Sequential Modelling of the Evolution of Word Representations for Semantic Change Detection
Adam Tsakalidis, Maria Liakata
Semantics: Lexical Semantics Long Paper
You can open the pre-recorded video in a separate window.
Abstract:
Semantic change detection concerns the task of identifying words whose meaning has changed over time. Current state-of-the-art approaches operating on neural embeddings detect the level of semantic change in a word by comparing its vector representation in two distinct time periods, without considering its evolution through time. In this work, we propose three variants of sequential models for detecting semantically shifted words, effectively accounting for the changes in the word representations over time. Through extensive experimentation under various settings with synthetic and real data we showcase the importance of sequential modelling of word vectors through time for semantic change detection. Finally, we compare different approaches in a quantitative manner, demonstrating that temporal modelling of word representations yields a clear-cut advantage in performance.
NOTE: Video may display a random order of authors.
Correct author list is at the top of this page.
Connected Papers in EMNLP2020
Similar Papers
Methods for Numeracy-Preserving Word Embeddings
Dhanasekar Sundararaman, Shijing Si, Vivek Subramanian, Guoyin Wang, Devamanyu Hazarika, Lawrence Carin,
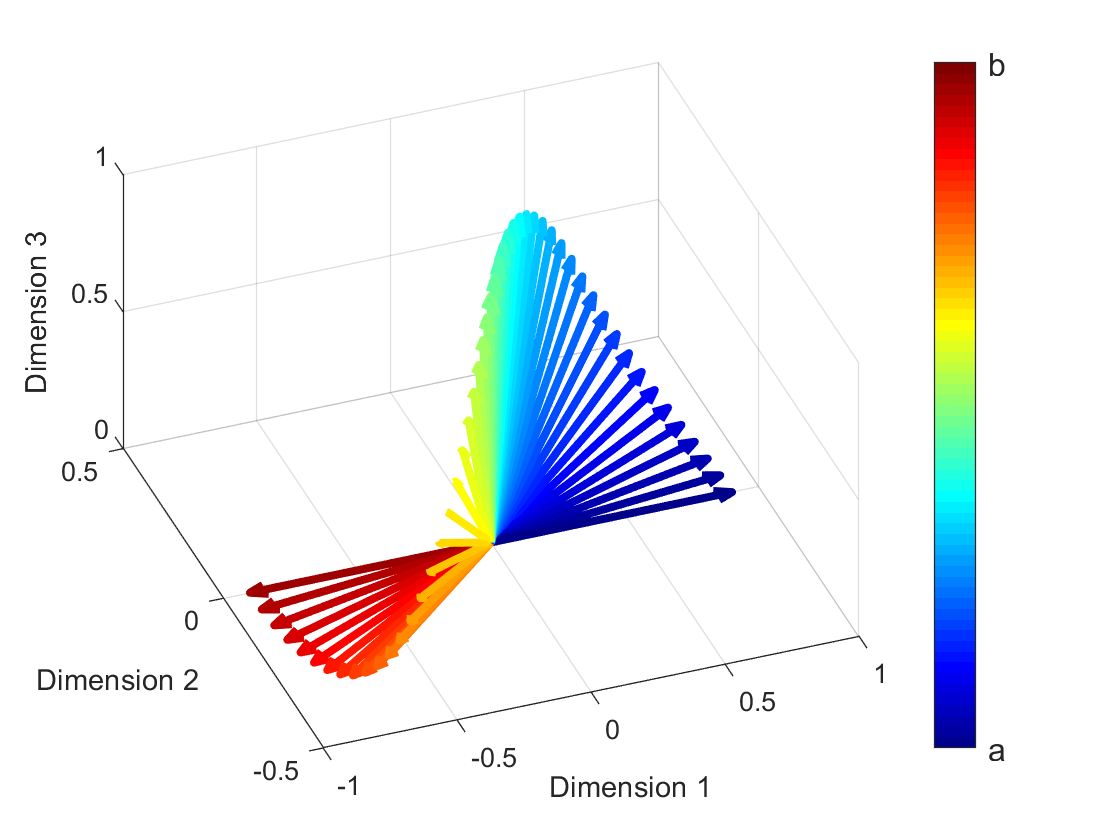
Don't Neglect the Obvious: On the Role of Unambiguous Words in Word Sense Disambiguation
Daniel Loureiro, Jose Camacho-Collados,
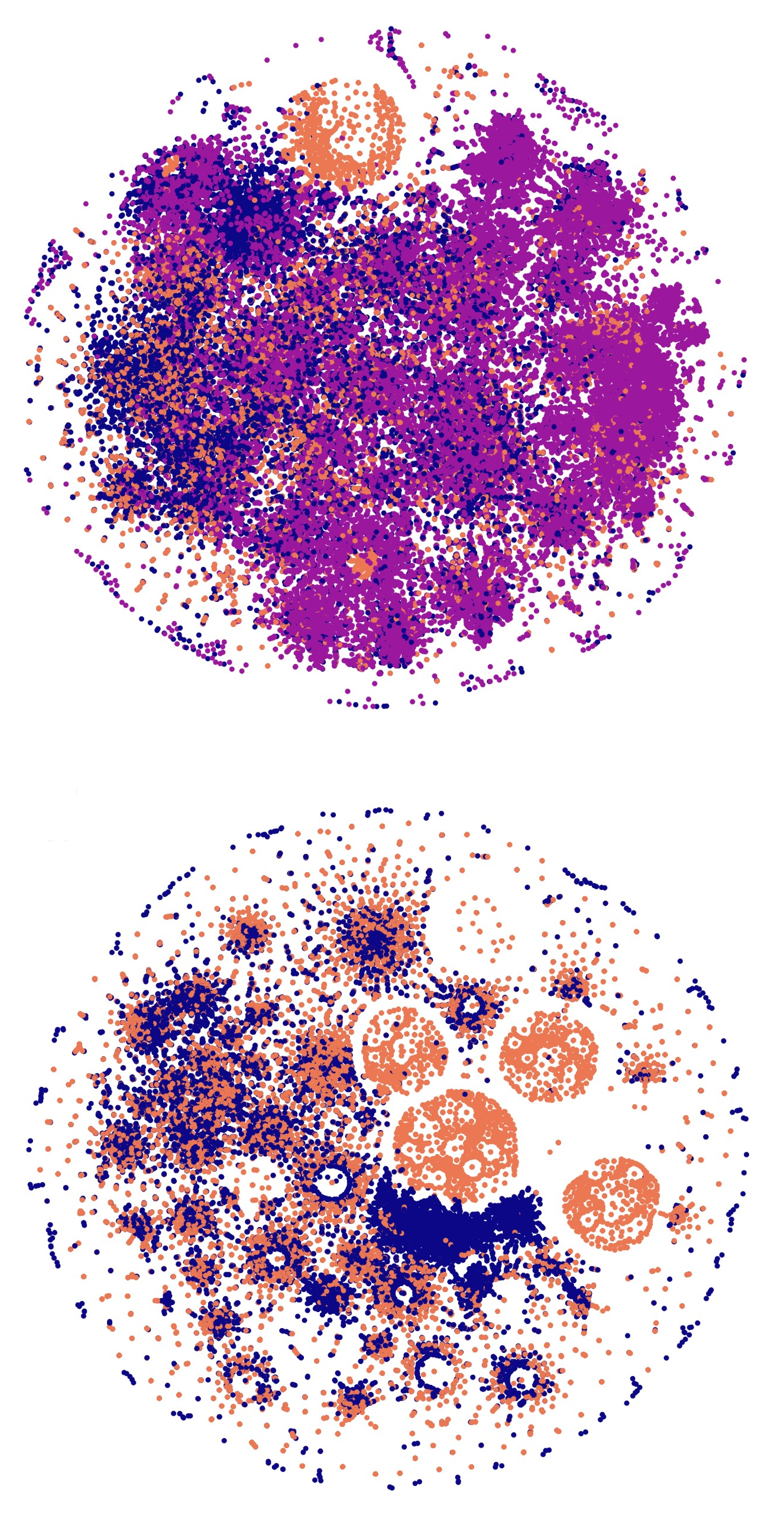