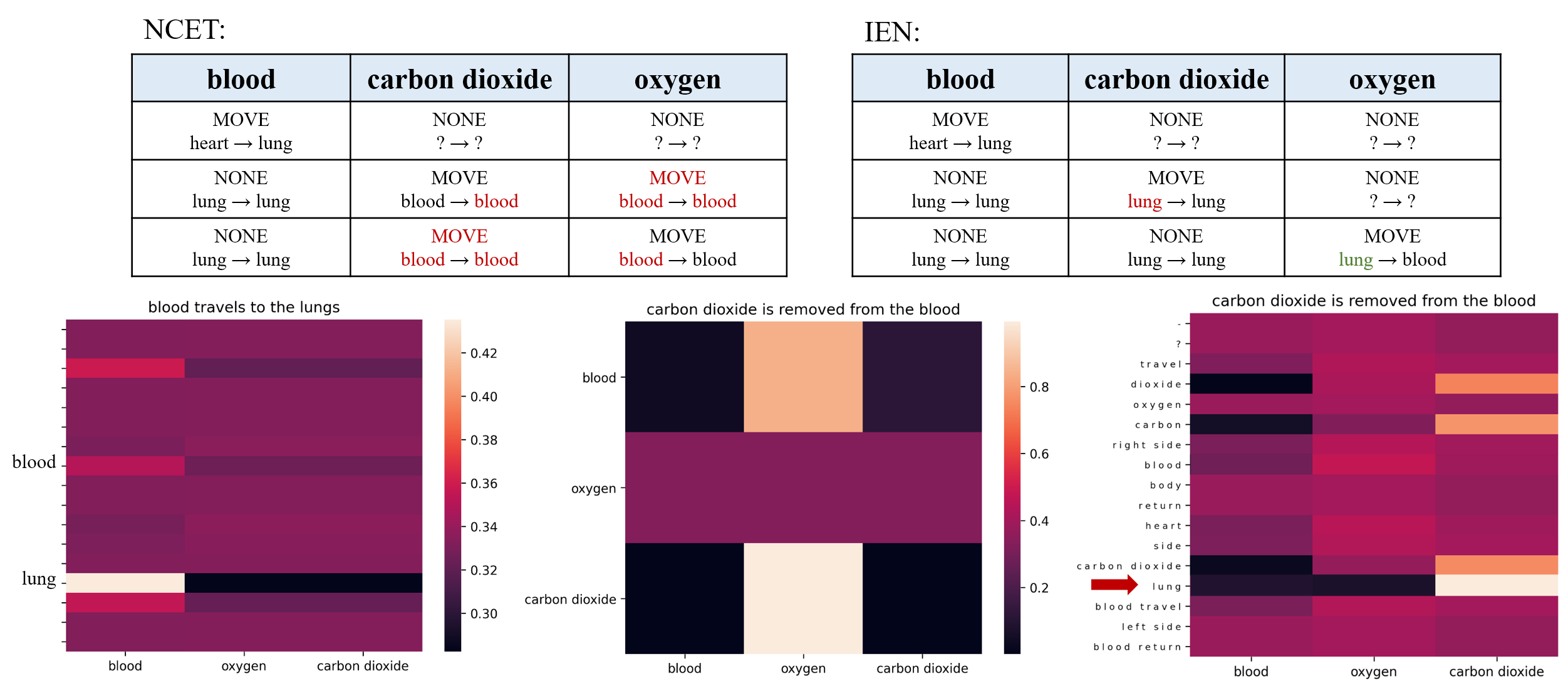
X-FACTR: Multilingual Factual Knowledge Retrieval from Pretrained Language Models
Zhengbao Jiang, Antonios Anastasopoulos, Jun Araki, Haibo Ding, Graham Neubig,
