Predicting In-game Actions from Interviews of NBA Players
Nadav Oved, Amir Feder, Roi Reichart
NLP Applications Cl Paper
You can open the pre-recorded video in a separate window.
Abstract:
Sports competitions are widely researched in computer and social science, with the goal of understanding how players act under uncertainty. Although there is an abundance of computational work on player metrics prediction based on past performance, very few attempts to incorporate out-of-game signals have been made. Specifically, it was previously unclear whether linguistic signals gathered from players’ interviews can add information that does not appear in performance metrics. To bridge that gap, we define text classification tasks of predicting deviations from mean in NBA players’ in-game actions, which are associated with strategic choices, player behavior, and risk, using their choice of language prior to the game. We collected a data set of transcripts from key NBA players’ pre-game interviews and their in-game performance metrics, totalling 5,226 interview-metric pairs. We design neural models for players’ action prediction based on increasingly more complex aspects of the language signals in their openended interviews. Our models can make their predictions based on the textual signal alone, or on a combination of that signal with signals from past-performance metrics. Our text-based models outperform strong baselines trained on performance metrics only, demonstrating the importance of language usage for action prediction. Moreover, the models that utilize both textual input and past-performance metrics produced the best results. Finally, as neural networks are notoriously difficult to interpret, we propose a method for gaining further insight into what our models have learned. Particularly, we present a latent Dirichlet allocation–based analysis, where we interpretmodel predictions in terms of correlated topics. We find that our best performing textual modelis most associated with topics that are intuitively related to each prediction task and that bettermodels yield higher correlation with more informative topics.
NOTE: Video may display a random order of authors.
Correct author list is at the top of this page.
Connected Papers in EMNLP2020
Similar Papers
Interactive Fiction Game Playing as Multi-Paragraph Reading Comprehension with Reinforcement Learning
Xiaoxiao Guo, Mo Yu, Yupeng Gao, Chuang Gan, Murray Campbell, Shiyu Chang,
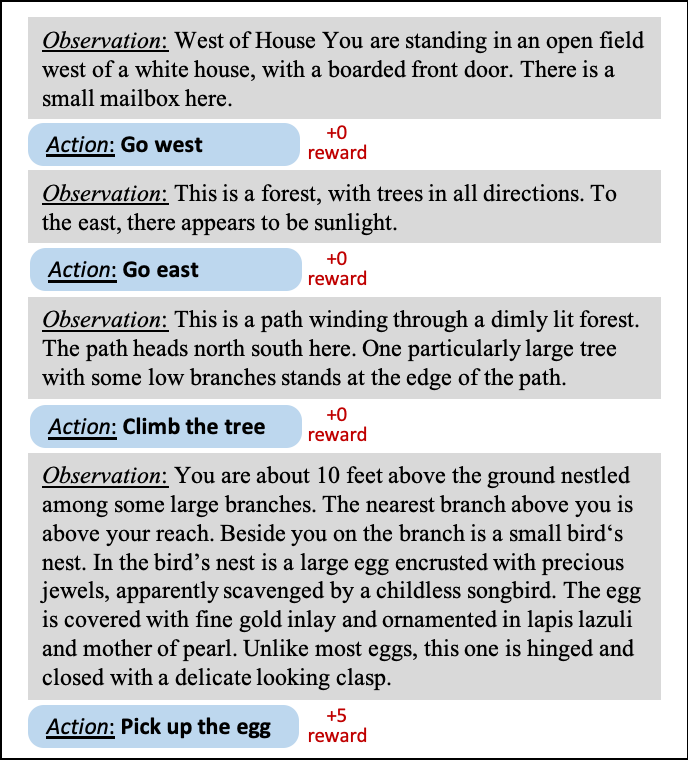
Information Seeking in the Spirit of Learning: A Dataset for Conversational Curiosity
Pedro Rodriguez, Paul Crook, Seungwhan Moon, Zhiguang Wang,
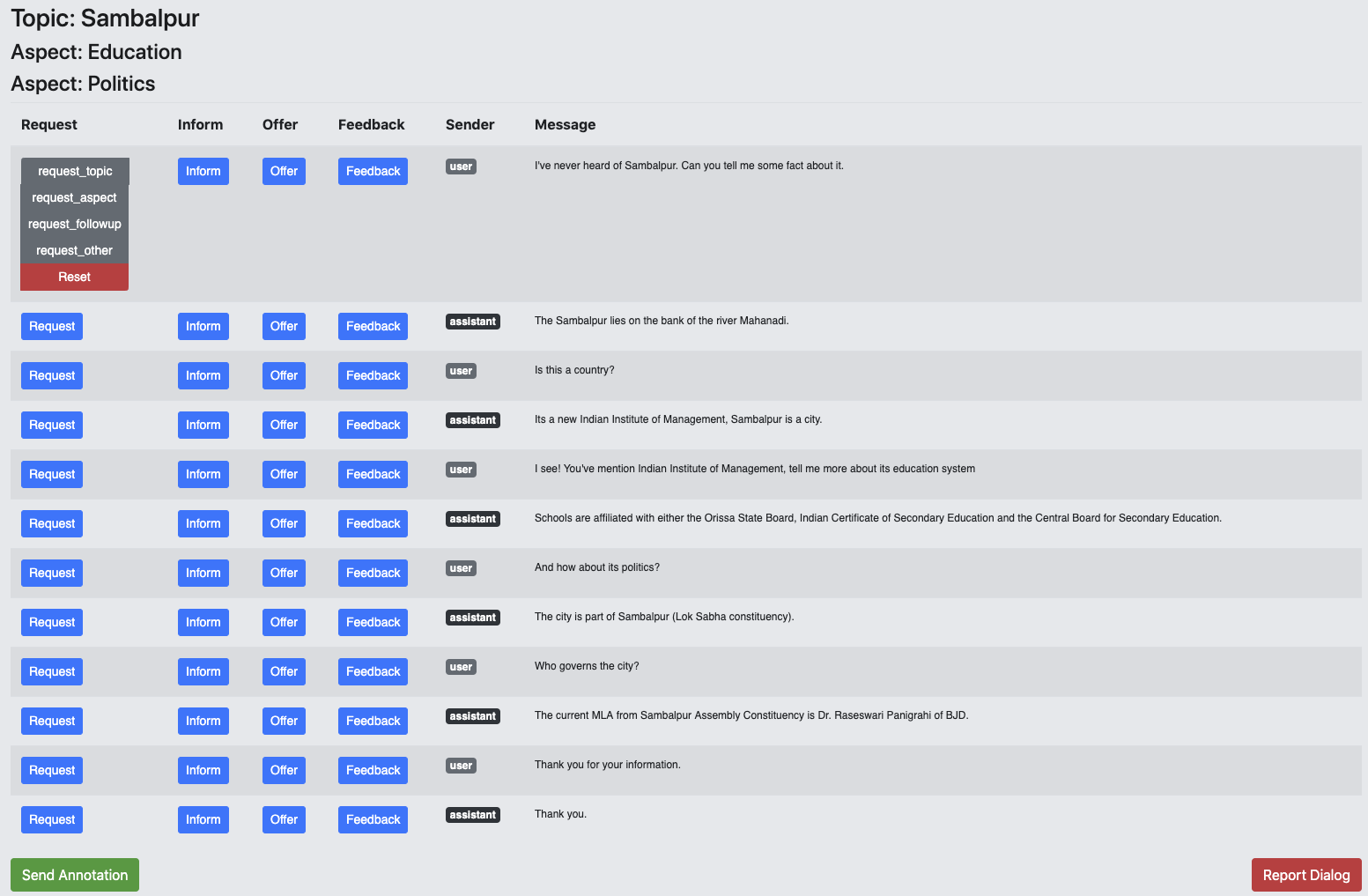
Human-centric dialog training via offline reinforcement learning
Natasha Jaques, Judy Hanwen Shen, Asma Ghandeharioun, Craig Ferguson, Agata Lapedriza, Noah Jones, Shixiang Gu, Rosalind Picard,
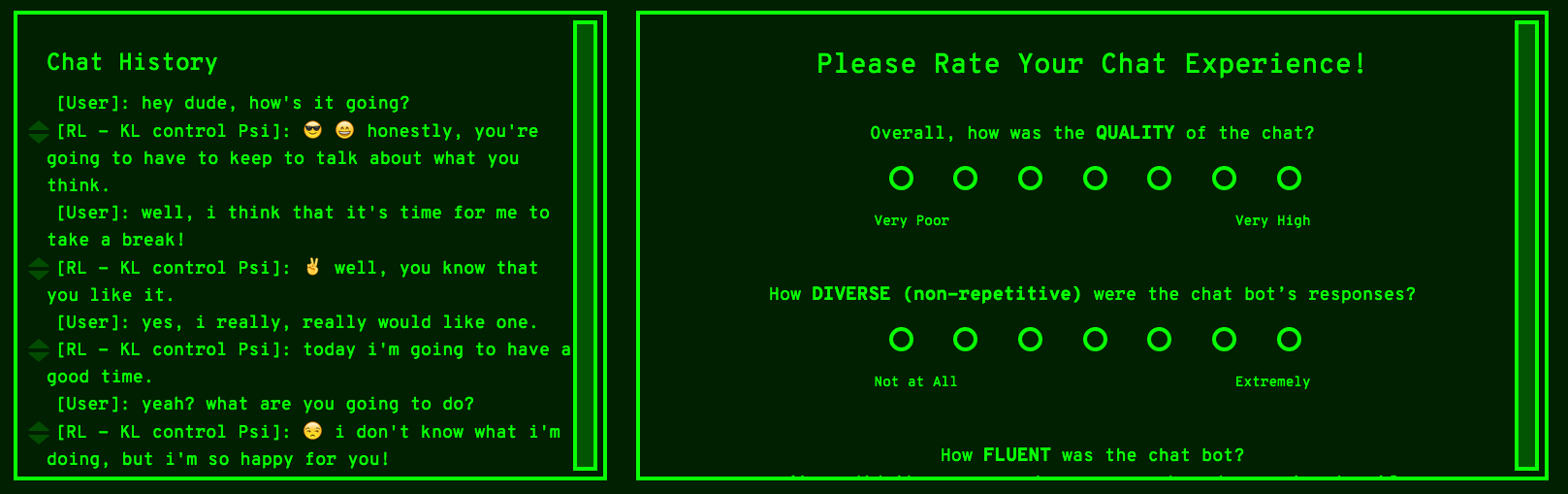
Joint Estimation and Analysis of Risk Behavior Ratings in Movie Scripts
Victor Martinez, Krishna Somandepalli, Yalda Tehranian-Uhls, Shrikanth Narayanan,
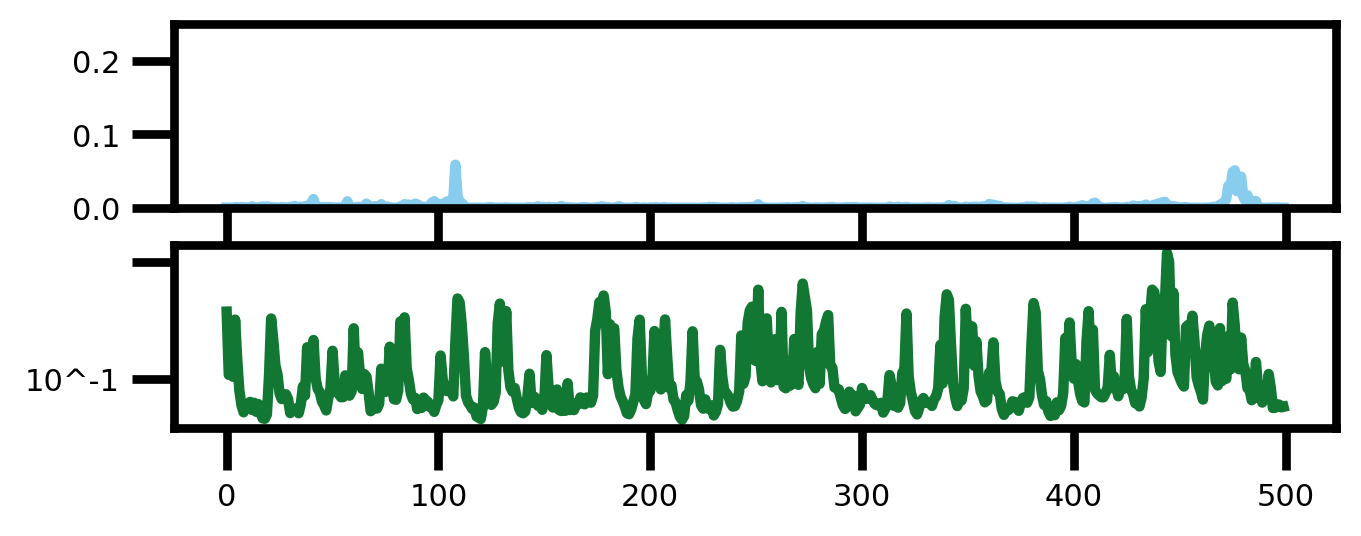